GPU in-memory processing using Spark for iterative computation.
CCGrid(2017)
摘要
Due to its simplicity and scalability, MapReduce has become a de facto standard computing model for big data processing. Since the original MapReduce model was only appropriate for embarrassingly parallel batch processing, many follow-up studies have focused on improving the efficiency and performance of the model. Spark follows one of these recent trends by providing in-memory processing capability to reduce slow disk I/O for iterative computing tasks. However, the acceleration of Spark's in-memory processing using graphics processing units (GPUs) is challenging due to its deep memory hierarchy and host-to-GPU communication overhead. In this paper, we introduce a novel GPU-accelerated MapReduce framework that extends Spark's in-memory processing so that iterative computing is performed only in the GPU memory. Having discovered that the main bottleneck in the current Spark system for GPU computing is data communication on a Java virtual machine, we propose a modification of the current Spark implementation to bypass expensive data management for iterative task offloading to GPUs. We also propose a novel GPU in-memory processing and caching framework that minimizes host-to-GPU communication via lazy evaluation and reuses GPU memory over multiple mapper executions. The proposed system employs message-passing interface (MPI)-based data synchronization for inter-worker communication so that more complicated iterative computing tasks, such as iterative numerical solvers, can be efficiently handled. We demonstrate the performance of our system in terms of several iterative computing tasks in big data processing applications, including machine learning and scientific computing. We achieved up to 50 times speed up over conventional Spark and about 10 times speed up over GPU-accelerated Spark.
更多查看译文
关键词
Spark, MapReduce, GPU, In-memory Computing
AI 理解论文
溯源树
样例
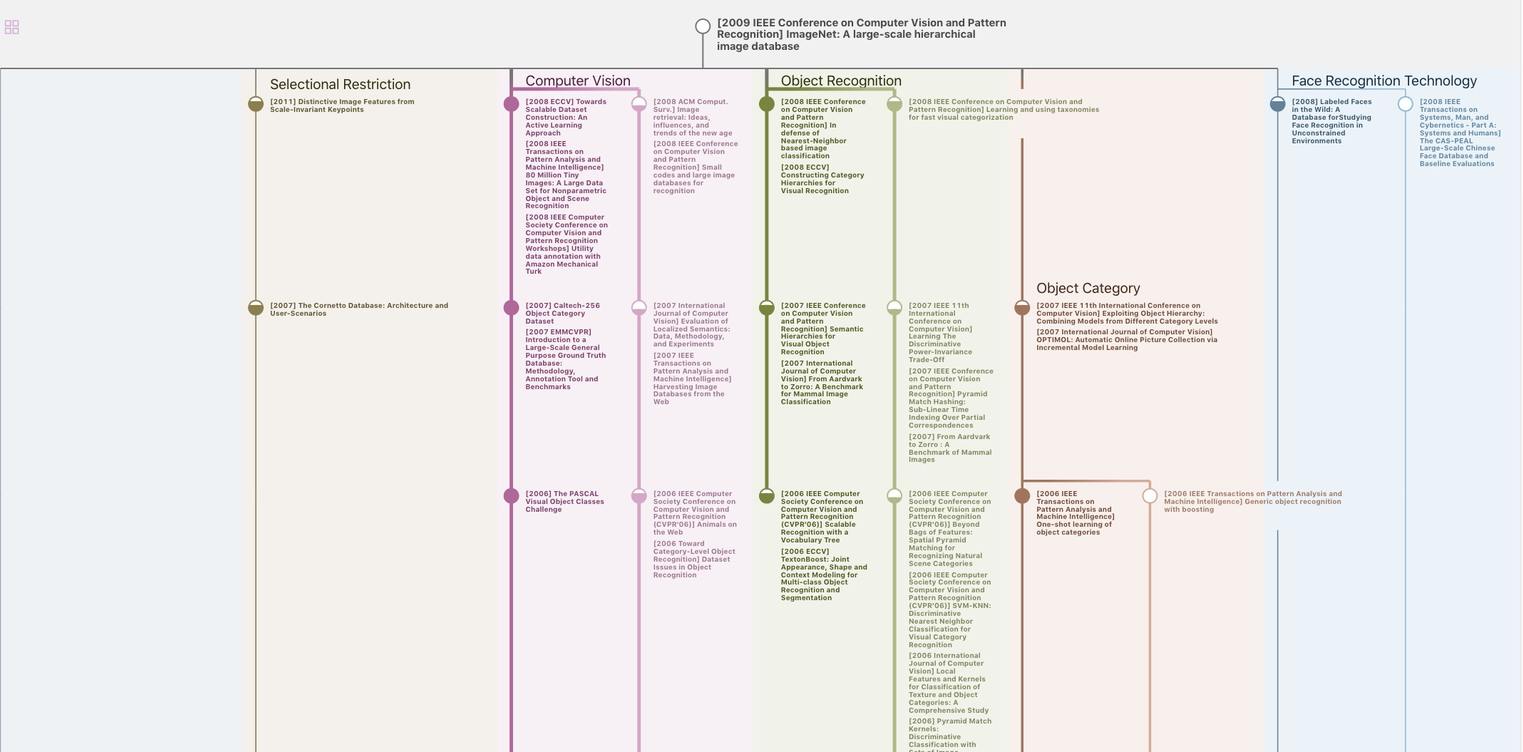
生成溯源树,研究论文发展脉络
Chat Paper
正在生成论文摘要