Construct, Merge, Solve and Adapt Versus Large Neighborhood Search for Solving the Multi-dimensional Knapsack Problem: Which One Works Better When?
Lecture Notes in Computer Science(2017)
摘要
Both, Construct, Merge, Solve and Adapt (CMSA) and Large Neighborhood Search (LNS), are hybrid algorithms that are based on iteratively solving sub-instances of the original problem instances, if possible, to optimality. This is done by reducing the search space of the tackled problem instance in algorithm-specific ways which differ from one technique to the other. In this paper we provide first experimental evidence for the intuition that, conditioned by the way in which the search space is reduced, LNS should generally work better than CMSA in the context of problems in which solutions are rather large, and the opposite is the case for problems in which solutions are rather small. The size of a solution is hereby measured by the number of components of which the solution is composed, in comparison to the total number of solution components. Experiments are conducted in the context of the multi-dimensional knapsack problem.
更多查看译文
AI 理解论文
溯源树
样例
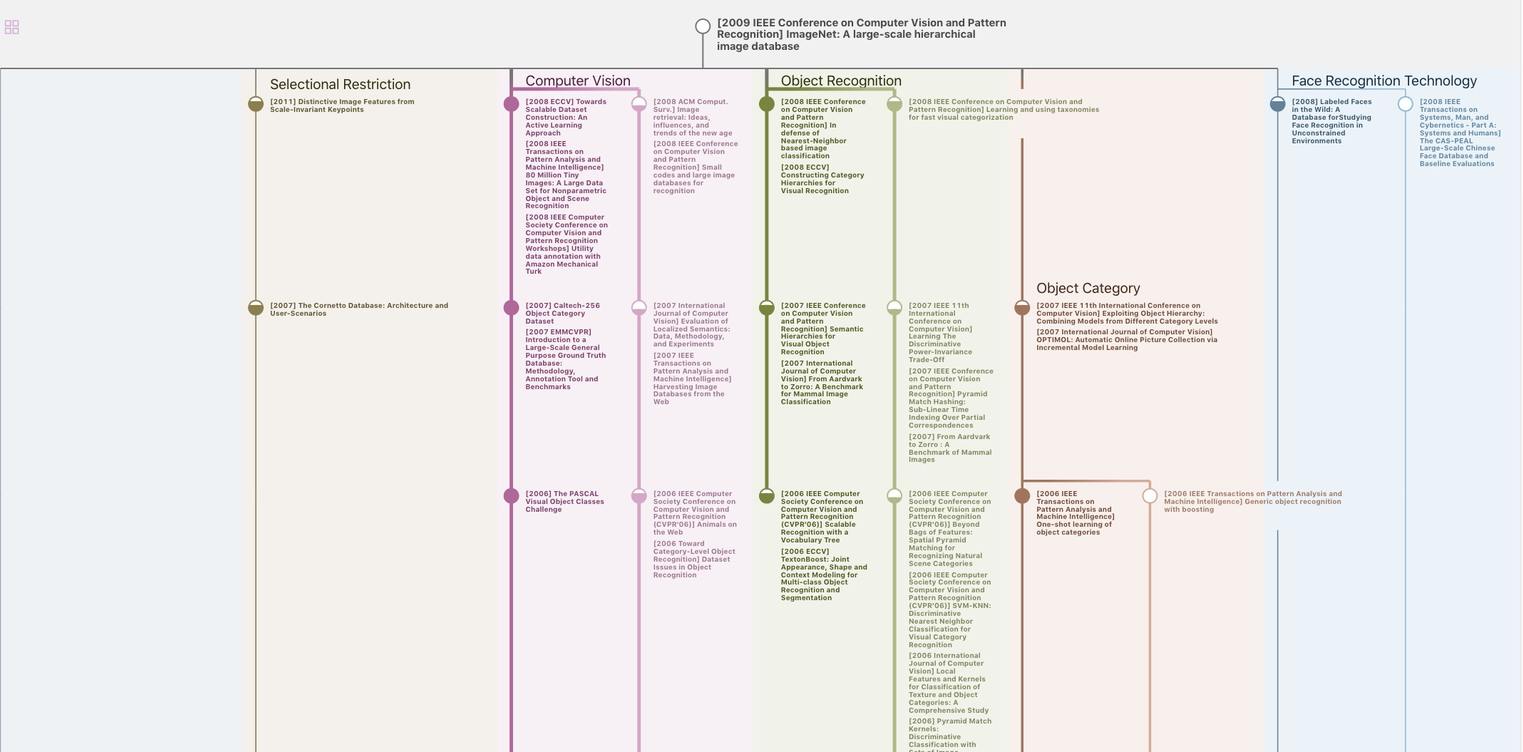
生成溯源树,研究论文发展脉络
Chat Paper
正在生成论文摘要