Abnormal Group User Detection in Recommender Systems Using Multi-dimension Time Series.
Lecture Notes of the Institute for Computer Sciences, Social Informatics, and Telecommunications Engineering(2016)
摘要
Collaborative filtering based recommender systems are capable of generating personalized recommendations, which are tools to alleviate information overload problem. However, due to the open nature of recommender systems, they are vulnerable to shilling attacks which insert forged user profiles to alter the recommendation list of targeted items. Previous research related to robustness of recommender systems has focused on detecting malicious profiles. Most approaches focus on profile classification but ignore the group attributes among shilling attack profiles. A method for detecting suspicious ratings by constructing multidimension time series TS-TIA is proposed. We reorganize all ratings on each item sorted by time series, each time series is examined and suspicious rating segments are checked. Then statistical metrics and target item analysis techniques are used to detect shilling attacks in these anomaly rating segments. Experiments show that our proposed method can be effective and less time consuming at detecting items under attacks in greater datasets.
更多查看译文
关键词
Abnormal group users,Shilling attack detection,Time series,Recommender system
AI 理解论文
溯源树
样例
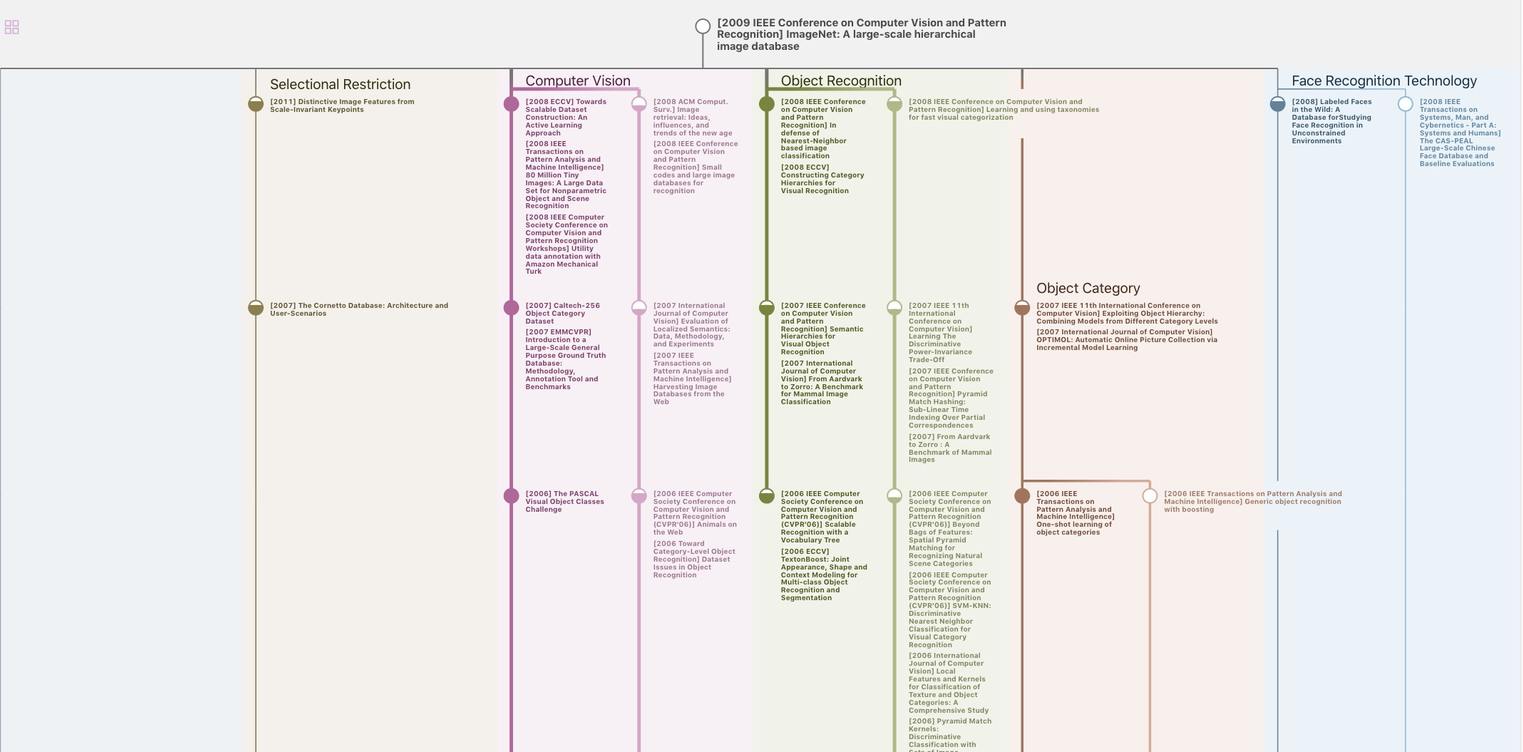
生成溯源树,研究论文发展脉络
Chat Paper
正在生成论文摘要