Fast Incremental Objects Identification And Localization Using Cross-Correlation On A 6 Dof Voting Scheme
2014 PROCEEDINGS OF THE 9TH INTERNATIONAL CONFERENCE ON COMPUTER GRAPHICS THEORY AND APPLICATIONS (GRAPP 2014)(2014)
Abstract
In this work, we propose a sparse features-based object recognition and localization system, well suited for online learning of new objects. Our method takes advantages of both depth and ego-motion information, along with salient feature descriptors information, in order to learn and recognize objects with a scalable approach. We extend the conventional probabilistic voting scheme for object the recognition task, proposing a correlation-based approach in which each object-related point feature contributes in a 6-dimensional voting space (i.e., the 6 degrees-of-freedom, DoF, object position) with a continuous probability density distribution (PDF) represented by a Mixture of Gaussian (MoG). A global PDF is then obtained adding the contribution of each feature. The object instance and pose are hence inferred exploiting an efficient mode-finding method for mixtures of Gaussian distributions. The special properties of the convolution operator for the MoG distributions, combined with the sparsity of the exploited data, provide our method with good computational efficiency and limited memory requirements, enabling real-time performances also in robots with limited resources.
MoreTranslated text
Key words
Gaussian Mixtures,Online Learning,Pose Estimation,Object Recognition
AI Read Science
Must-Reading Tree
Example
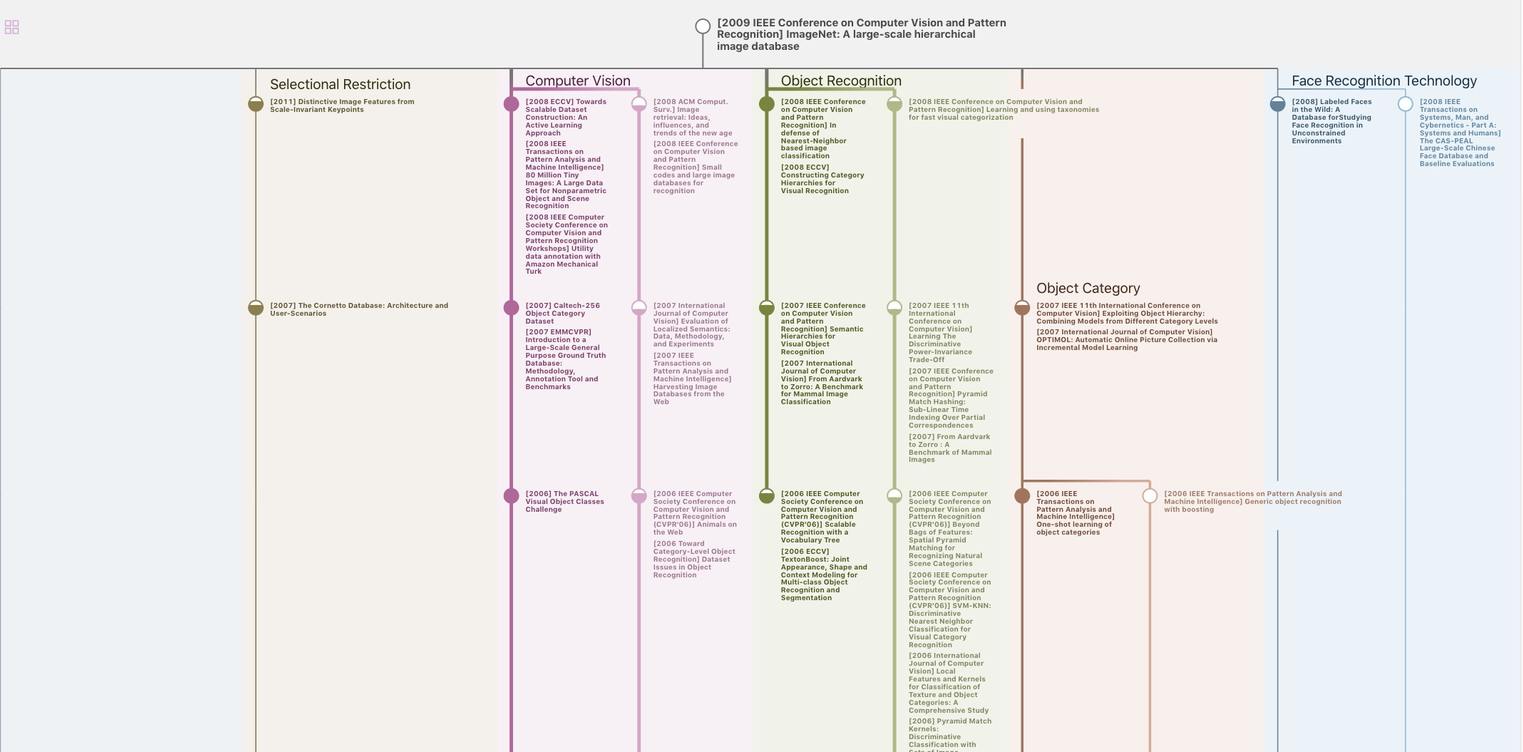
Generate MRT to find the research sequence of this paper
Chat Paper
Summary is being generated by the instructions you defined