Efficient Bayesian Yield Optimization Approach for Analog and SRAM Circuits.
DAC(2017)
摘要
Conventional yield optimization approaches rely on accurate yield estimation for given design parameters, which would be computational intensive. In this paper, a novel Bayesian yield optimization approach is proposed for analog and SRAM circuits. An equivalent a problem is formulated via applying Bayes' theorem on the augmented yield problem. The yield optimization problem is converted to identifying the design parameters with maximal probability density conditioning on the event that the corresponding circuit is \"pass\". Gaussian kernel density estimation is employed to approximate the conditional probability, and a multi-start-point based EM-like algorithm is proposed to solve the equivalent problem efficiently Compared with the state-of-the-art yield optimization approaches, the proposed method can significantly reduce the number of circuit simulations with comparable optimization accuracy by avoiding repetitive yield estimations.
更多查看译文
关键词
conditional probability,analog circuits,multistart-point based EM-like algorithm,gaussian kernel density estimation,corresponding circuit,maximal probability density conditioning,yield optimization problem,augmented yield problem,equivalent problem,novel Bayesian yield optimization approach,accurate yield estimation,SRAM circuits,efficient Bayesian yield optimization approach,repetitive yield estimations,comparable optimization accuracy,circuit simulations
AI 理解论文
溯源树
样例
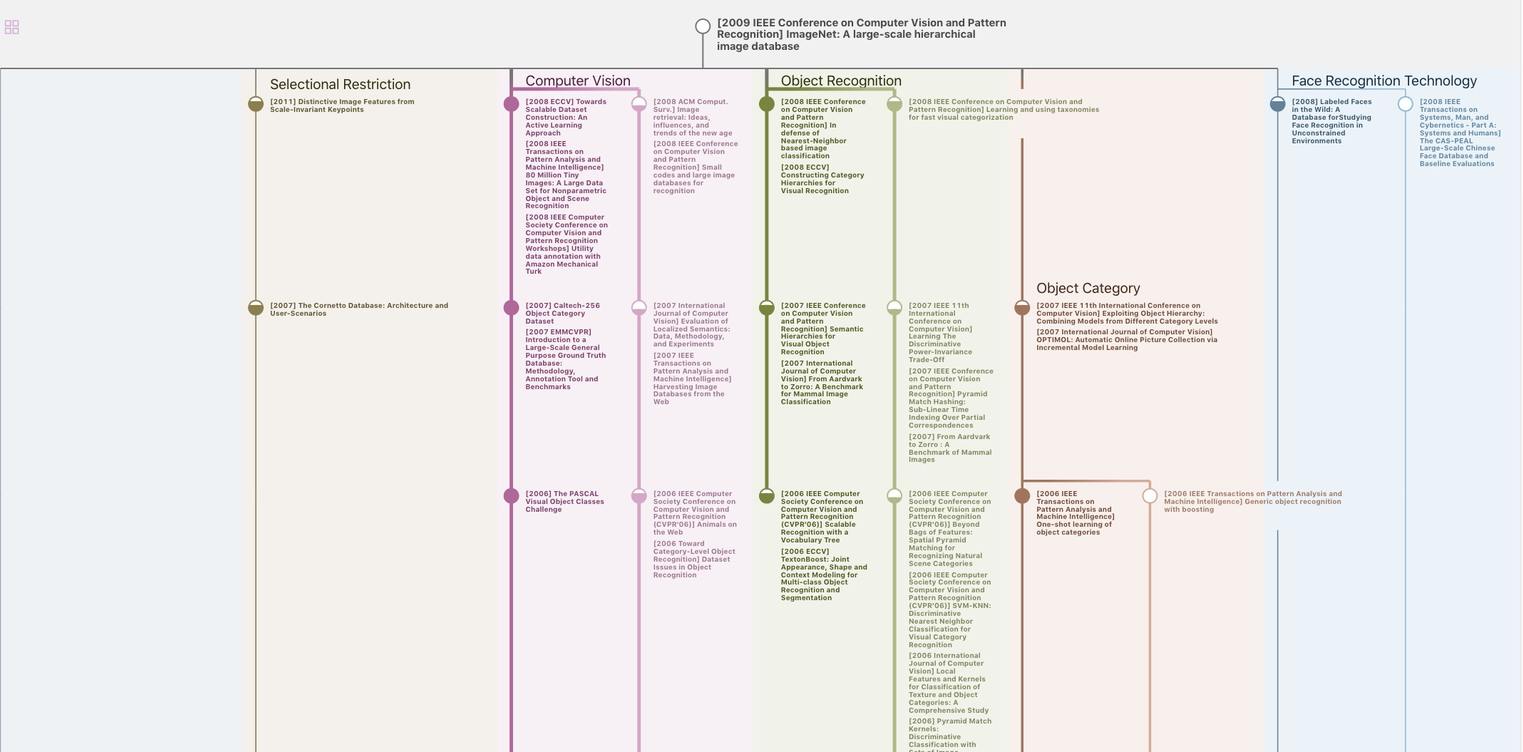
生成溯源树,研究论文发展脉络
Chat Paper
正在生成论文摘要