Guided Deep Network For Depth Map Super-Resolution: How Much Can Color Help?
2017 IEEE INTERNATIONAL CONFERENCE ON ACOUSTICS, SPEECH AND SIGNAL PROCESSING (ICASSP)(2017)
Abstract
Since the quality of depth maps produced by Time-of-Flight (TOF) cameras is low, color-guided recovery methods have been proposed to increase spatial resolution and suppress unwanted noise. Despite successful applications of deep neural networks in color image super-resolution (SR), their potential for depth map SR is largely unknown. In this paper, we present a deep neural network architecture to leam the endto- end mapping between low-resolution and high-resolution depth maps. Furthermore, we introduce a novel color-guided deep Fully Convolutional Network (FCN) and propose to jointly leam two nonlinear mapping functions (color-to-depth and LR-to-HR) in the presence of noise. Experimental results on several benchmark data sets show that our method outperforms several existing state-of-the-art depth SR algorithms. Moreover, this work attempts to partially shed some light onto the fundamental question in color-guided depth recovery - how much can color help in depth SR?
MoreTranslated text
Key words
Depth map super-resolution, colorguided depth recovery, deep neural network
AI Read Science
Must-Reading Tree
Example
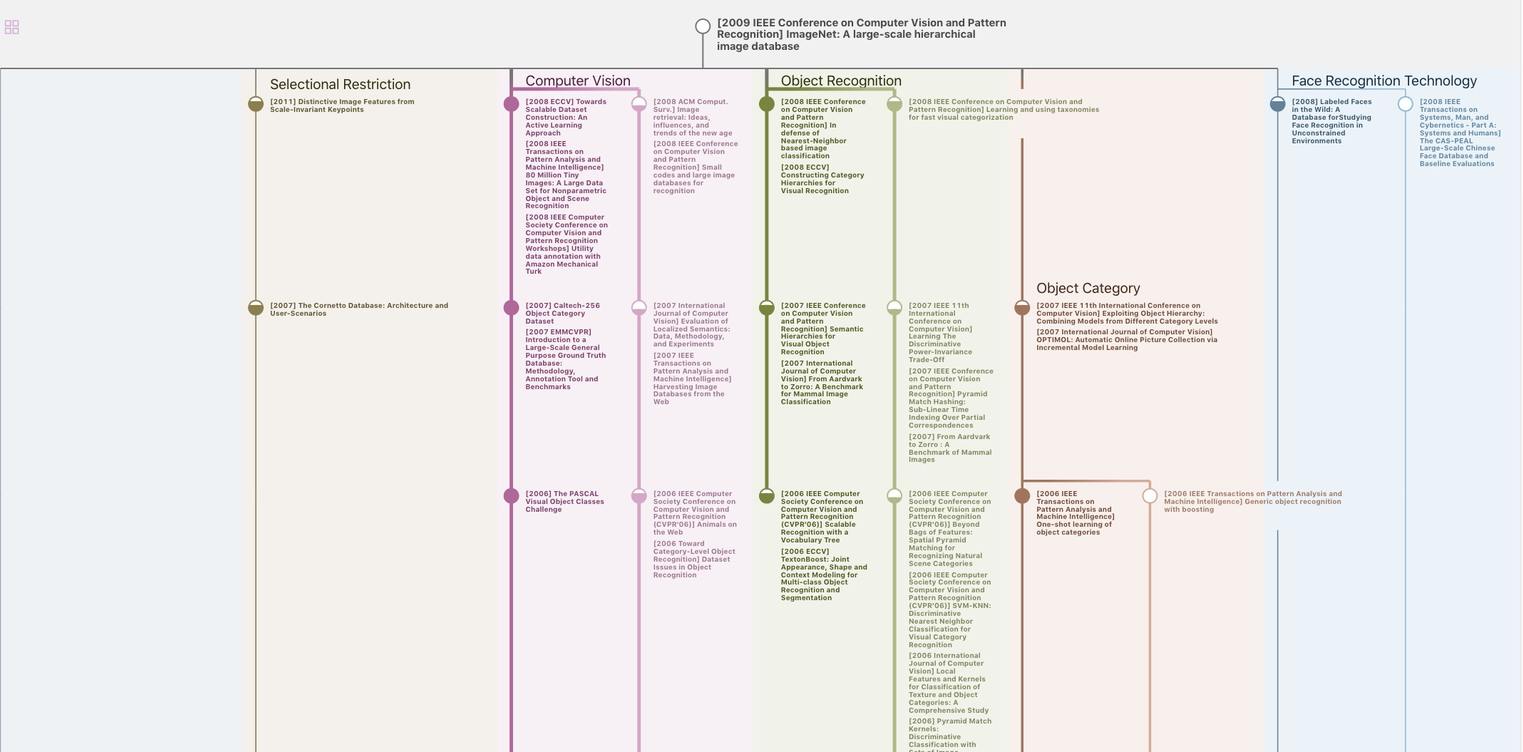
Generate MRT to find the research sequence of this paper
Chat Paper
Summary is being generated by the instructions you defined