Instruction completion through instance-based learning and semantic analogical reasoning.
ICRA(2017)
摘要
As autonomous, mobile robots are increasingly entering our everyday lives and the tasks they are to perform are getting continuously more complex and versatile, instructing robots by means of natural-language commands becomes more and more important. Such instructions, stated by humans and originally intended for human use, are typically formulated very vaguely and lack critical information about how to perform particular actions. Probabilistic relational models have shown promise in filling in missing information pieces that have been omitted in such instructions. However, the enormous size of these models and the computational expense in learning and reasoning often impedes their practical applicability to real-world domains. In this work, we propose a novel instance-based learning approach towards building up knowledge bases for instruction completion, which combines probabilistic methods with semantic analogical reasoning. Probabilistic reasoning is employed to build up a knowledge base of natural-language instruction sheets, while instruction completion can be achieved through fast database queries. We showcase the scalabilty of our approach by building up a KB of more than 100,000 instruction steps that have been mined from the wikihow.com web site and which are publicly accessible from within the Prac [21] natural-language interpreter.
更多查看译文
关键词
instance-based learning,knowledge bases,semantic analogical reasoning,probabilistic reasoning,natural-language instruction sheets,instruction completion,database queries,KB,PRAC natural-language interpreter,autonomous mobile robots
AI 理解论文
溯源树
样例
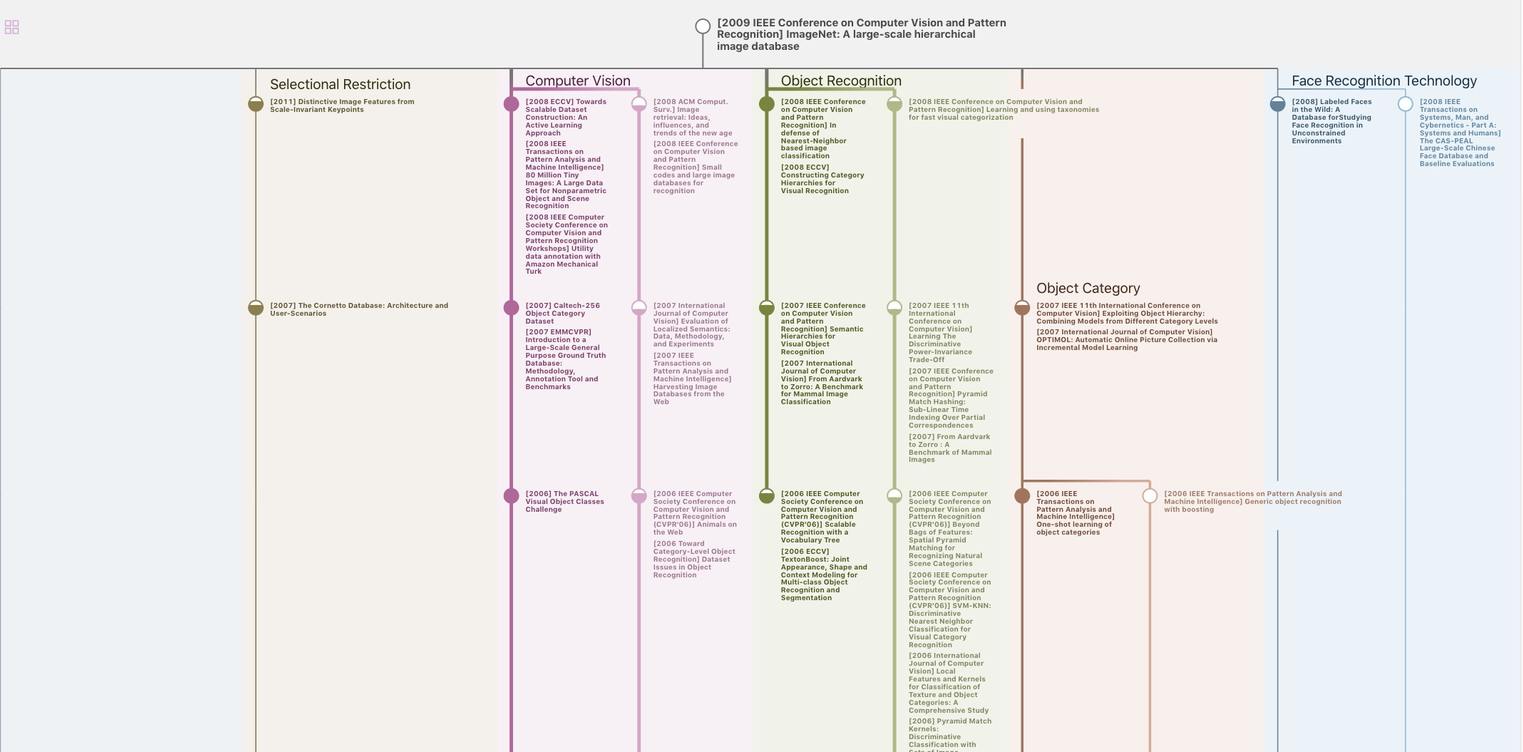
生成溯源树,研究论文发展脉络
Chat Paper
正在生成论文摘要