Ensemble Learning Based Urban Traffic State Prediction For Coupling Traffic Network With Large Scale Data
2016 IEEE 19TH INTERNATIONAL CONFERENCE ON INTELLIGENT TRANSPORTATION SYSTEMS (ITSC)(2016)
Abstract
Current urban transportation system applications often deal with the scenarios of large scale multiple-layer road networks, with the requirement of qualified overall prediction accuracy. However, the common related studies mostly focus on the prediction of individual road segments or intersections. In this paper, we propose a generic method for large scale coupling urban traffic to forecast the future states of the overall network. In the preliminary stage, the features are extracted using unsupervised method. Subsequently, an ensemble learning via matching the historical data and the current information are implemented to predict the traffic state transition. The data set for experiment is composed of multiple layers of road network in Shanghai, which are collected by loop detectors every two minutes. We verify the effectiveness of the developed method by evaluating the experimental prediction performance using the actual data. The results also indicate that adding the interactive features of the coupling road network to the forecasting algorithm can generate superior prediction accuracy comparing with the independent prediction method.
MoreTranslated text
Key words
learning based urban traffic state prediction,urban transportation system applications,multiple-layer road networks,road segment prediction,unsupervised method,feature extraction,traffic state transition,coupling road network interactive features,forecasting algorithm,prediction accuracy
AI Read Science
Must-Reading Tree
Example
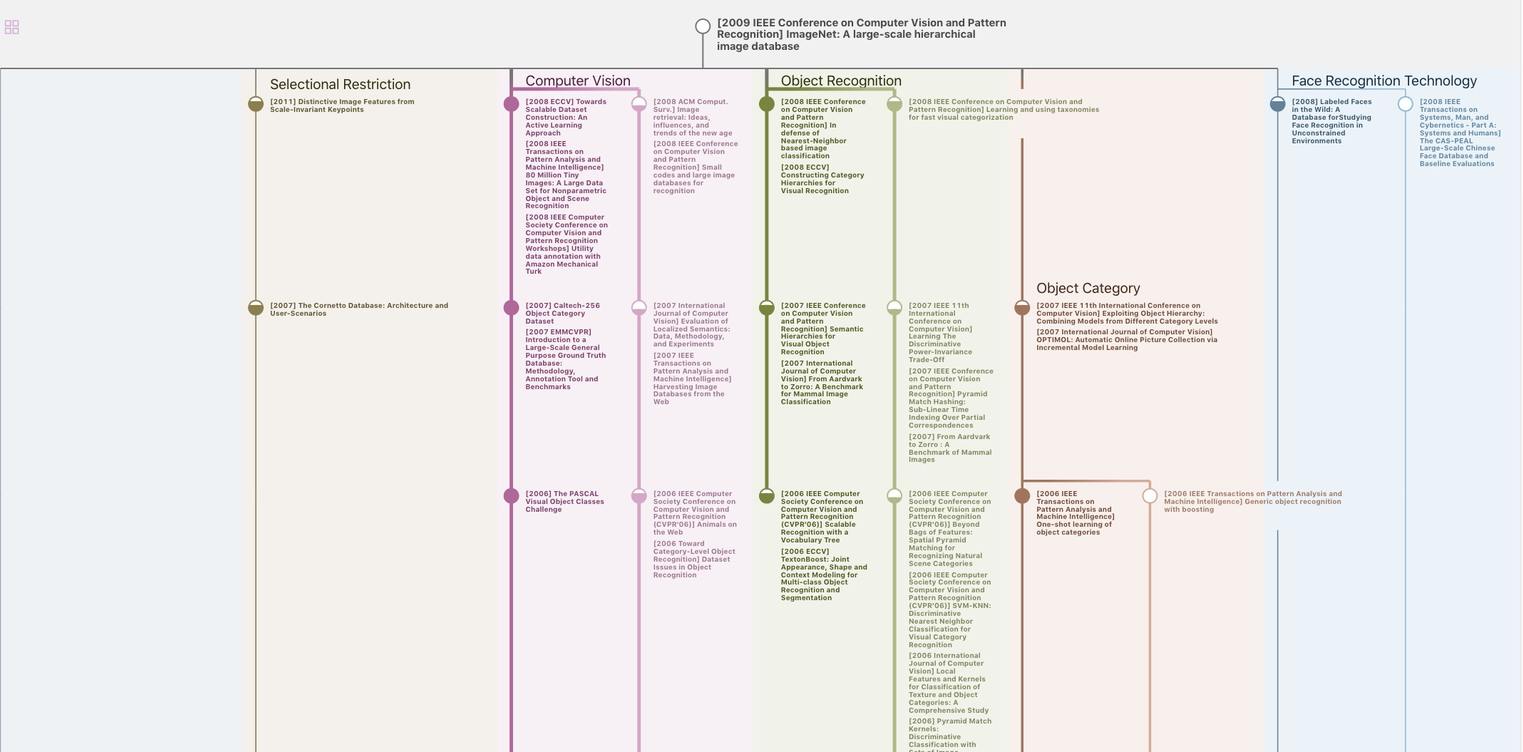
Generate MRT to find the research sequence of this paper
Chat Paper
Summary is being generated by the instructions you defined