A deep learning approach to traffic lights: Detection, tracking, and classification.
ICRA(2017)
摘要
Reliable traffic light detection and classification is crucial for automated driving in urban environments. Currently, there are no systems that can reliably perceive traffic lights in real-time, without map-based information, and in sufficient distances needed for smooth urban driving. We propose a complete system consisting of a traffic light detector, tracker, and classifier based on deep learning, stereo vision, and vehicle odometry which perceives traffic lights in real-time. Within the scope of this work, we present three major contributions. The first is an accurately labeled traffic light dataset of 5000 images for training and a video sequence of 8334 frames for evaluation. The dataset is published as the Bosch Small Traffic Lights Dataset and uses our results as baseline. It is currently the largest publicly available labeled traffic light dataset and includes labels down to the size of only 1 pixel in width. The second contribution is a traffic light detector which runs at 10 frames per second on 1280×720 images. When selecting the confidence threshold that yields equal error rate, we are able to detect traffic lights as small as 4 pixels in width. The third contribution is a traffic light tracker which uses stereo vision and vehicle odometry to compute the motion estimate of traffic lights and a neural network to correct the aforementioned motion estimate.
更多查看译文
关键词
deep learning,traffic light detection,traffic light classification,traffic light tracking,automated driving,real-time traffic light perception,smooth urban driving,stereo vision,vehicle odometry,labeled traffic light dataset,video sequence,Bosch Small Traffic Lights dataset,confidence threshold selection,motion estimation,neural network
AI 理解论文
溯源树
样例
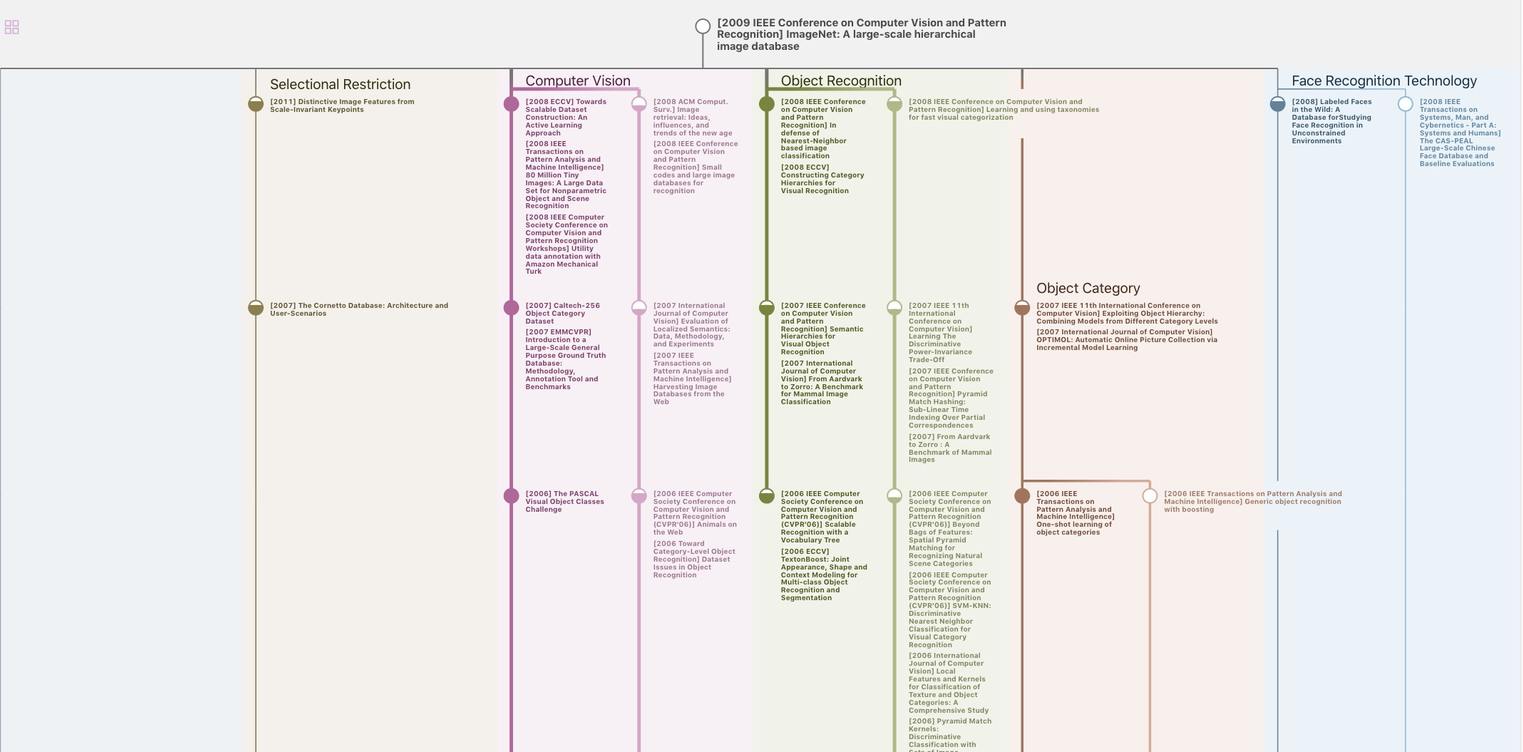
生成溯源树,研究论文发展脉络
Chat Paper
正在生成论文摘要