Socially Aware Motion Planning with Deep Reinforcement Learning
2017 IEEE/RSJ International Conference on Intelligent Robots and Systems (IROS)(2018)
摘要
For robotic vehicles to navigate safely and efficiently in pedestrian-rich environments, it is important to model subtle human behaviors and navigation rules (e.g., passing on the right). However, while instinctive to humans, socially compliant navigation is still difficult to quantify due to the stochasticity in people's behaviors. Existing works are mostly focused on using feature-matching techniques to describe and imitate human paths, but often do not generalize well since the feature values can vary from person to person, and even run to run. This work notes that while it is challenging to directly specify the details of what to do (precise mechanisms of human navigation), it is straightforward to specify what not to do (violations of social norms). Specifically, using deep reinforcement learning, this work develops a time-efficient navigation policy that respects common social norms. The proposed method is shown to enable fully autonomous navigation of a robotic vehicle moving at human walking speed in an environment with many pedestrians.
更多查看译文
关键词
deep reinforcement learning,pedestrian-rich environments,navigation rules,human navigation,time-efficient navigation policy,fully autonomous navigation,human walking speed,human behaviors,social norms,socially aware motion planning,robotic vehicle navigation,socially compliant navigation
AI 理解论文
溯源树
样例
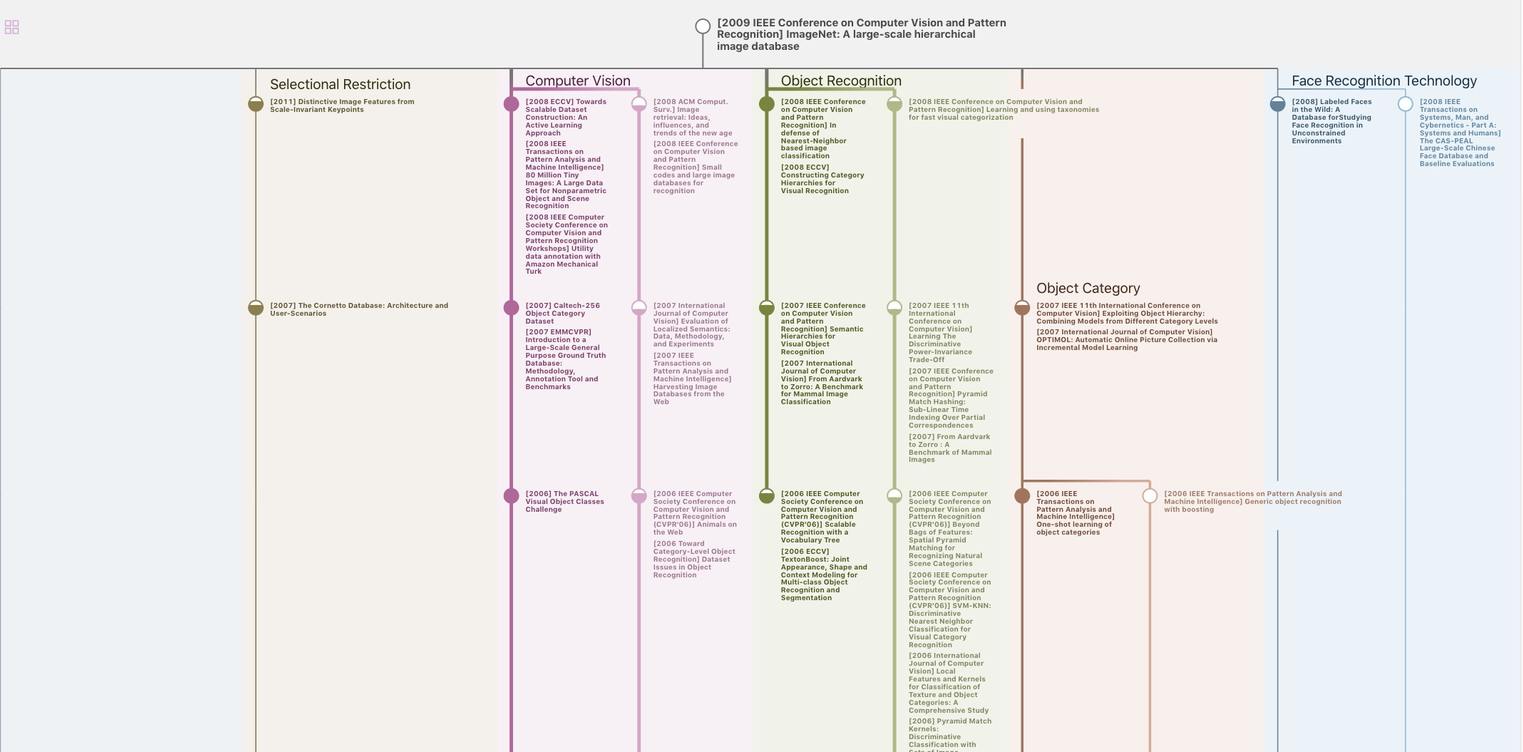
生成溯源树,研究论文发展脉络
Chat Paper
正在生成论文摘要