Adaptive Statistical Detection Of False Data Injection Attacks In Smart Grids
2016 IEEE GLOBAL CONFERENCE ON SIGNAL AND INFORMATION PROCESSING (GLOBALSIP)(2016)
摘要
The smart power grid is a synergistic system that integrates diverse network components for power generation, transmission, and distribution. Its advanced metering infrastructure (AMI) enables the grid's efficient and reliable operation. Nevertheless, it is amenable to advanced cyber threats; malicious actors can compromise vulnerable meters and arbitrarily alter their readings. These orchestrated "false data injection attacks" can lead to power outages and service interruption. We propose a framework that uses measurements from trusted (secure) nodes in order to detect abnormal "spoofing" activity of other nodes, possibly tampered. Our model considers the structural similarities in the electricity consumption of AMI nodes, and exploits the spatial correlation amongst meters. To alleviate the problem's large-dimensionality aspect, the meters are clustered into classes of similar energy patterns. We evaluate our algorithms using real-world building data obtained from a large university campus.
更多查看译文
关键词
Network kriging, anomaly detection, false data injection attacks, AMI power data
AI 理解论文
溯源树
样例
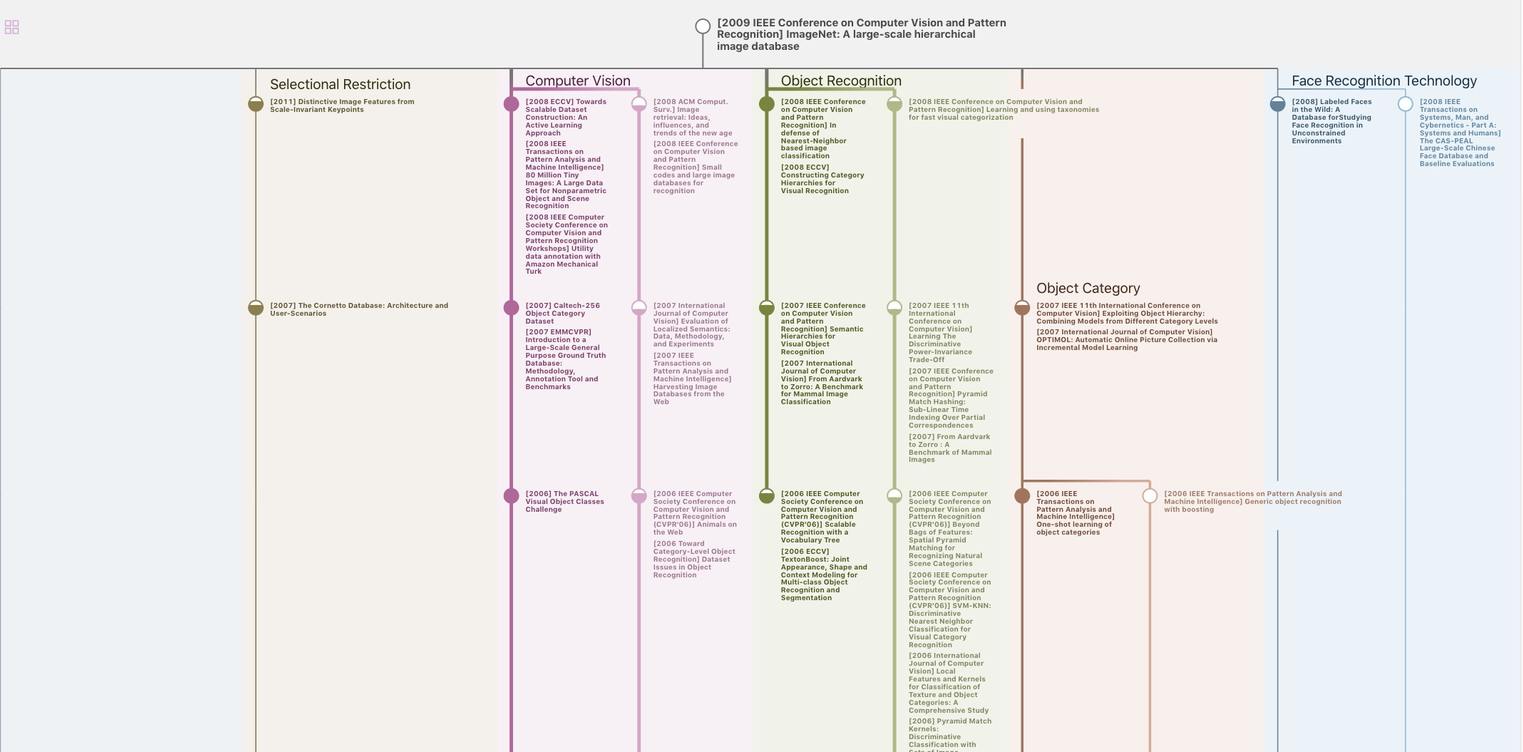
生成溯源树,研究论文发展脉络
Chat Paper
正在生成论文摘要