Coupling novelty and surprise for evolutionary divergence.
GECCO(2017)
摘要
Divergent search techniques applied to evolutionary computation, such as novelty search and surprise search, have demonstrated their efficacy in highly deceptive problems compared to traditional objective-based fitness evolutionary processes. While novelty search rewards unseen solutions, surprise search rewards unexpected solutions. As a result these two algorithms perform a different form of search since an expected solution can be novel while an already seen solution can be surprising. As novelty and surprise search have already shown much promise individually, the hypothesis is that an evolutionary process that rewards both novel and surprising solutions will be able to handle deception in a better fashion and lead to more successful solutions faster. In this paper we introduce an algorithm that realises both novelty and surprise search and we compare it against the two algorithms that compose it in a number of robot navigation tasks. The key findings of this paper suggest that coupling novelty and surprise is advantageous compared to each search approach on its own. The introduced algorithm breaks new ground in divergent search as it outperforms both novelty and surprise in terms of efficiency and robustness, and it explores the behavioural space more extensively.
更多查看译文
关键词
Surprise search, novelty search, divergent search, deception, fitness-based evolution, map navigation, NEAT
AI 理解论文
溯源树
样例
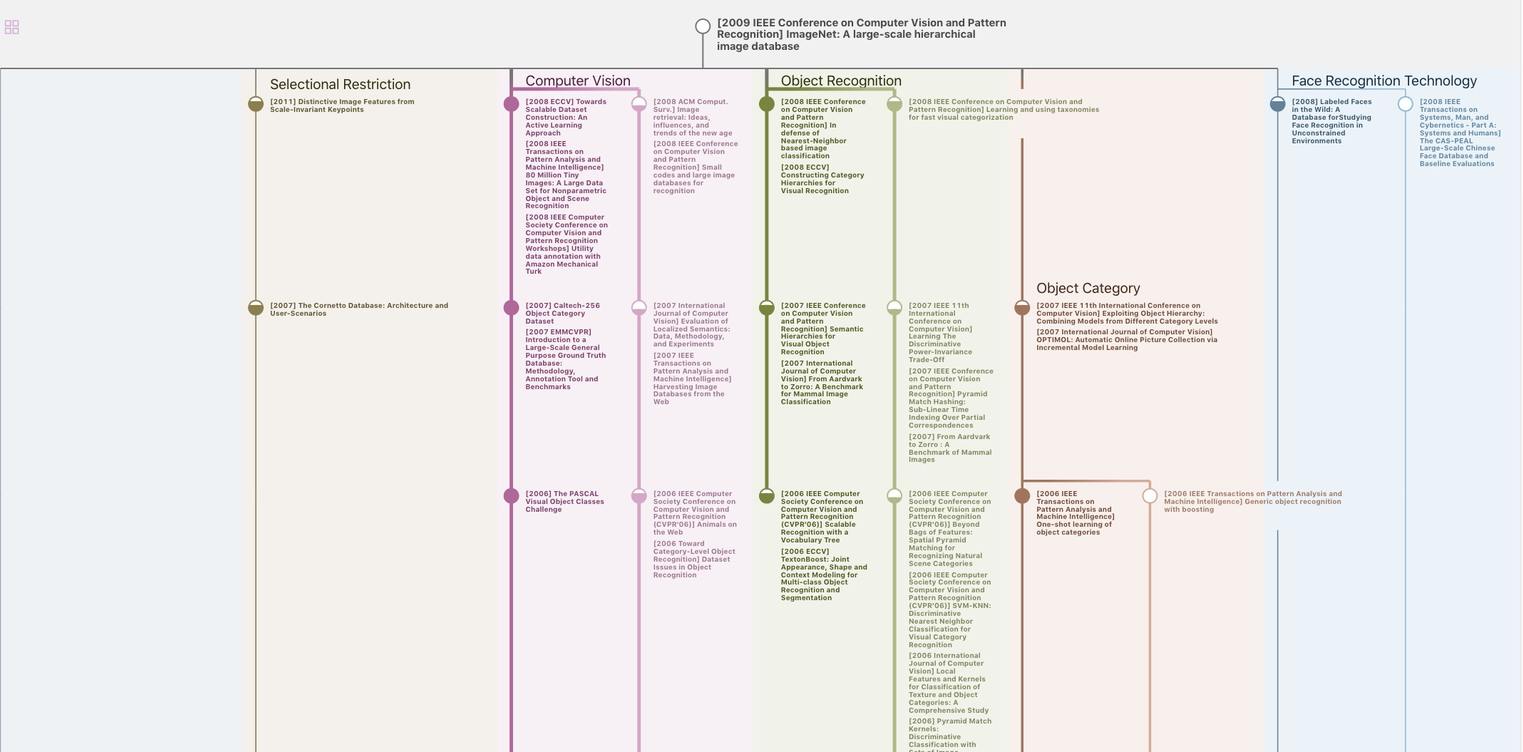
生成溯源树,研究论文发展脉络
Chat Paper
正在生成论文摘要