Pre-emphasizing Binarized Ensembles to Improve Classification Performance.
ADVANCES IN COMPUTATIONAL INTELLIGENCE, IWANN 2017, PT I(2017)
摘要
Machine ensembles are learning architectures that offer high expressive capacities and, consequently, remarkable performances. This is due to their high number of trainable parameters. In this paper, we explore and discuss whether binarization techniques are effective to improve standard diversification methods and if a simple additional trick, consisting in weighting the training examples, allows to obtain better results. Experimental results, for three selected classification problems, show that binarization permits that standard direct diversification methods (bagging, in particular) achieve better results, obtaining even more significant performance improvements when pre-emphasizing the training samples. Some research avenues that this finding opens are mentioned in the conclusions.
更多查看译文
关键词
Classification,Multi-Layer Perceptron,Ensemble classifiers,Bagging,ECOC
AI 理解论文
溯源树
样例
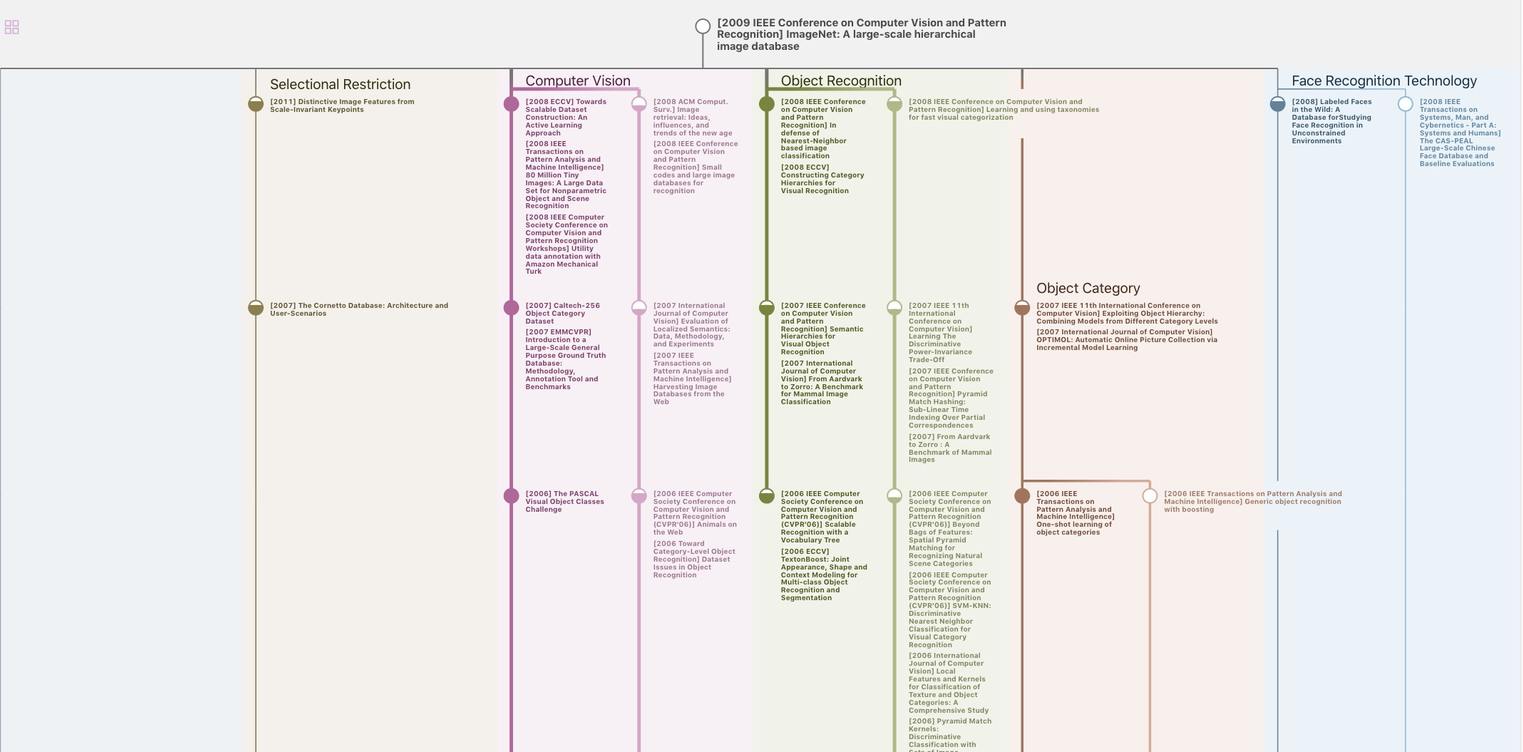
生成溯源树,研究论文发展脉络
Chat Paper
正在生成论文摘要