Partial Multi-View Clustering Using Graph Regularized Nmf
2016 23RD INTERNATIONAL CONFERENCE ON PATTERN RECOGNITION (ICPR)(2016)
摘要
Real-world datasets consist of data representations (views) from different sources which often provide information complementary to each other. Multi-view learning algorithms aim at exploiting the complementary information present in different views for clustering and classification tasks. Several multi-view clustering methods that aim at partitioning objects into clusters based on multiple representations of the object have been proposed. Almost all of the proposed methods assume that each example appears in all views or at least there is one view containing all examples. In real-world settings this assumption might be too restrictive. Recent work on Partial View Clustering addresses this limitation by proposing a Non-negative Matrix Factorization based approach called PVC. Our work extends the PVC work in two directions. First, the current PVC algorithm is designed specifically for two-view datasets. We extend this algorithm for the k partial-view scenario. Second, we extend our k partial-view algorithm to include view specific graph laplacian regularization. This enables the proposed algorithm to exploit the intrinsic geometry of the data distribution in each view. The proposed method, which is referred to as GPMVC (Graph Regularized Partial Multi-View Clustering), is compared against 7 baseline methods (including PVC) on 5 publicly available text and image datasets. In all settings the proposed GPMVC method outperforms all baselines. For the purpose of reproducibility, we provide access to our code.
更多查看译文
关键词
graph regularized NMF,data representations,multiview learning algorithms,classification,nonnegative matrix factorization,PVC algorithm,k partial-view algorithm,view specific graph Laplacian regularization,data distribution,intrinsic geometry,GPMVC,graph regularized partial multiview clustering,text datasets,image datasets
AI 理解论文
溯源树
样例
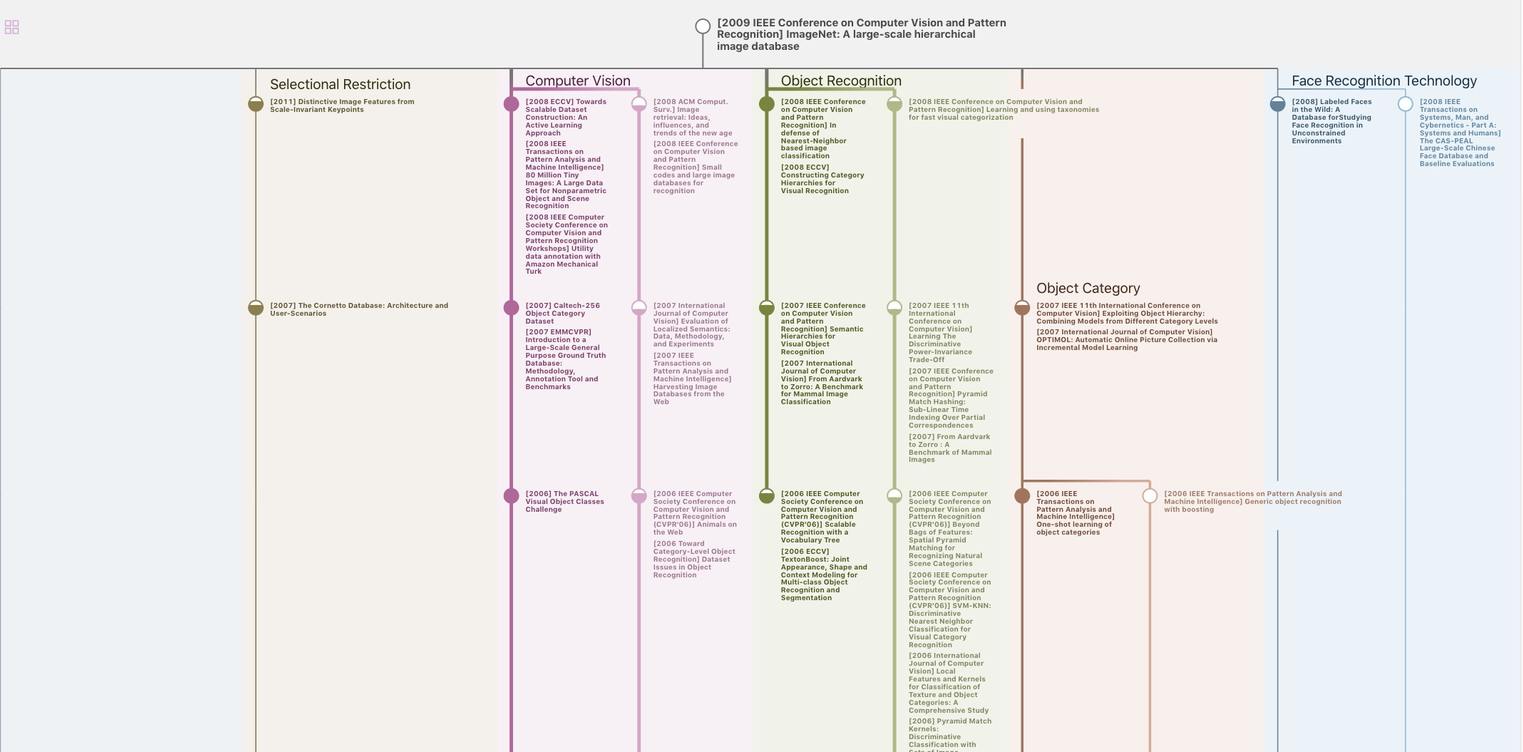
生成溯源树,研究论文发展脉络
Chat Paper
正在生成论文摘要