Adversarial Examples for Semantic Segmentation and Object Detection
2017 IEEE International Conference on Computer Vision (ICCV)(2017)
摘要
It has been well demonstrated that adversarial examples, i.e., natural images with visually imperceptible perturbations added, generally exist for deep networks to fail on image classification. In this paper, we extend adversarial examples to semantic segmentation and object detection which are much more difficult. Our observation is that both segmentation and detection are based on classifying multiple targets on an image (e.g., the basic target is a pixel or a receptive field in segmentation, and an object proposal in detection), which inspires us to optimize a loss function over a set of pixels/proposals for generating adversarial perturbations. Based on this idea, we propose a novel algorithm named Dense Adversary Generation (DAG), which generates a large family of adversarial examples, and applies to a wide range of state-of-the-art deep networks for segmentation and detection. We also find that the adversarial perturbations can be transferred across networks with different training data, based on different architectures, and even for different recognition tasks. In particular, the transferability across networks with the same architecture is more significant than in other cases. Besides, summing up heterogeneous perturbations often leads to better transfer performance, which provides an effective method of black-box adversarial attack.
更多查看译文
关键词
dense adversary generation,black-box adversarial attack,adversarial perturbations,image classification,deep networks,visually imperceptible perturbations,natural images,object detection,semantic segmentation
AI 理解论文
溯源树
样例
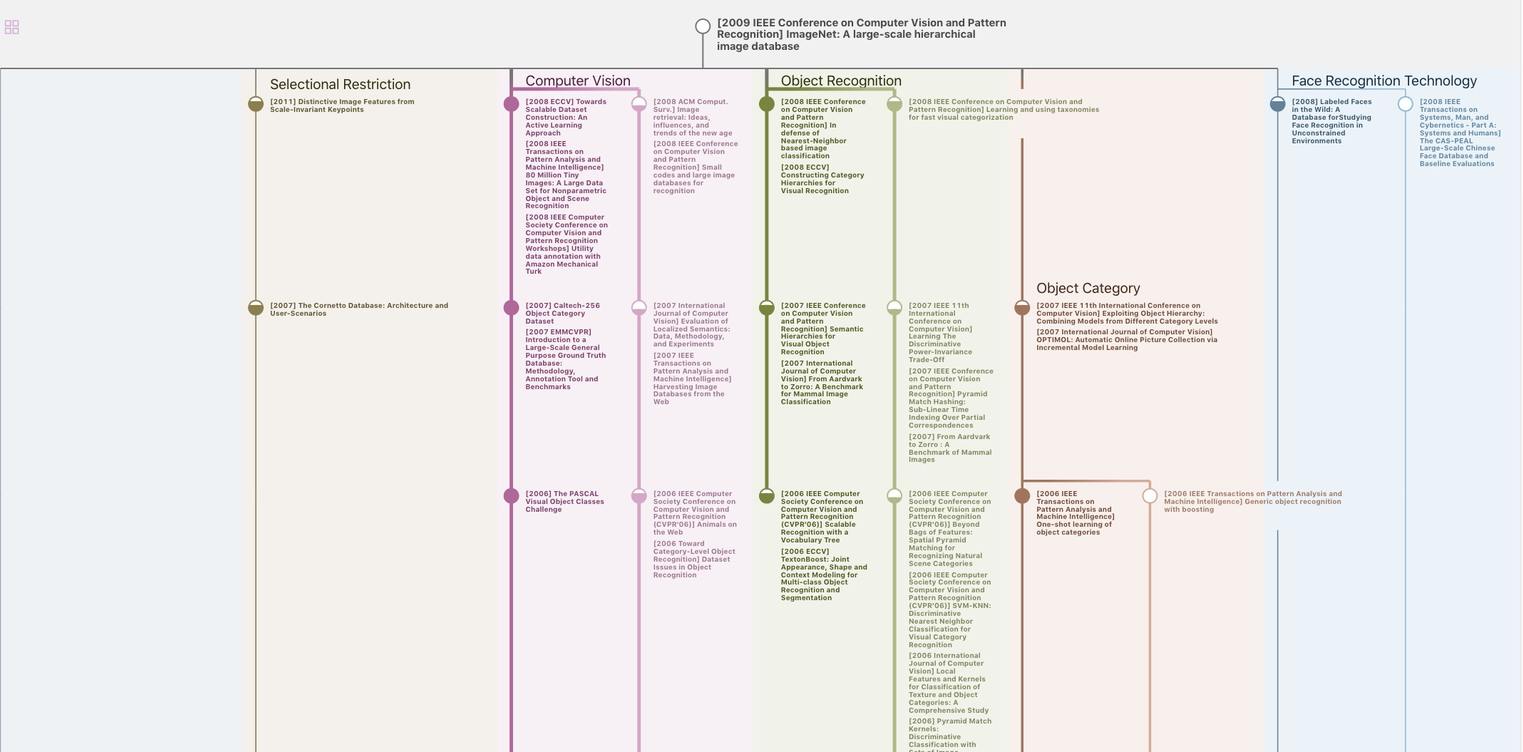
生成溯源树,研究论文发展脉络
Chat Paper
正在生成论文摘要