Model Ensemble for Click Prediction in Bing Search Ads.
WWW (Companion Volume)(2017)
摘要
Accurate estimation of the click-through rate (CTR) in sponsored ads significantly impacts the user search experience and businesses' revenue, even 0.1% of accuracy improvement would yield greater earnings in the hundreds of millions of dollars. CTR prediction is generally formulated as a supervised classification problem. In this paper, we share our experience and learning on model ensemble design and our innovation. Specifically, we present 8 ensemble methods and evaluate them on our production data. Boosting neural networks with gradient boosting decision trees turns out to be the best. With larger training data, there is a nearly 0.9% AUC improvement in offline testing and significant click yield gains in online traffic. In addition, we share our experience and learning on improving the quality of training.
更多查看译文
关键词
click prediction,bing search ads,model
AI 理解论文
溯源树
样例
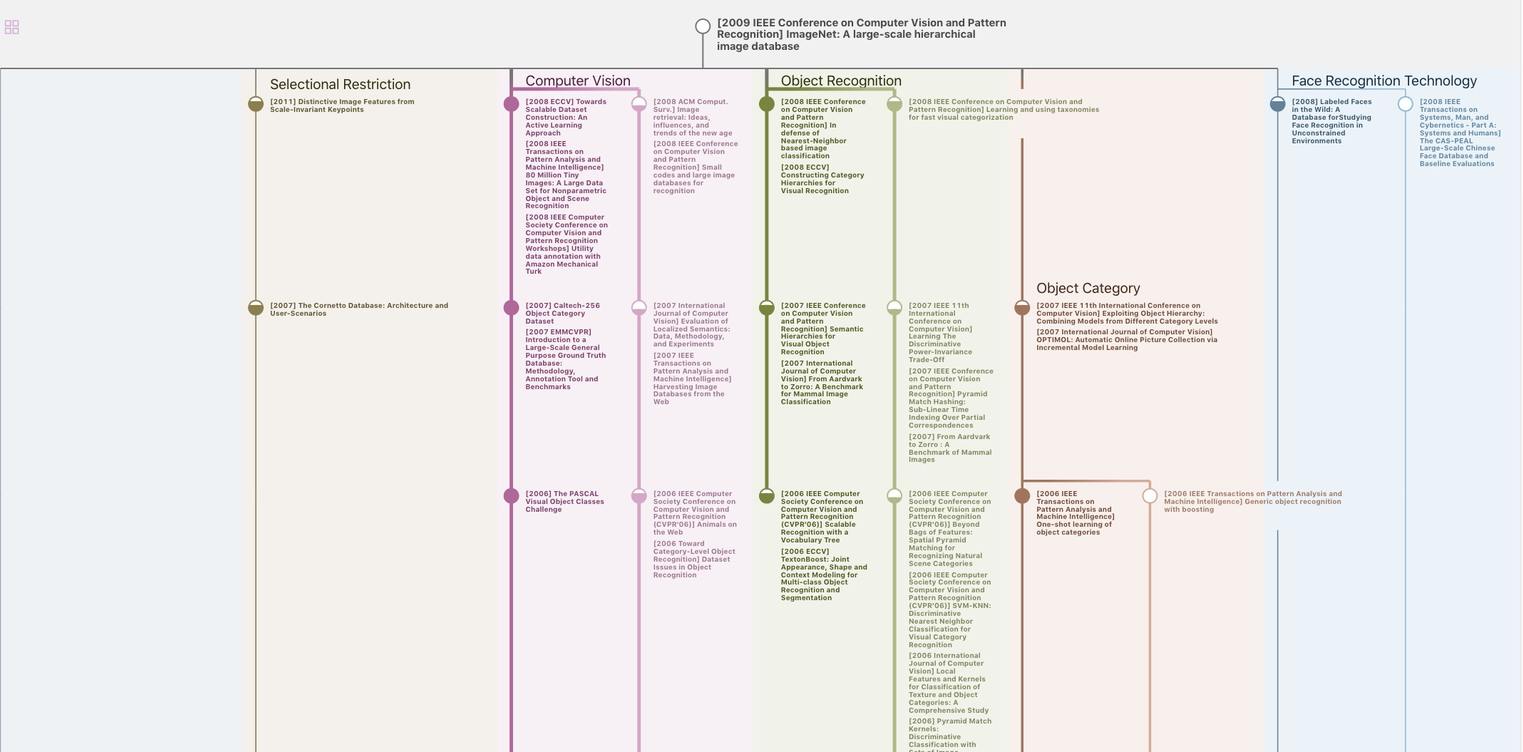
生成溯源树,研究论文发展脉络
Chat Paper
正在生成论文摘要