Algorithmic Performance-Accuracy Trade-off in 3D Vision Applications Using HyperMapper
2017 IEEE INTERNATIONAL PARALLEL AND DISTRIBUTED PROCESSING SYMPOSIUM WORKSHOPS (IPDPSW)(2017)
摘要
In this paper we investigate an emerging application, 3D scene understanding, likely to be significant in the mobile space in the near future. The goal of this exploration is to reduce execution time while meeting our quality of result objectives. In previous work we showed for the first time that it is possible to map this application to power constrained embedded systems, highlighting that decision choices made at the algorithmic design-level have the most impact. As the algorithmic design space is too large to be exhaustively evaluated, we use a previously introduced multi-objective Random Forest Active Learning prediction framework dubbed HyperMapper, to find good algorithmic designs. We show that HyperMapper generalizes on a recent cutting edge 3D scene understanding algorithm and on a modern GPU-based computer architecture. HyperMapper is able to beat an expert human hand-tuning the algorithmic parameters of the class of Computer Vision applications taken under consideration in this paper automatically. In addition, we use crowd-sourcing using a 3D scene understanding Android app to show that the Pareto front obtained on an embedded system can be used to accelerate the same application on all the 83 smart-phones and tablets crowd-sourced with speedups ranging from 2 to over 12.
更多查看译文
关键词
design space exploration,machine learning,computer vision,SLAM,embedded systems,GPU,crowd-sourcing
AI 理解论文
溯源树
样例
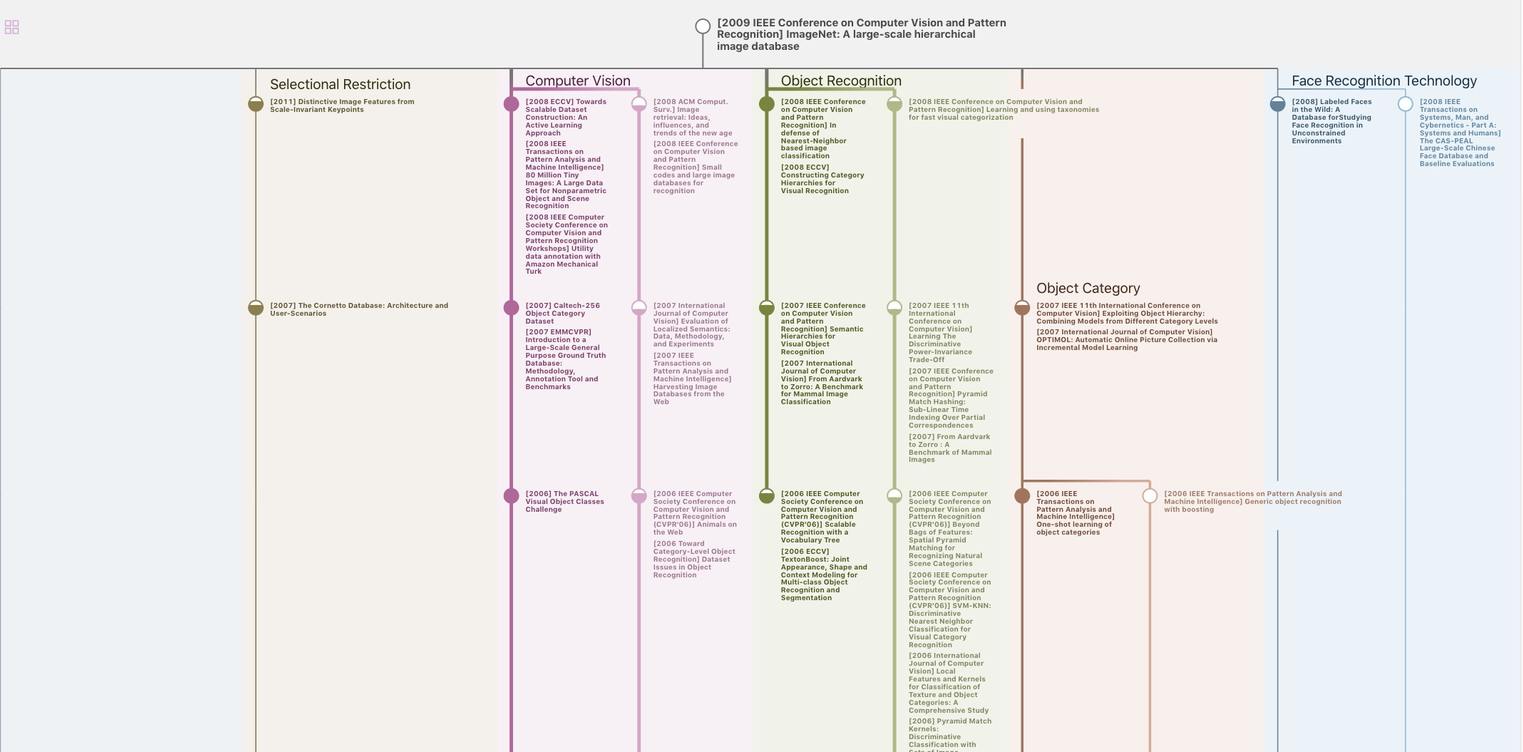
生成溯源树,研究论文发展脉络
Chat Paper
正在生成论文摘要