Multilevel Integration Entropies: The Case Of Reconstruction Of Structural Quasi-Stability In Building Complex Datasets
ENTROPY(2017)
Abstract
The emergence of complex datasets permeates versatile research disciplines leading to the necessity to develop methods for tackling complexity through finding the patterns inherent in datasets. The challenge lies in transforming the extracted patterns into pragmatic knowledge. In this paper, new information entropy measures for the characterization of the multidimensional structure extracted from complex datasets are proposed, complementing the conventionally-applied algebraic topology methods. Derived from topological relationships embedded in datasets, multilevel entropy measures are used to track transitions in building the high dimensional structure of datasets captured by the stratified partition of a simplicial complex. The proposed entropies are found suitable for defining and operationalizing the intuitive notions of structural relationships in a cumulative experience of a taxi driver's cognitive map formed by origins and destinations. The comparison of multilevel integration entropies calculated after each new added ride to the data structure indicates slowing the pace of change over time in the origin-destination structure. The repetitiveness in taxi driver rides, and the stability of origin-destination structure, exhibits the relative invariance of rides in space and time. These results shed light on taxi driver's ride habits, as well as on the commuting of persons whom he/she drove.
MoreTranslated text
Key words
integration entropy,information,topological data analysis,Q-analysis,high dimensional data,urban dynamics
AI Read Science
Must-Reading Tree
Example
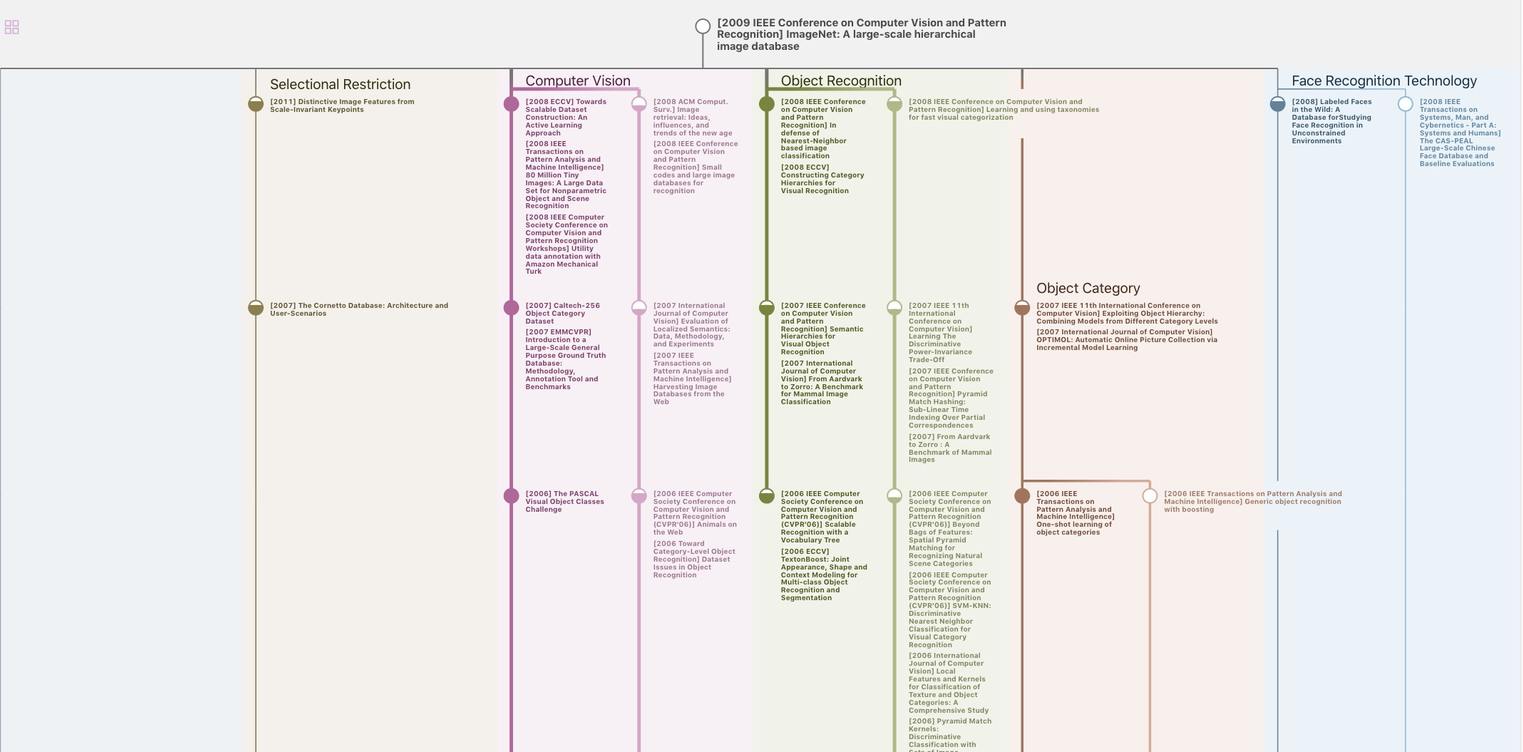
Generate MRT to find the research sequence of this paper
Chat Paper
Summary is being generated by the instructions you defined