A machine-learning approach for predicting palmitoylation sites from integrated sequence-based features.
JOURNAL OF BIOINFORMATICS AND COMPUTATIONAL BIOLOGY(2017)
Abstract
Palmitoylation is the covalent attachment of lipids to amino acid residues in proteins. As an important form of protein posttranslational modification, it increases the hydrophobicity of proteins, which contributes to the protein transportation, organelle localization, and functions, therefore plays an important role in a variety of cell biological processes. Identification of palmitoylation sites is necessary for understanding protein-protein interaction, protein stability, and activity. Since conventional experimental techniques to determine palmitoylation sites in proteins are both labor intensive and costly, a fast and accurate computational approach to predict palmitoylation sites from protein sequences is in urgent need. In this study, a support vector machine (SVM)-based method was proposed through integrating PSI-BLAST profile, physicochemical properties, k-mer amino acid compositions (AACs), and k-mer pseudo AACs into the principal feature vector. A recursive feature selection scheme was subsequently implemented to single out the most discriminative features. Finally, an SVM method was implemented to predict palmitoylation sites in proteins based on the optimal features. The proposed method achieved an accuracy of 99.41% and Matthews Correlation Coeffcient of 0.9773 for a benchmark dataset. The result indicates the effciency and accuracy of our method in prediction of palmitoylation sites based on protein sequences.
MoreTranslated text
Key words
Amino acid physicochemical properties,palmitoylation,K-mer amino acid composition,position-specific score matrix,support vector machine,recursive feature elimination
AI Read Science
Must-Reading Tree
Example
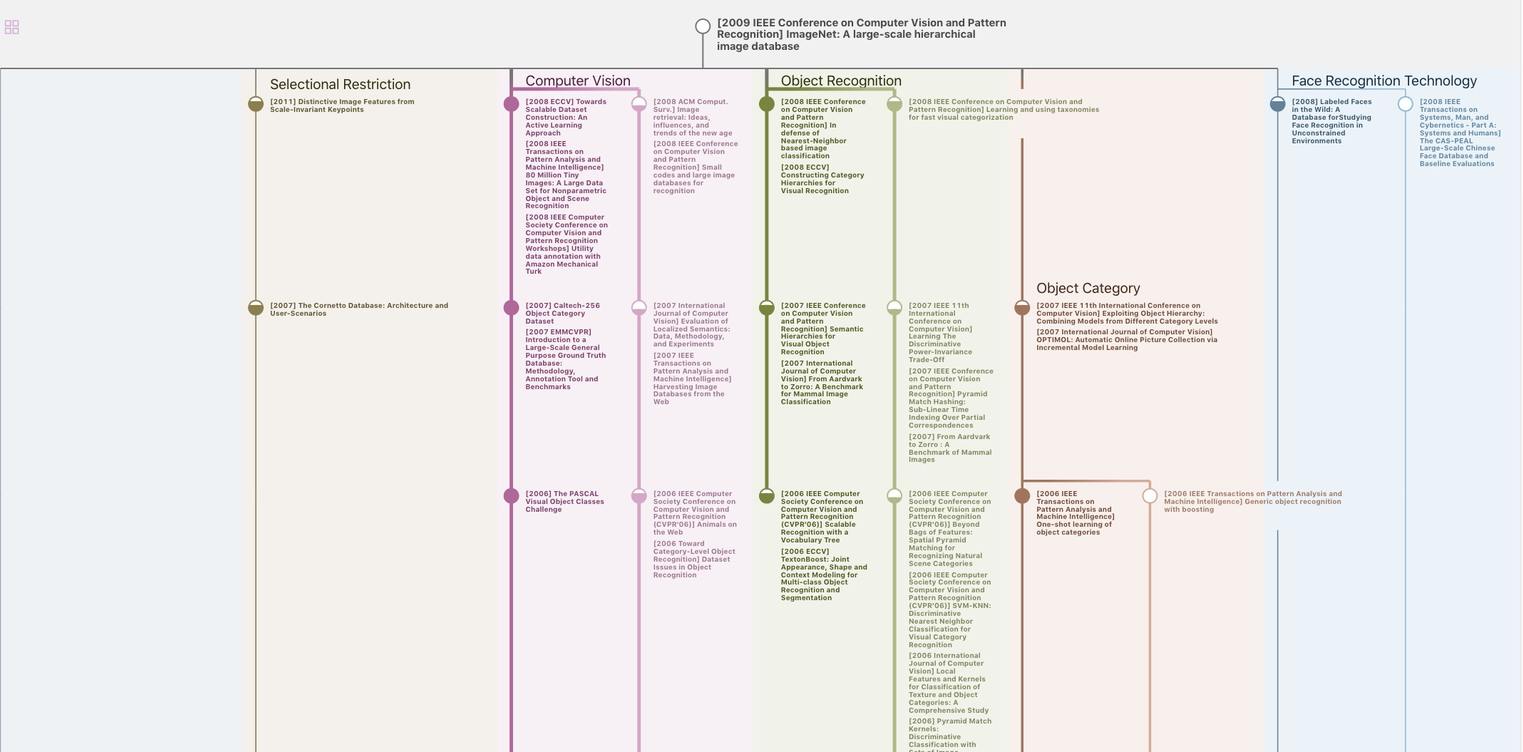
Generate MRT to find the research sequence of this paper
Chat Paper
Summary is being generated by the instructions you defined