Supervised Group Nonnegative Matrix Factorisation With Similarity Constraints And Applications To Speaker Identification
2017 IEEE INTERNATIONAL CONFERENCE ON ACOUSTICS, SPEECH AND SIGNAL PROCESSING (ICASSP)(2017)
摘要
This paper presents supervised feature learning approaches for speaker identification that rely on nonnegative matrix factorisation. Recent studies have shown that group nonnegative matrix factorisation and task-driven supervised dictionary learning can help performing effective feature learning for audio classification problems.This paper proposes to integrate a recent method that relies on group nonnegative matrix factorisation into a task-driven supervised framework for speaker identification. The goal is to capture both the speaker variability and the session variability while exploiting the discriminative learning aspect of the task-driven approach. Results on a subset of the ESTER corpus prove that the proposed approach can be competitive with I-vectors.
更多查看译文
关键词
Nonnegative matrix factorisation, feature learning, dictionary learning, online learning, speaker identification
AI 理解论文
溯源树
样例
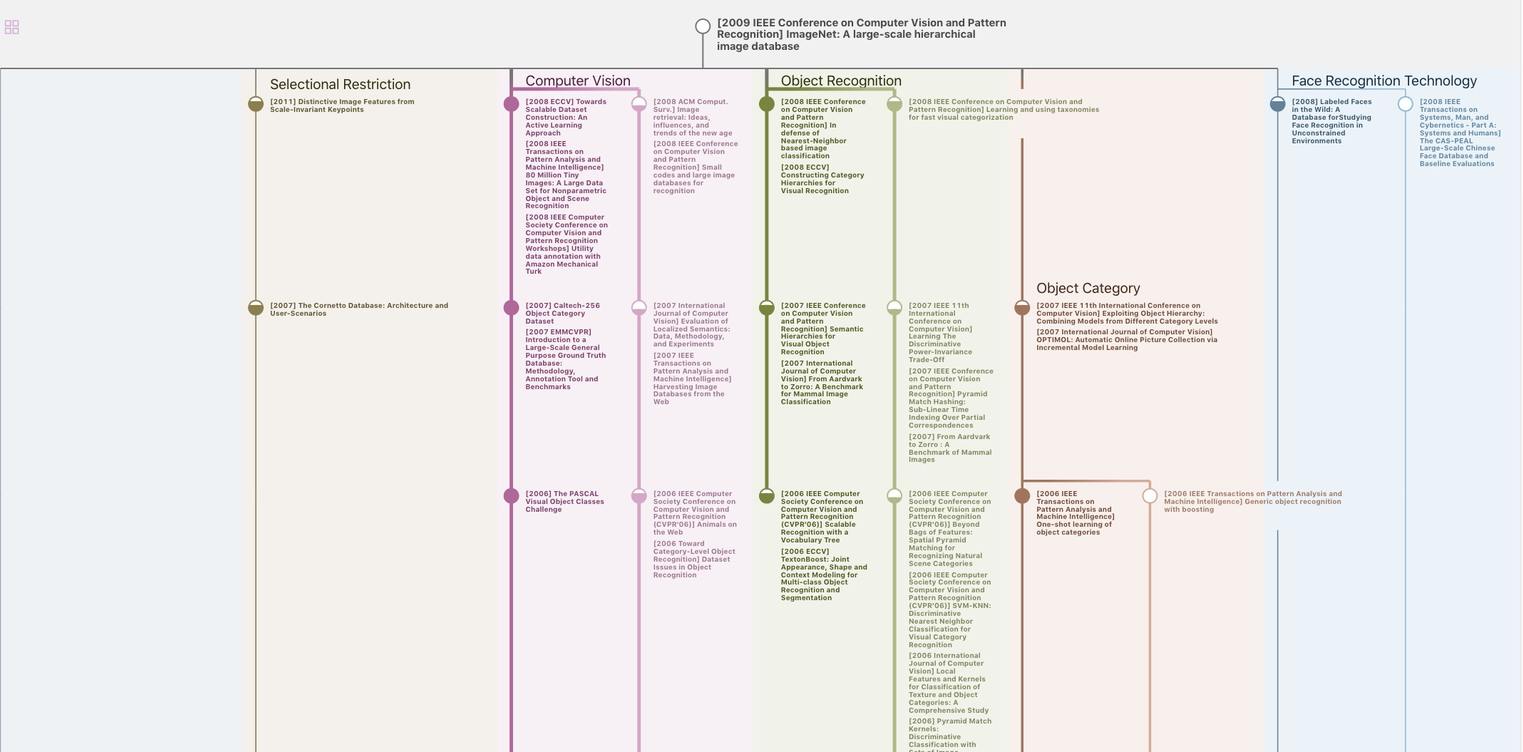
生成溯源树,研究论文发展脉络
Chat Paper
正在生成论文摘要