Evolving connectionist method for adaptive audiovisual speech recognition
Evolving Systems(2016)
摘要
Reliability is the primary requirement in noisy conditions and for highly variable utterances. Integrating the recognition of visual signals with the recognition of audio signals is indispensable for many applications that require automatic speech recognition (ASR) in harsh conditions. Several important experiments have shown that integrating and adapting to multiple behavioral end-context information during the speech-recognition task significantly improves its success rate. By integrating audio and visual data from speech information, we can improve the performance of an ASR system by differentiating between the most critical cases of phonetic-unit mismatch that occur when processing audio or visual input alone. The evolving fuzzy neural-network (EFuNN) inference method is applied at the decision layer to accomplish this task. This is done through a paradigm that adapts to the environment by changing structure. The EFuNN’s capacity to learn quickly from incoming data and to adapt while on line lowers the ASR system’s complexity and enhances its performance in harsh conditions. Two independent feature extractors were developed, one for speech phonetics (listening to the speech) and the other for speech visemics (lip-reading the spoken input). The EFuNN network was trained to fuse decisions made disjointly by the audio unit and the visual unit. Our experiments have confirmed that the proposed method is reliable for developing a robust, automatic, speech-recognition system.
更多查看译文
关键词
Audiovisual speech recognition (AVSR), Evolving fuzzy neural network (EFuNN), Speech-to-text (STT), Decision fusion, Multimodal speech recognition
AI 理解论文
溯源树
样例
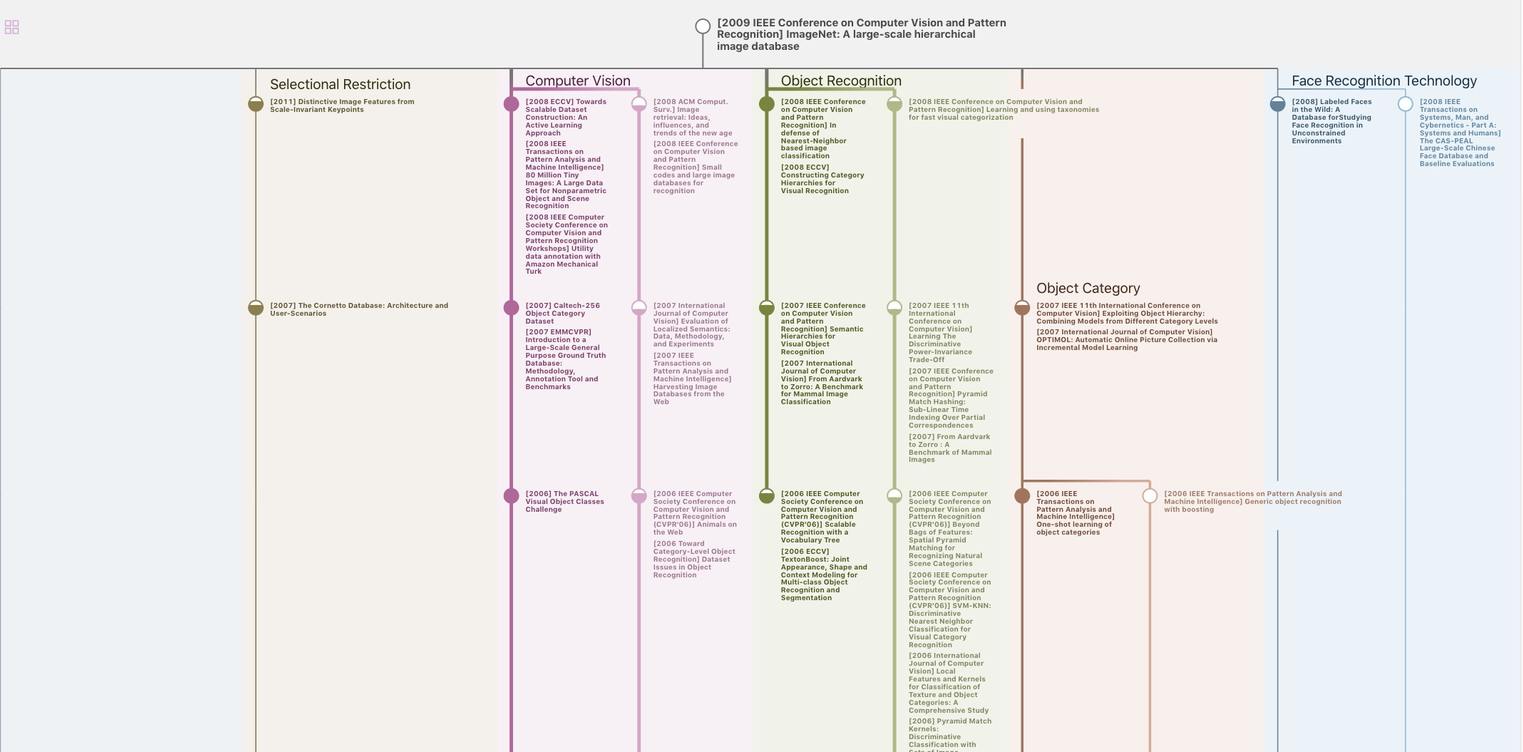
生成溯源树,研究论文发展脉络
Chat Paper
正在生成论文摘要