Pre-Processing And Classification Of Hyperspectral Imagery Via Selective Inpainting
2017 IEEE INTERNATIONAL CONFERENCE ON ACOUSTICS, SPEECH AND SIGNAL PROCESSING (ICASSP)(2017)
Abstract
We propose a semi-supervised algorithm for processing and classification of hyperspectral imagery. For initialization, we keep 20% of the data intact, and use Principal Component Analysis to discard voxels from noisier bands and pixels. Then, we use either an Accelerated Proximal Gradient algorithm (APGL), or a modified APGL algorithm with a penalty term for distance between inpainted pixels and endmembers (APGL_Hyp), on the initialized datacube to inpaint the missing data. APGL and APGL_Hyp are distinguished by performance on datasets with full pixels removed or extreme noise. This inpainting technique results in band-by-band datacube sharpening and removal of noise from individual spectral signatures. We can also classify the inpainted cube by assigning each pixel to its nearest endmember via Euclidean distance. We demonstrate improved accuracy in classification over data-mining techniques like k-means, unmixing techniques like Hierarchical Non-Negative Matrix Factorization, and graph-based methods like Non-Local Total Variation.
MoreTranslated text
Key words
Hyperspectral imagery (HSI), data preprocessing, image inpainting, image classification, image enhancement
AI Read Science
Must-Reading Tree
Example
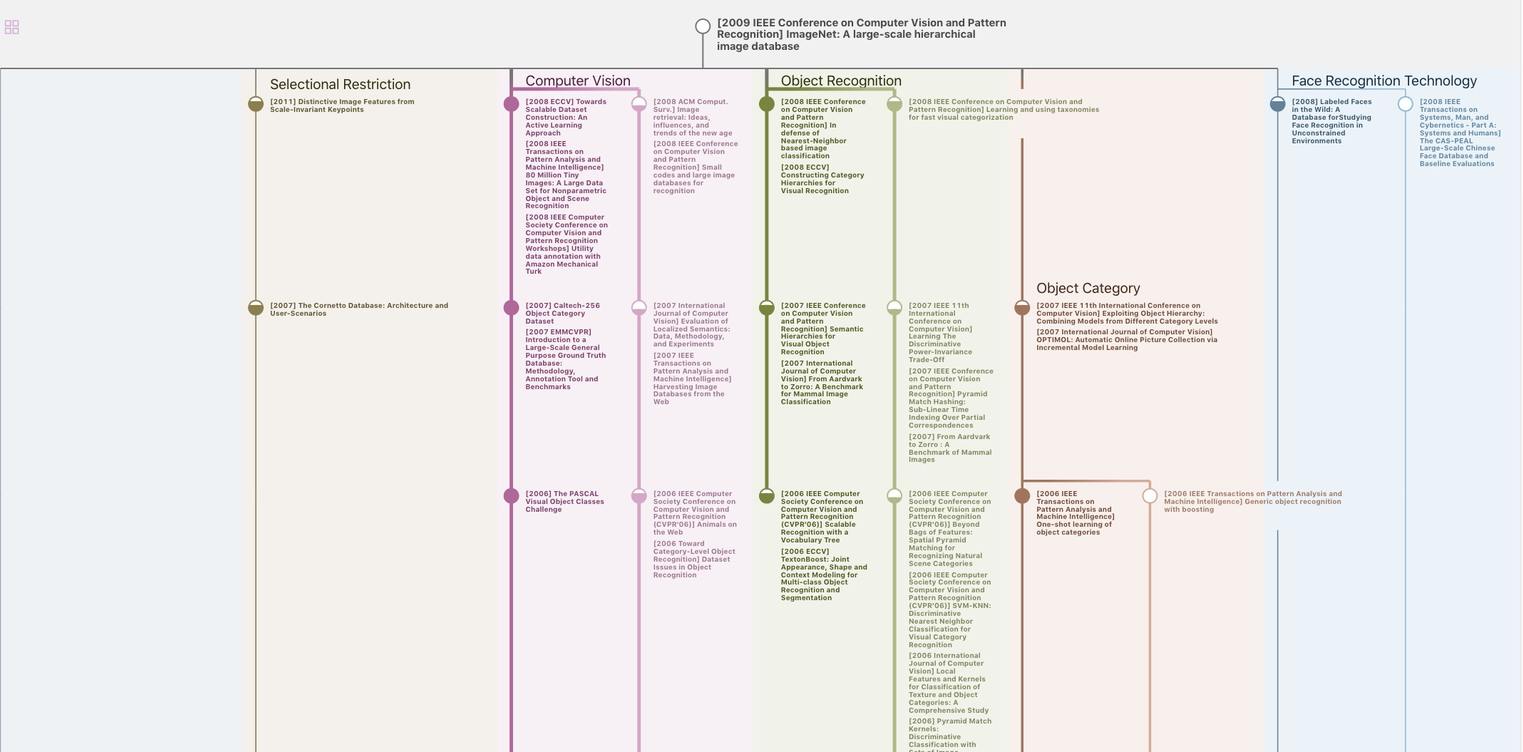
Generate MRT to find the research sequence of this paper
Chat Paper
Summary is being generated by the instructions you defined