Urban event detection with big data of taxi OD trips: A time series decomposition approach.
TRANSACTIONS IN GIS(2017)
摘要
Big urban mobility data, such as taxi trips, cell phone records, and geo-social media check-ins, offer great opportunities for analyzing the dynamics, events, and spatiotemporal trends of the urban social landscape. In this article, we present a new approach to the detection of urban events based on location-specific time series decomposition and outlier detection. The approach first extracts long-term temporal trends and seasonal periodicity patterns. Events are defined as anomalies that deviate significantly from the prediction with the discovered temporal patterns, i.e., trend and periodicity. Specifically, we adopt the STL approach, i.e., seasonal and trend decomposition using LOESS (locally weighted scatterplot smoothing), to decompose the time series for each location into three components: long-term trend, seasonal periodicity, and the remainder. Events are extracted from the remainder component for each location with an outlier detection method. We analyze over a billion taxi trips for over seven years in Manhattan (New York City) to detect and map urban events at different temporal resolutions. Results show that the approach is effective and robust in detecting events and revealing urban dynamics with both holistic understandings and location-specific interpretations.
更多查看译文
关键词
urban event detection,taxi od trips,big data
AI 理解论文
溯源树
样例
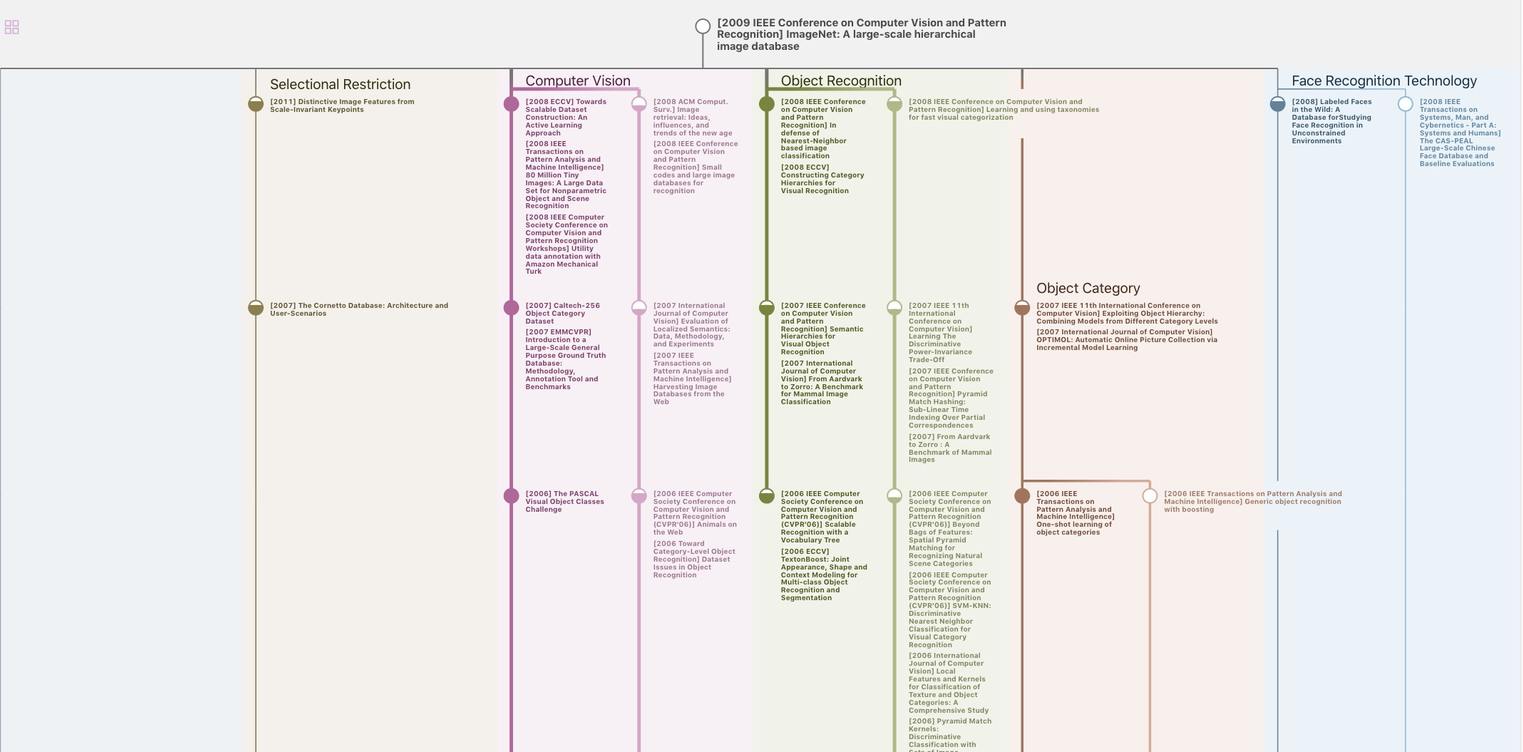
生成溯源树,研究论文发展脉络
Chat Paper
正在生成论文摘要