Bi-directional Joint Inference for User Links and Attributes on Large Social Graphs.
WWW (Companion Volume)(2017)
摘要
Users on social networks primarily do two things, connect to existing or new friends and exchange information. Recently, social media apps have become the primary information source for users to consume news stories. Users are inundated with a flood of information from social media networks shared by their friends and other content providers. For social media networks, it is important for us to study users' friendships and interests in order to best serve their need. However, given the nature of online applications, information on both sides is highly incomplete and noisy. Inspired by the well-known homophily phenomenon which found the two properties strongly interleaving, we propose to jointly learn them by leveraging their data redundancy and mutual reinforcement. Specifically, we exploit homophily by iteratively addressing smoothness on the graph in two directions, ie, from closeness to similarity (stronger links lead to more similar attributes), and vice versa. The two processes are done in a unified probabilistic framework through label propagation and graph construction. The refined user links and attributes are immediately useful for various tasks including link recommendation and content targeting on social networks.
更多查看译文
AI 理解论文
溯源树
样例
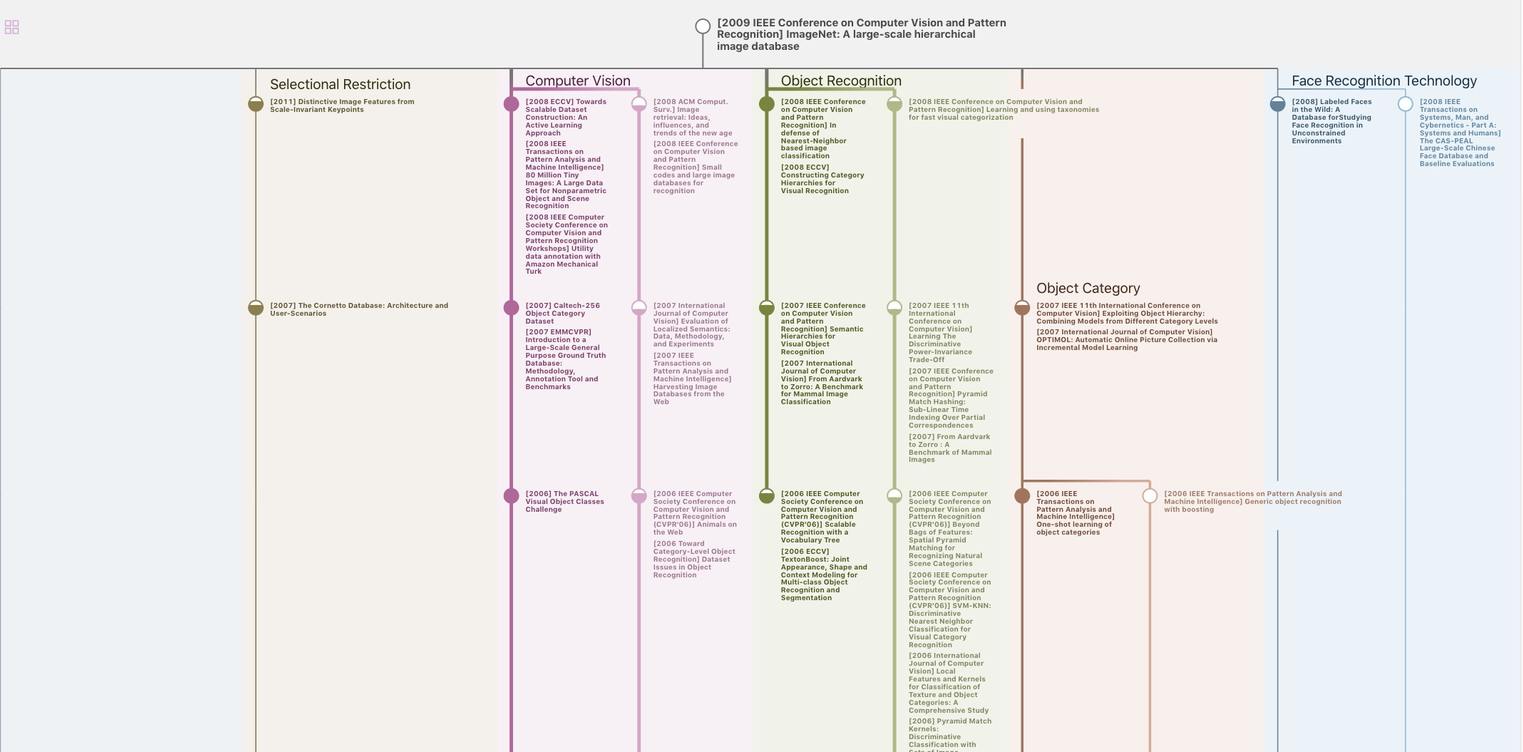
生成溯源树,研究论文发展脉络
Chat Paper
正在生成论文摘要