Proper Learning of k-term DNF Formulas from Satisfying Assignments.
Electronic Colloquium on Computational Complexity (ECCC)(2017)
摘要
In certain applications there may be only positive examples available to learn concepts of a class of interest. Furthermore, learning has to be done properly, i.e. the hypothesis space has to coincide with the concept class, and without false positives, i.e. the hypothesis always has to be a subset of the real concept (one-sided error). For the well studied class of k-term DNF formulas it has been known that learning is difficult. Unless RP = NP, it is not feasible to learn k-term DNF formulas properly in a distribution-free sense even if both positive and negative examples are available and even if false positives are allowed.
更多查看译文
关键词
Algorithmic learning,Learning from positive examples,q-bounded distributions,k-term DNF formulas
AI 理解论文
溯源树
样例
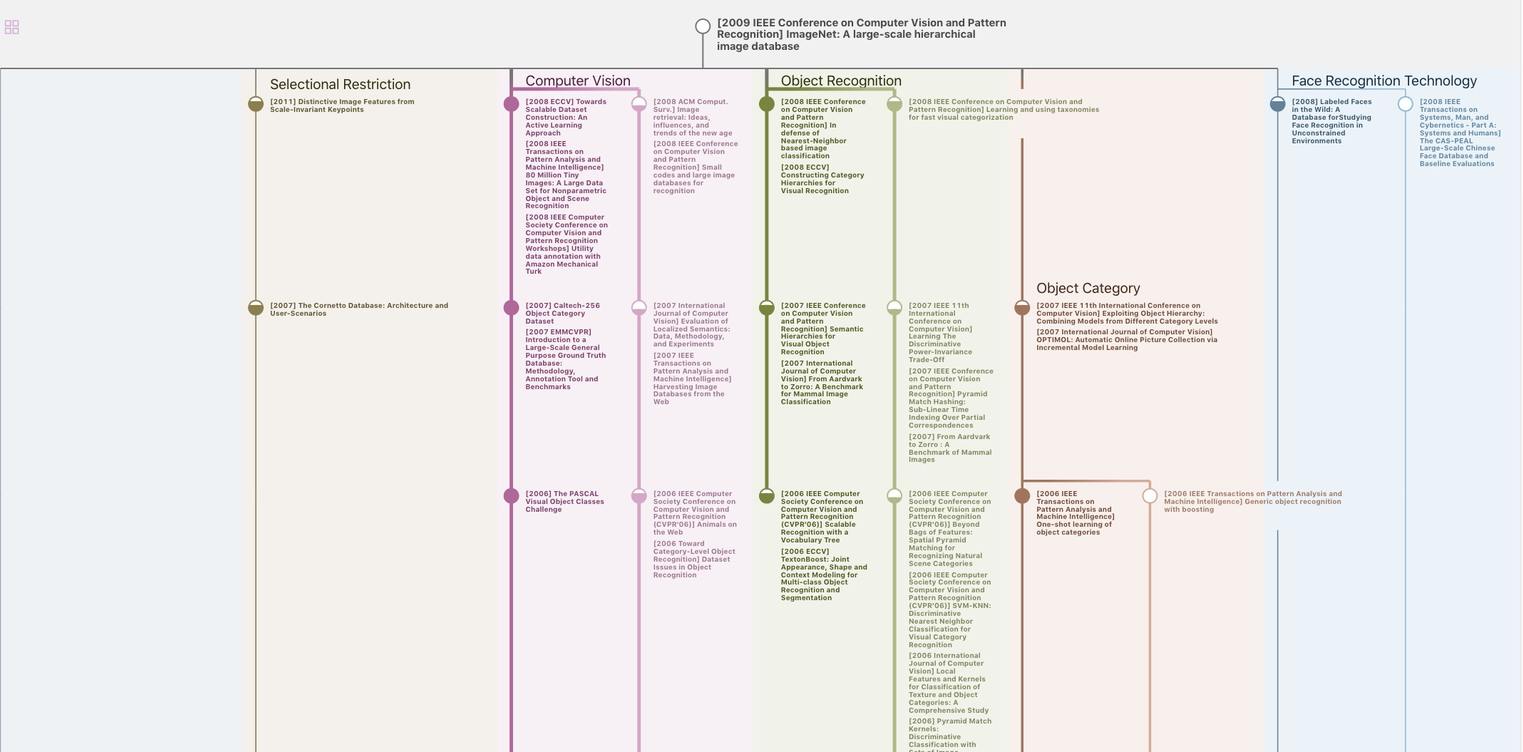
生成溯源树,研究论文发展脉络
Chat Paper
正在生成论文摘要