A new approach to characterize epileptic seizures using analytic time-frequency flexible wavelet transform and fractal dimension.
Pattern Recognition Letters(2017)
摘要
A new automated model for epileptic seizure detection.The model employs a combination of recently introduced analytic time-frequency flexible wavelet transform and fractal dimension.Eight different classification problems are investigated pertaining to EEG signals.The model surpasses all existing models.Model attains perfect 100% classification sensitivity. The identification of seizure activities in non-stationary electroencephalography (EEG) is a challenging task. The seizure detection by human inspection of EEG signals is prone to errors, inaccurate as well as time-consuming. Several attempts have been made to develop automatic systems so as to assist neurophysiologists in identifying epileptic seizures accurately. The proposed study brings forth a novel automatic approach to detect epileptic seizures using analytic time-frequency flexible wavelet transform (ATFFWT) and fractal dimension (FD). The ATFFWT has inherent attractive features such as, shift-invariance property, tunable oscillatory attribute and flexible time-frequency covering favorable for the analysis of non-stationary and transient signals. We have used ATFFWT to decompose EEG signals into the desired subbands. Following the ATFFWT decomposition, we calculate FD for each subband. Finally, FDs of all subbands have been fed to the least-squares support vector machine (LS-SVM) classifier. The 10-fold cross validation has been used to obtain stable and reliable performance and to avoid the over fitting of the model. In this study, we investigate various different classification problems (CPs) pertaining to different classes of EEG signals, including the following popular CPs: (i) ictal versus normal (ii) ictal versus inter-ictal (iii) ictal versus non-ictal. The proposed model is found to be outperforming all existing models in terms of classification sensitivity (CSE) as it achieves perfect 100% sensitivity for seven CPs investigated by us. The prominent attribute of the proposed system is that though the model employs only one set of discriminating features (FD) for all CPs, it yields promising classification accuracy. Since, the proposed model attains the perfect classification performance it appears that a system is in place to assist clinicians to diagnose seizures accurately in less time. Further, the proposed system seems useful and attractive, especially, in the rural areas of developing countries where there is a shortage of experienced clinicians and expensive machines like functional magnetic resonance imaging (fMRI).
更多查看译文
关键词
Electroencephalography,EEG,Epilepsy,Seizure,Analytic time-frequency flexible wavelet transforms,Fractal dimension
AI 理解论文
溯源树
样例
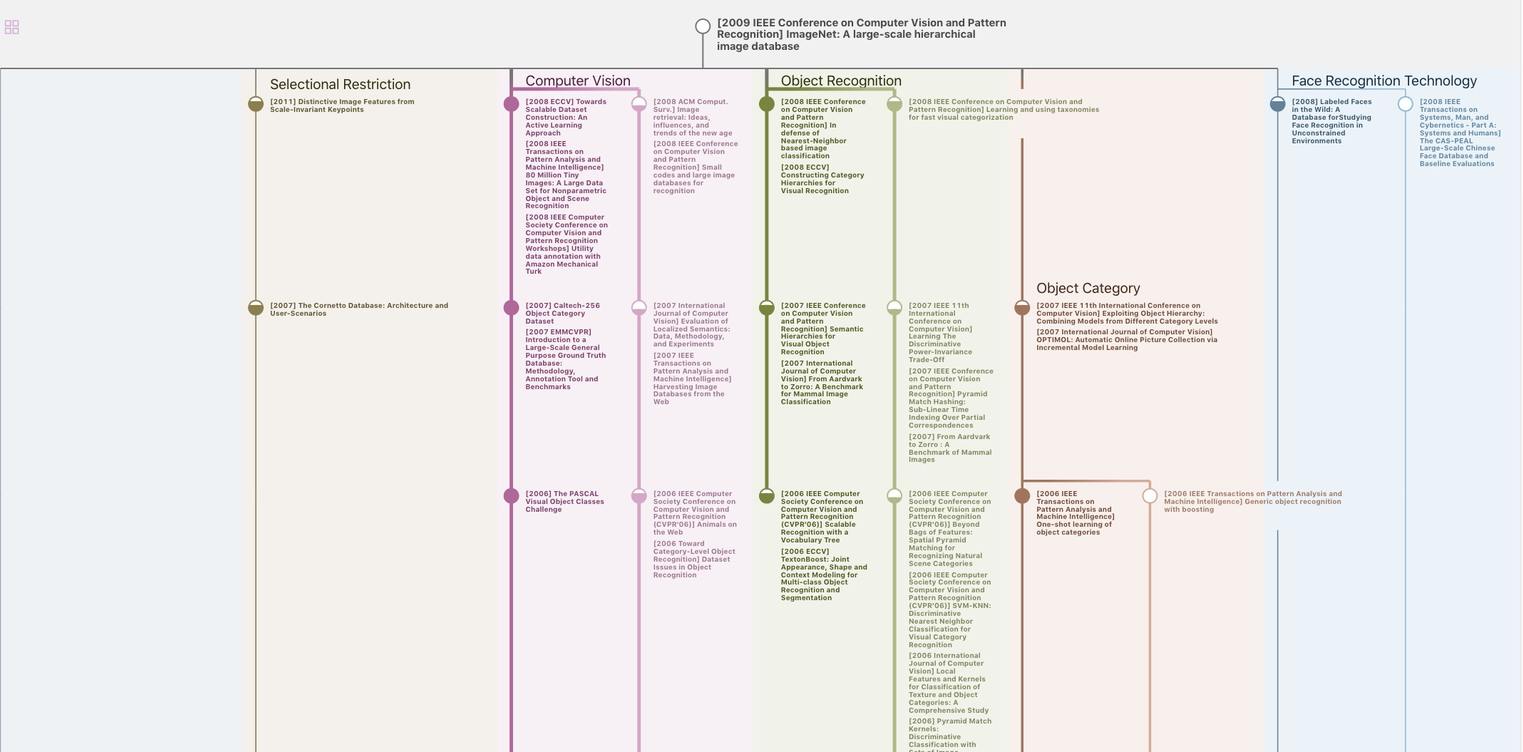
生成溯源树,研究论文发展脉络
Chat Paper
正在生成论文摘要