A Bayesian feature allocation model for tumor heterogeneity
ANNALS OF APPLIED STATISTICS(2015)
摘要
We develop a feature allocation model for inference on genetic tumor variation using next-generation sequencing data. Specifically, we record single nucleotide variants (SNVs) based on short reads mapped to human reference genome and characterize tumor heterogeneity by latent haplotypes defined as a scaffold of SNVs on the same homologous genome. For multiple samples from a single tumor, assuming that each sample is composed of some sample-specific proportions of these haplotypes, we then fit the observed variant allele fractions of SNVs for each sample and estimate the proportions of haplotypes. Varying proportions of haplotypes across samples is evidence of tumor heterogeneity since it implies varying composition of cell subpopulations. Taking a Bayesian perspective, we proceed with a prior probability model for all relevant unknown quantities, including, in particular, a prior probability model on the binary indicators that characterize the latent haplotypes. Such prior models are known as feature allocation models. Specifically, we define a simplified version of the Indian buffet process, one of the most traditional feature allocation models. The proposed model allows overlapping clustering of SNVs in defining latent haplotypes, which reflects the evolutionary process of subclonal expansion in tumor samples.
更多查看译文
关键词
Haplotypes,feature allocation models,Indian buffet process,Markov chain Monte Carlo,next-generation sequencing,random binary matrices,variant calling
AI 理解论文
溯源树
样例
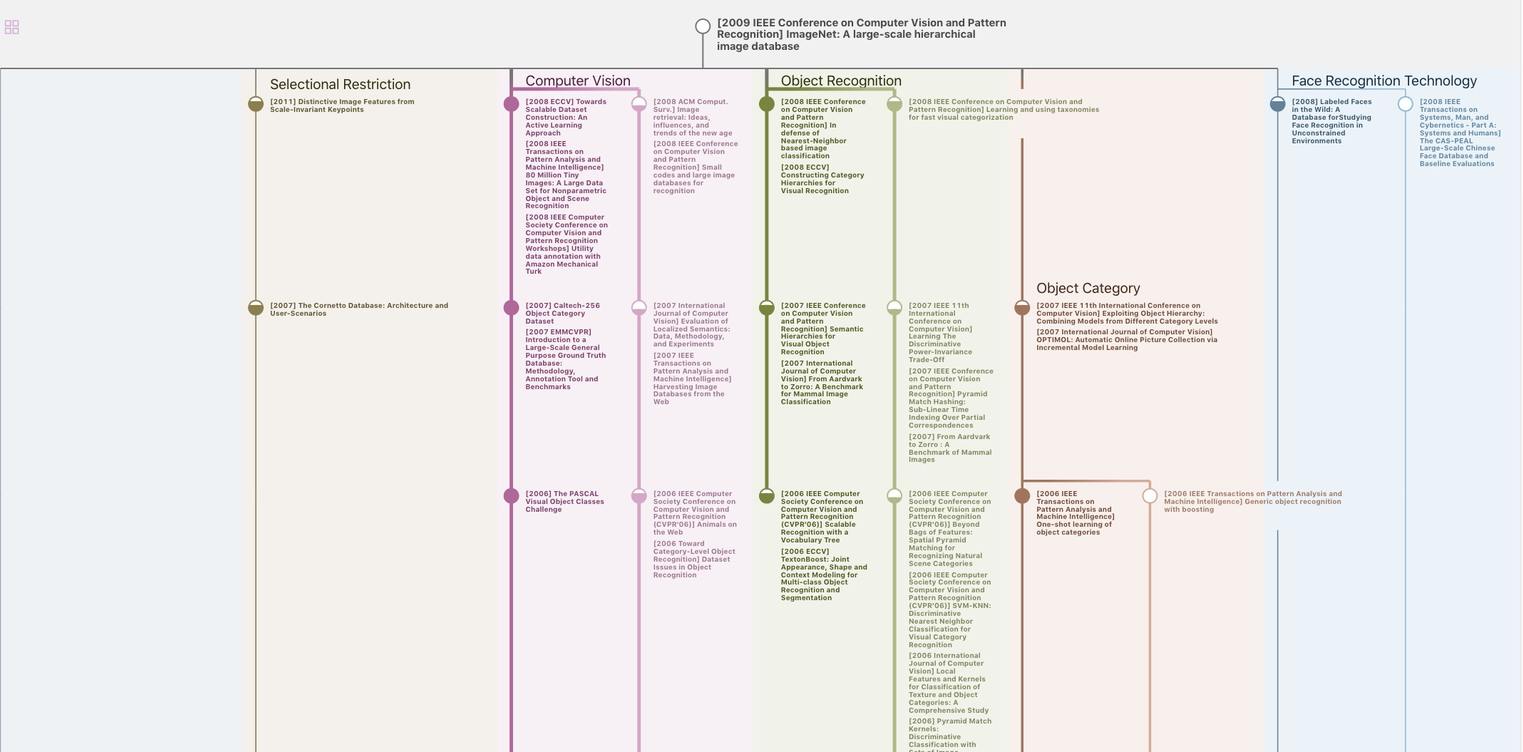
生成溯源树,研究论文发展脉络
Chat Paper
正在生成论文摘要