Multi-Agent Surveillance And Tracking Using Cyclic Stochastic Gradient
2016 AMERICAN CONTROL CONFERENCE (ACC)(2016)
摘要
This paper formulates a solution to a problem in stochastic multi-agent and multi-target surveillance. The research goal is to implement a cyclic stochastic optimization (CSO) application for an ensemble of cooperating, mobile sensing agents executing a surveillance mission. Each agent continually combines information to determine its best estimate of the multi-target system state and then decides on its next action. This decision is selected to optimize a criterion related to the Fisher information matrix. For CSO, only noisy measurements of the objective function are available to each agent, and in this study, each agent attempts to optimize this objective function by calculating its stochastic gradient. This paper examines the implications and applicability of CSO convergence via simulation-based experiments. In these experiments, a cooperating multi-agent ensemble of sensing agents search a region for targets and, using our proposed algorithm, maintain track on all discovered targets.
更多查看译文
关键词
multiagent tracking,cyclic stochastic gradient,stochastic multiagent surveillance,stochastic multitarget surveillance,cyclic stochastic optimization,CSO application,cooperating mobile sensing agents,surveillance mission,agent information,multitarget system state estimation,agent action decision,criterion optimization,Fisher information matrix,noisy measurement,objective function optimization,CSO convergence,simulation-based experiment,target tracking
AI 理解论文
溯源树
样例
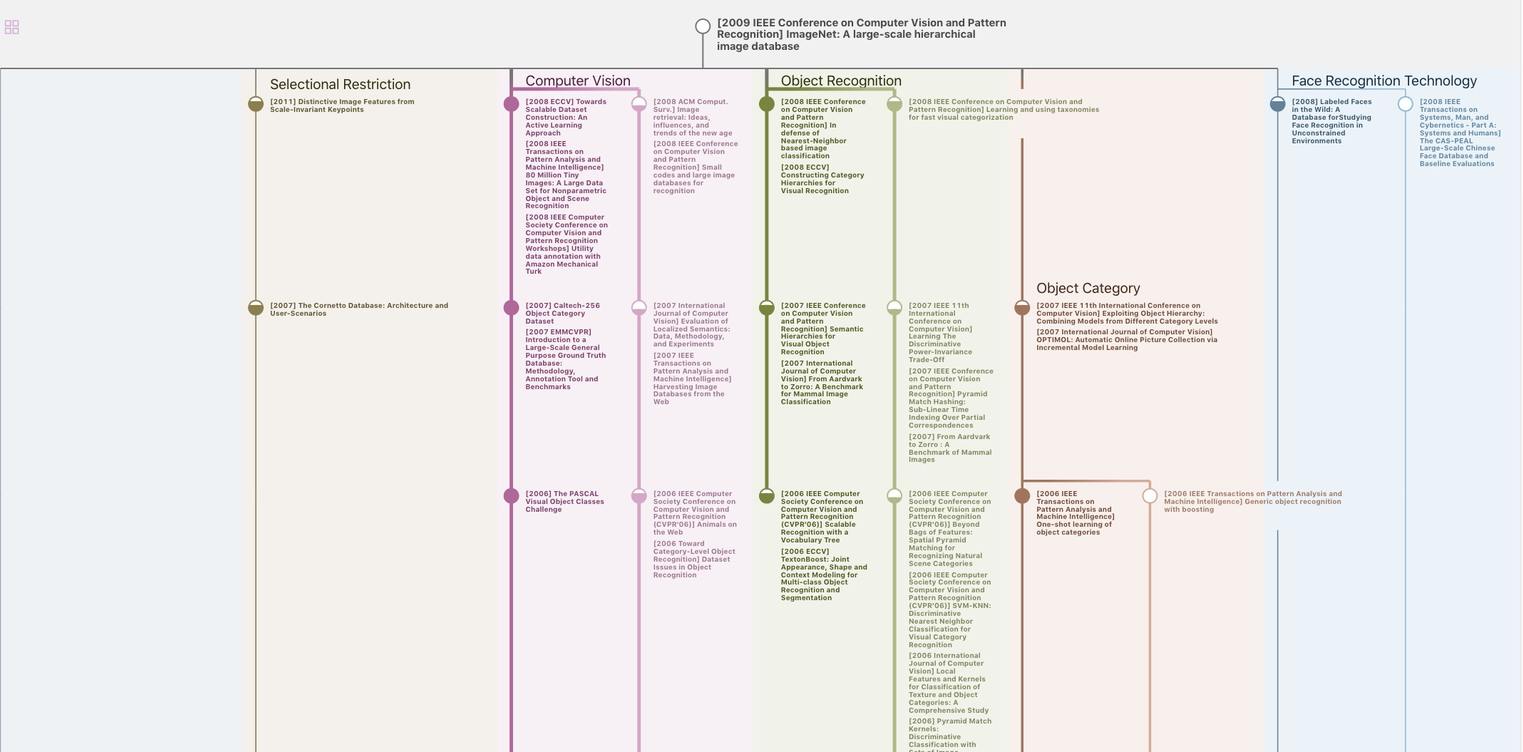
生成溯源树,研究论文发展脉络
Chat Paper
正在生成论文摘要