Temporal Models for Robot Classification of Human Interruptibility.
AAMAS(2017)
摘要
Robots are increasingly being deployed in unstructured human environments where they will need to approach and interrupt collocated humans. Most prior work on robot interruptions has focused on how to interrupt a person or on estimating a human's awareness of the robot. Our work makes three contributions to this research area. First, we introduce an ordinal scale of interruptibility that can be used to rate the interruptibility of a human. Second, we propose the use of Conditional Random Fields (CRFs) and their variants, Hidden CRFs, and Latent-Dynamic CRFs, for classifying interruptibility. Third, we introduce the use of object labels as a visual cue to the context of an interruption in order to improve interruptibility estimates. Our results show that Latent-Dynamic CRFs outperform all other models across all tested conditions, and that the inclusion of object labels as a cue to context improves interruptibility classification performance, yielding the best overall results.
更多查看译文
关键词
Human-Robot Interaction,Interruptibility,Conditional Random Fields
AI 理解论文
溯源树
样例
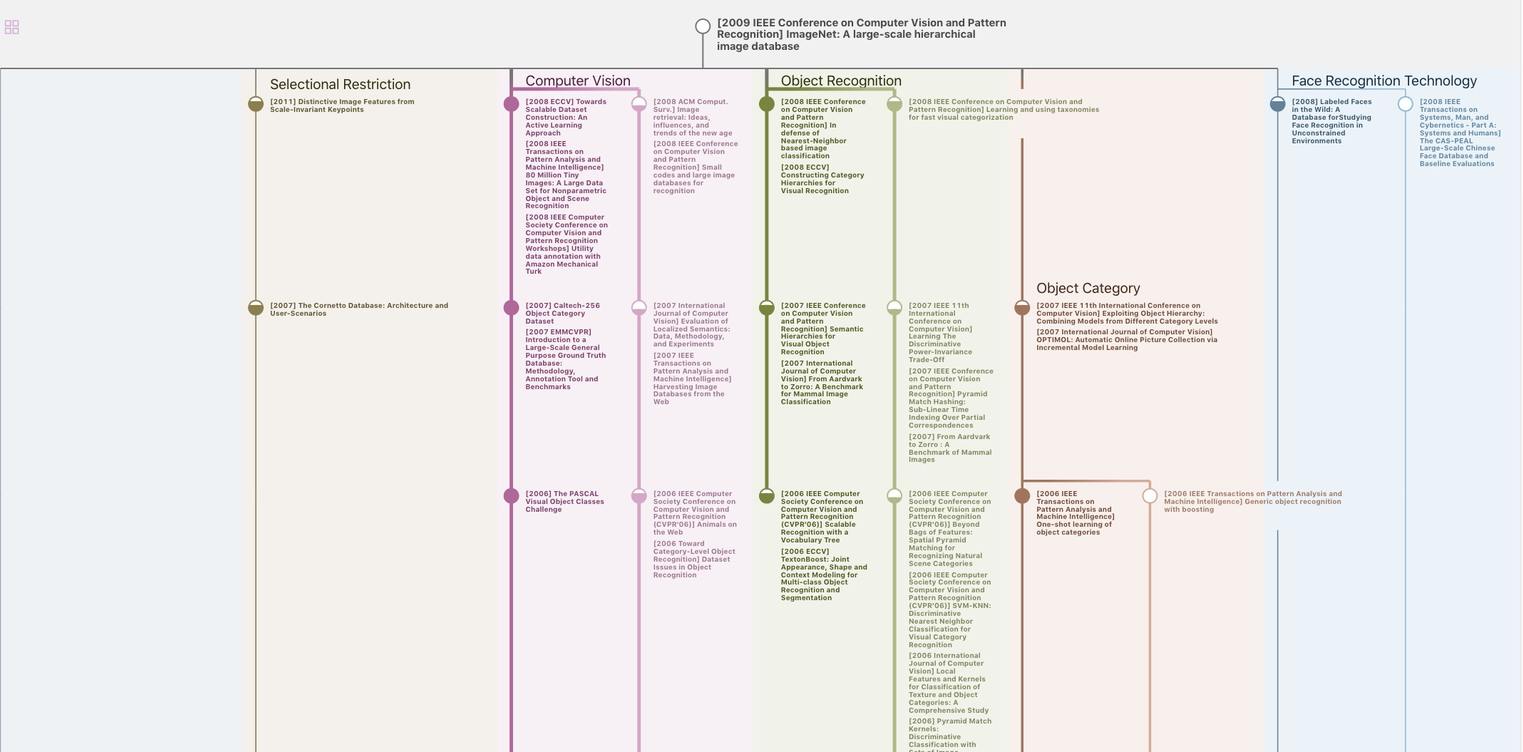
生成溯源树,研究论文发展脉络
Chat Paper
正在生成论文摘要