Improving Audio-Visual Speech Recognition Using Deep Neural Networks With Dynamic Stream Reliability Estimates
2017 IEEE INTERNATIONAL CONFERENCE ON ACOUSTICS, SPEECH AND SIGNAL PROCESSING (ICASSP)(2017)
摘要
Audio-visual speech recognition is a promising approach to tackling the problem of reduced recognition rates under adverse acoustic conditions. However, finding an optimal mechanism for combining multi-modal information remains a challenging task. Various methods are applicable for integrating acoustic and visual information in Gaussian-mixture-model-based speech recognition, e.g., via dynamic stream weighting. The recent advances of deep neural network (DNN)-based speech recognition promise improved performance when using audio-visual information. However, the question of how to optimally integrate acoustic and visual information remains.In this paper, we propose a state-based integration scheme that uses dynamic stream weights in DNN-based audio-visual speech recognition. The dynamic weights are obtained from a time-variant reliability estimate that is derived from the audio signal. We show that this state-based integration is superior to early integration of multi-modal features, even if early integration also includes the proposed reliability estimate. Furthermore, the proposed adaptive mechanism is able to outperform a fixed weighting approach that exploits oracle knowledge of the true signal-to-noise ratio.
更多查看译文
关键词
audio-visual speech recognition, deep neural networks, feature fusion, dynamic stream weighting
AI 理解论文
溯源树
样例
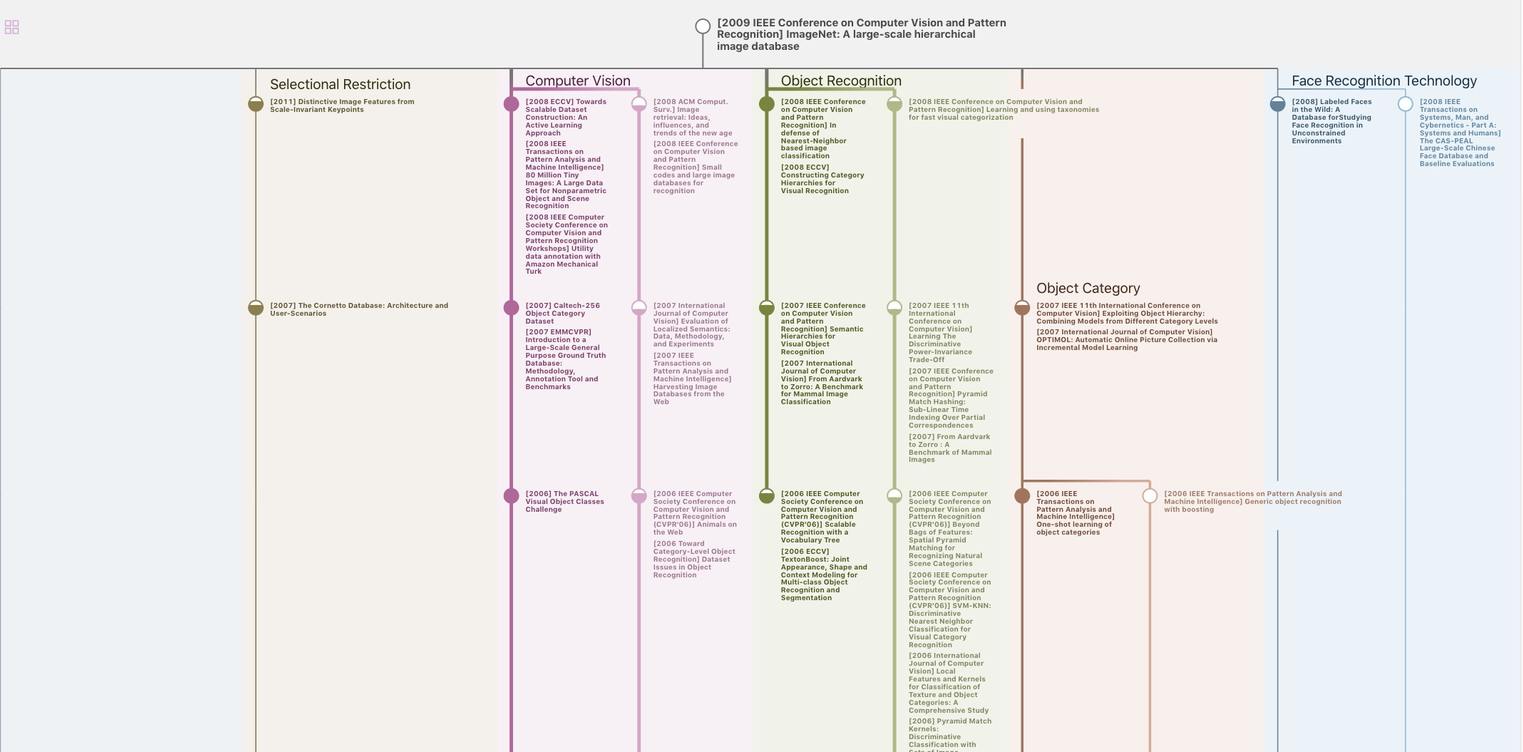
生成溯源树,研究论文发展脉络
Chat Paper
正在生成论文摘要