Neural Network-based Question Answering over Knowledge Graphs on Word and Character Level.
WWW(2017)
摘要
Question Answering (QA) systems over Knowledge Graphs (KG) automatically answer natural language questions using facts contained in a knowledge graph. Simple questions, which can be answered by the extraction of a single fact, constitute a large part of questions asked on the web but still pose challenges to QA systems, especially when asked against a large knowledge resource. Existing QA systems usually rely on various components each specialised in solving different sub-tasks of the problem (such as segmentation, entity recognition, disambiguation, and relation classification etc.). In this work, we follow a quite different approach: We train a neural network for answering simple questions in an end-to-end manner, leaving all decisions to the model. It learns to rank subject-predicate pairs to enable the retrieval of relevant facts given a question. The network contains a nested word/character-level question encoder which allows to handle out-of-vocabulary and rare word problems while still being able to exploit word-level semantics. Our approach achieves results competitive with state-of-the-art end-to-end approaches that rely on an attention mechanism.
更多查看译文
关键词
Question Answering, Knowledge Graphs
AI 理解论文
溯源树
样例
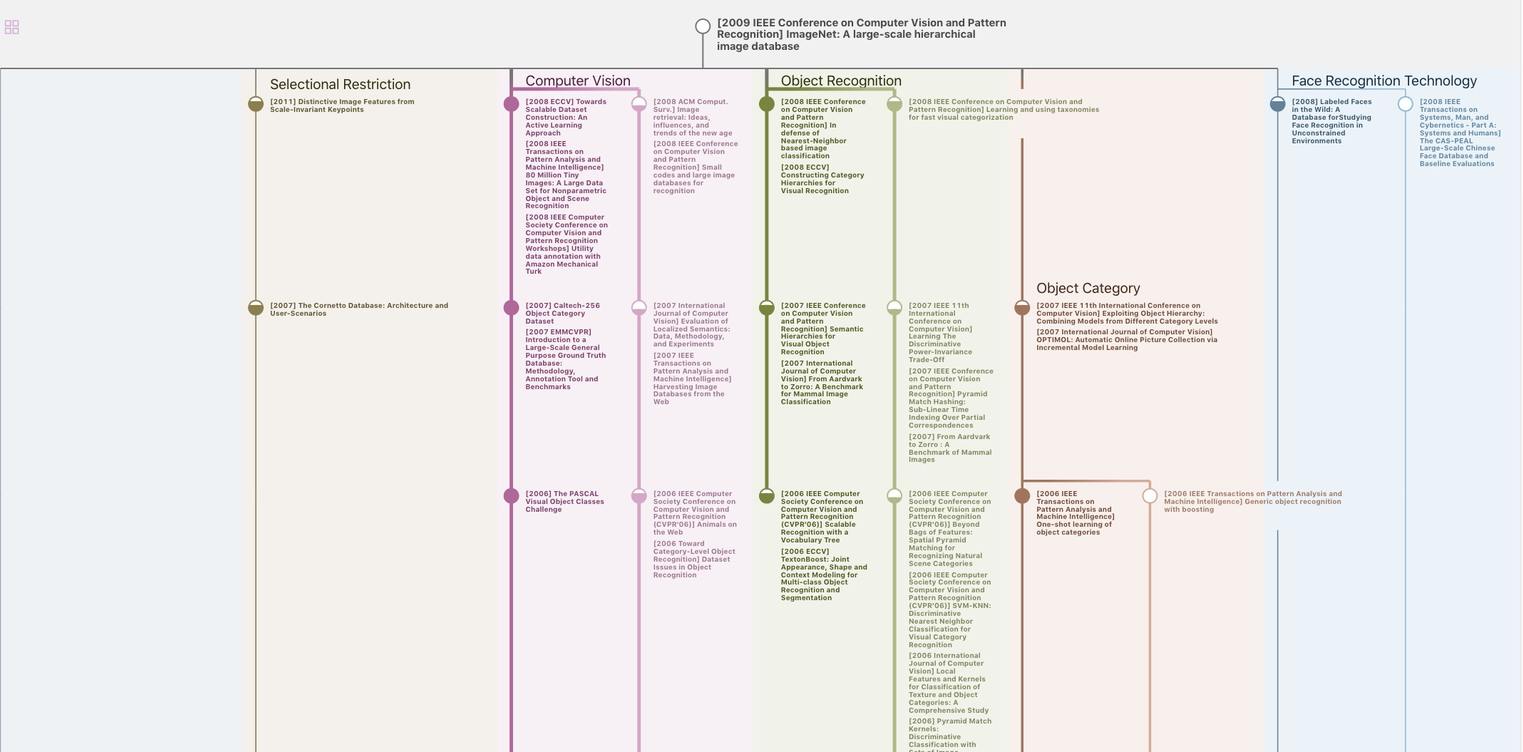
生成溯源树,研究论文发展脉络
Chat Paper
正在生成论文摘要