Fast Many-to-One Voice Conversion using Autoencoders.
ICAART: PROCEEDINGS OF THE 9TH INTERNATIONAL CONFERENCE ON AGENTS AND ARTIFICIAL INTELLIGENCE, VOL 2(2017)
摘要
Most of voice conversion (VC) methods were dealing with a one-to-one VC issue and there were few studies that tackled many-to-one / many-to-many cases. It is difficult to prepare the training data for an application with the methods because they require a lot of parallel data. Furthermore, the length of time required to convert a speech by Deep Neural Network (DNN) gets longer than pre-DNN methods because the DNN-based methods use complicated networks. In this study, we propose a VC method using autoencoders in order to reduce the amount of the training data and to shorten the converting time. In the method, higher-order features are extracted from acoustic features of source speakers by an autoencoder trained with source speakers' data. Then they are converted to higher-order features of a target speaker by DNN. The converted higher-order features are restored to the acoustic features by an autoencoder trained with data drawn from the target speaker. In the evaluation experiment, the proposed method outperforms the conventional VC methods that use Gaussian Mixture Models (GMM) and DNNs in both one-to-one conversion and many-to-one conversion with a small training set in terms of the conversion accuracy and the converting time.
更多查看译文
关键词
Voice Conversion,Autoencoder,Deep Learning,Deep Neural Network,Spectral Envelope
AI 理解论文
溯源树
样例
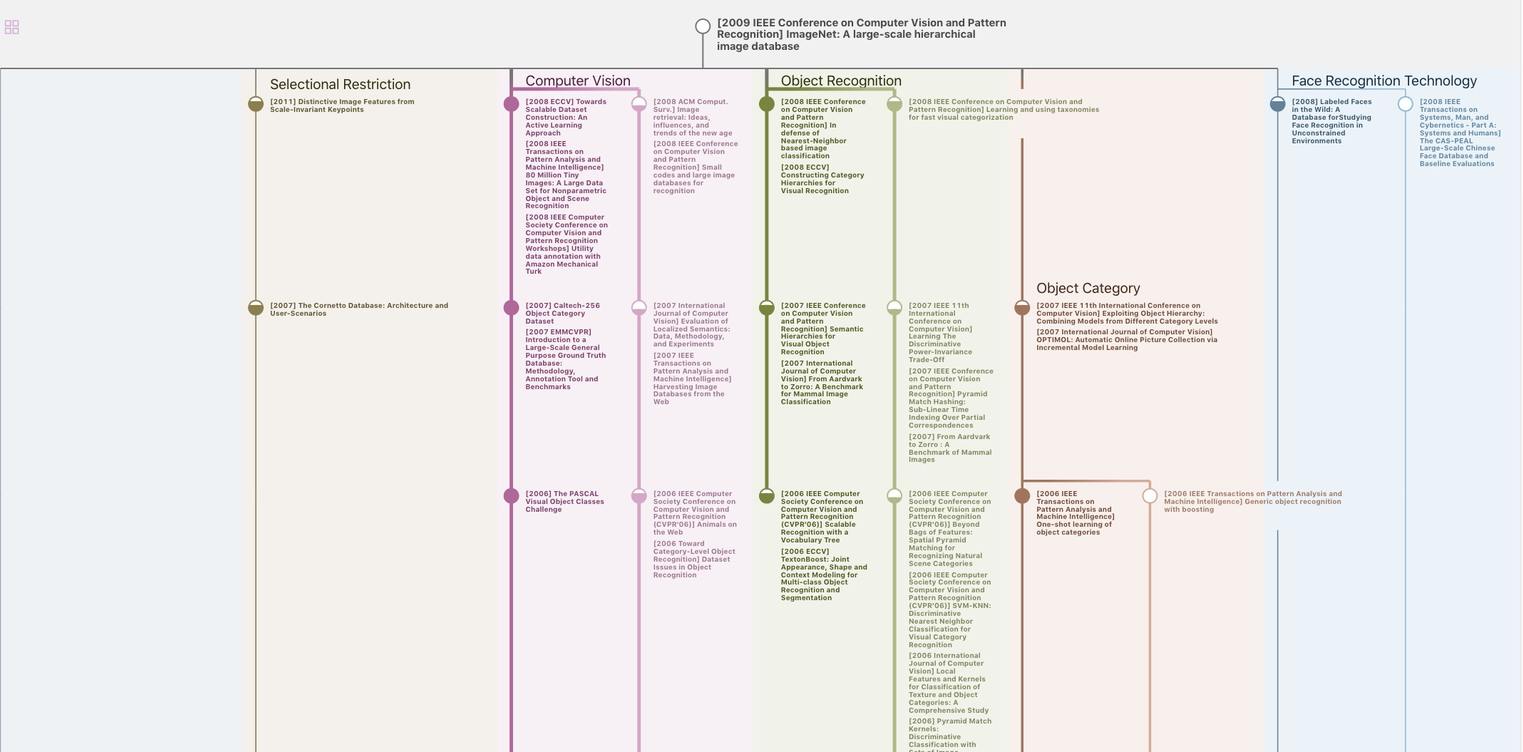
生成溯源树,研究论文发展脉络
Chat Paper
正在生成论文摘要