Adversarially Regularized Autoencoders for Generating Discrete Structures.
arXiv: Learning(2017)
摘要
Generative adversarial networks are an effective approach for learning rich latent representations of continuous data, but have proven difficult to apply directly to discrete structured data, such as text sequences or discretized images. Ideally we could encode discrete structures in a continuous code space to avoid this problem, but it is difficult to learn an appropriate general-purpose encoder. In this work, we consider a simple approach for handling these two challenges jointly, employing a discrete structure autoencoder with a code space regularized by generative adversarial training. The model learns a smooth regularized code space while still being able to model the underlying data, and can be used as a discrete GAN with the ability to generate coherent discrete outputs from continuous samples. We demonstrate empirically how key properties of the data are captured in the modelu0027s latent space, and evaluate the model itself on the tasks of discrete image generation, text generation, and semi-supervised learning.
更多查看译文
AI 理解论文
溯源树
样例
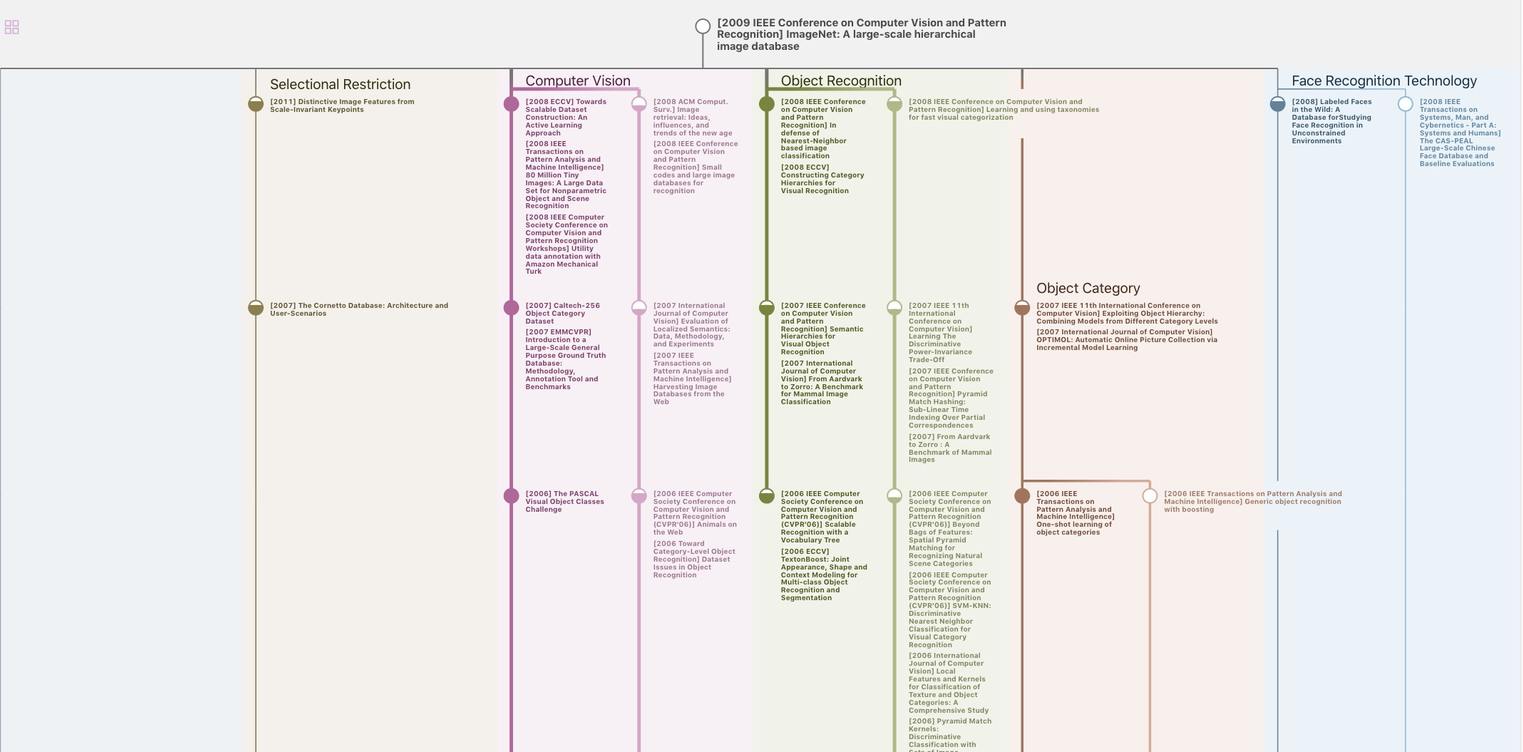
生成溯源树,研究论文发展脉络
Chat Paper
正在生成论文摘要