D-Lsm: Deep Liquid State Machine With Unsupervised Recurrent Reservoir Tuning
2016 23RD INTERNATIONAL CONFERENCE ON PATTERN RECOGNITION (ICPR)(2016)
摘要
The Liquid State Machine (LSM) is a biologically plausible model of computation for recurrent spiking neural networks, which offers promising solutions to real-world applications in both software and hardware based systems. At the same time, deep feedforward rate-based neural networks such as convolutional neural networks (CNNs) have achieved great success in many computer vision related applications. However, a systematic exploration of deep recurrent spiking neural networks is lacking. We propose a new model of Deep Liquid State Machine (D-LSM), which simultaneously explores the powers of recurrent spiking networks and deep architectures. D-LSM consists of multiple basic LSM processing and pooling stages. Recurrent reservoir networks across different LSM stages act as nonlinear filters capable of extracting spatio-temporal features of increasingly higher levels from the input. We propose to train the D-LSM practically by adopting unsupervised training (e.g. through STDP) for recurrent reservoirs and spike-based supervised rules for the final readout stage. The perspective of realizing D-LSM based hardware processors is also presented.
更多查看译文
关键词
D-LSM,deep liquid state machine,unsupervised recurrent reservoir tuning,deep feedforward rate-based neural networks,convolutional neural networks,CNN,deep recurrent spiking neural networks,deep architectures,LSM processing,LSM pooling,recurrent reservoir networks,nonlinear filters,spatio-temporal feature extraction,unsupervised training,spike-based supervised rules,hardware processors
AI 理解论文
溯源树
样例
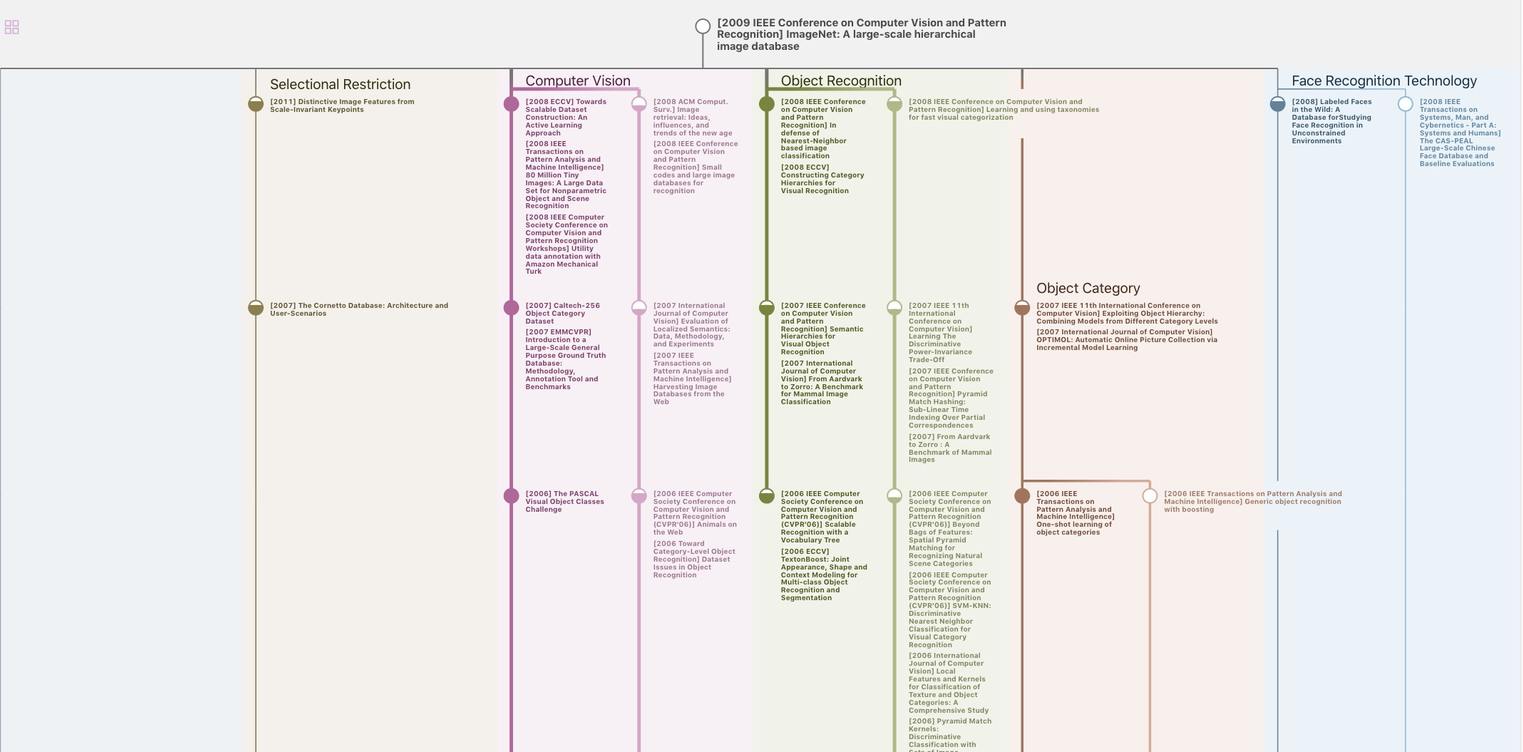
生成溯源树,研究论文发展脉络
Chat Paper
正在生成论文摘要