Fault Detection For Ironmaking Process Based On Stacked Denoising Autoencoders
2016 AMERICAN CONTROL CONFERENCE (ACC)(2016)
摘要
It is quite challenging to monitor an ironmaking process due to some of its special characteristics such as lack of direct measurements and strong disturbances. Hence extracting robust features of the normal process from complex historical data is vitally important. Denoising autoencoder (dA), a recently developed deep learning technique, has become a popular tool to extract and compose robust features. However, its application to fault detection in process control fields are still limited. In this paper, a denoising autoencoder based monitoring approach is proposed for a practical ironmaking process, in which peak-like disturbances due to the switchings between two arbitrary distinct host-blast stoves are involved. To validate the proposed monitoring method, the data corresponding to a cold furnace fault of the process is used and comparative fault detection performances with the existing methods are presented.
更多查看译文
关键词
ironmaking process,stacked denoising autoencoders,feature extraction,deep learning technique,monitoring method,cold furnace fault,fault detection performances
AI 理解论文
溯源树
样例
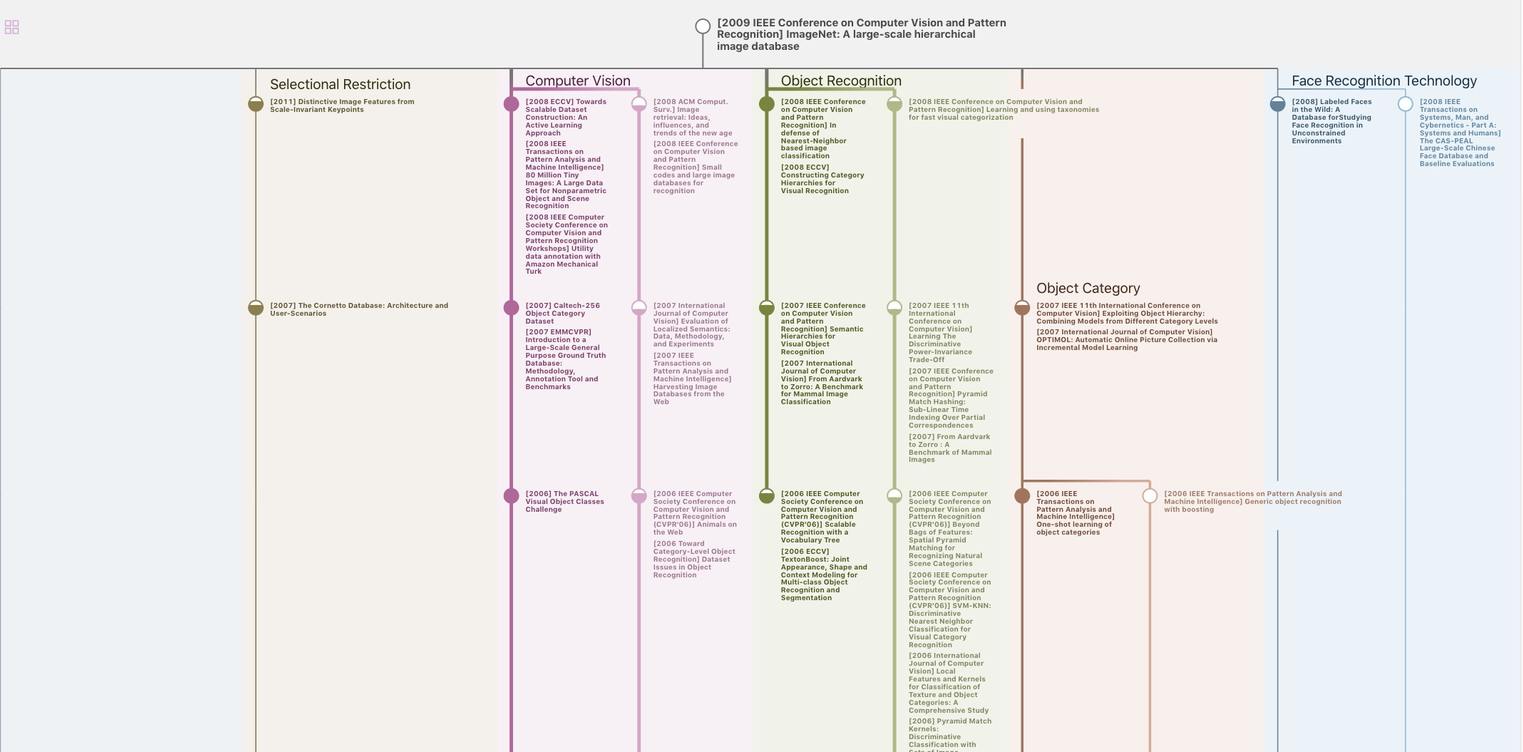
生成溯源树,研究论文发展脉络
Chat Paper
正在生成论文摘要