Empirical Evaluation Of Parallel Training Algorithms On Acoustic Modeling
18TH ANNUAL CONFERENCE OF THE INTERNATIONAL SPEECH COMMUNICATION ASSOCIATION (INTERSPEECH 2017), VOLS 1-6: SITUATED INTERACTION(2017)
摘要
Deep learning models (DLMs) are state-of-the-art techniques in speech recognition. However, training good DLMs can be time consuming especially for production-size models and corpora. Although several parallel training algorithms have been proposed to improve training efficiency, there is no clear guidance on which one to choose for the task in hand due to lack of systematic and fair comparison among them. In this paper we aim at filling this gap by comparing four popular parallel training algorithms in speech recognition, namely asynchronous stochastic gradient descent (ASGD). blockwise model-update filtering (BMUF), bulk synchronous parallel (BSP) and elastic averaging stochastic gradient descent (EASGD), on 1000-hour LibriSpeech corpora using feed-forward deep neural networks (DNNs) and convolutional, long short-term memory, DNNs (CLDNNs). Based on our experiments, we recommend using BMUF as the top choice to train acoustic models since it is most stable, scales well with number of GPUs, can achieve reproducible results. and in many cases even outperforms single-GPU SGD. ASGD can be used as a substitute in some cases.
更多查看译文
关键词
speech recognition, parallel algorithm, ASGD, BMUF, BSP, EASGD
AI 理解论文
溯源树
样例
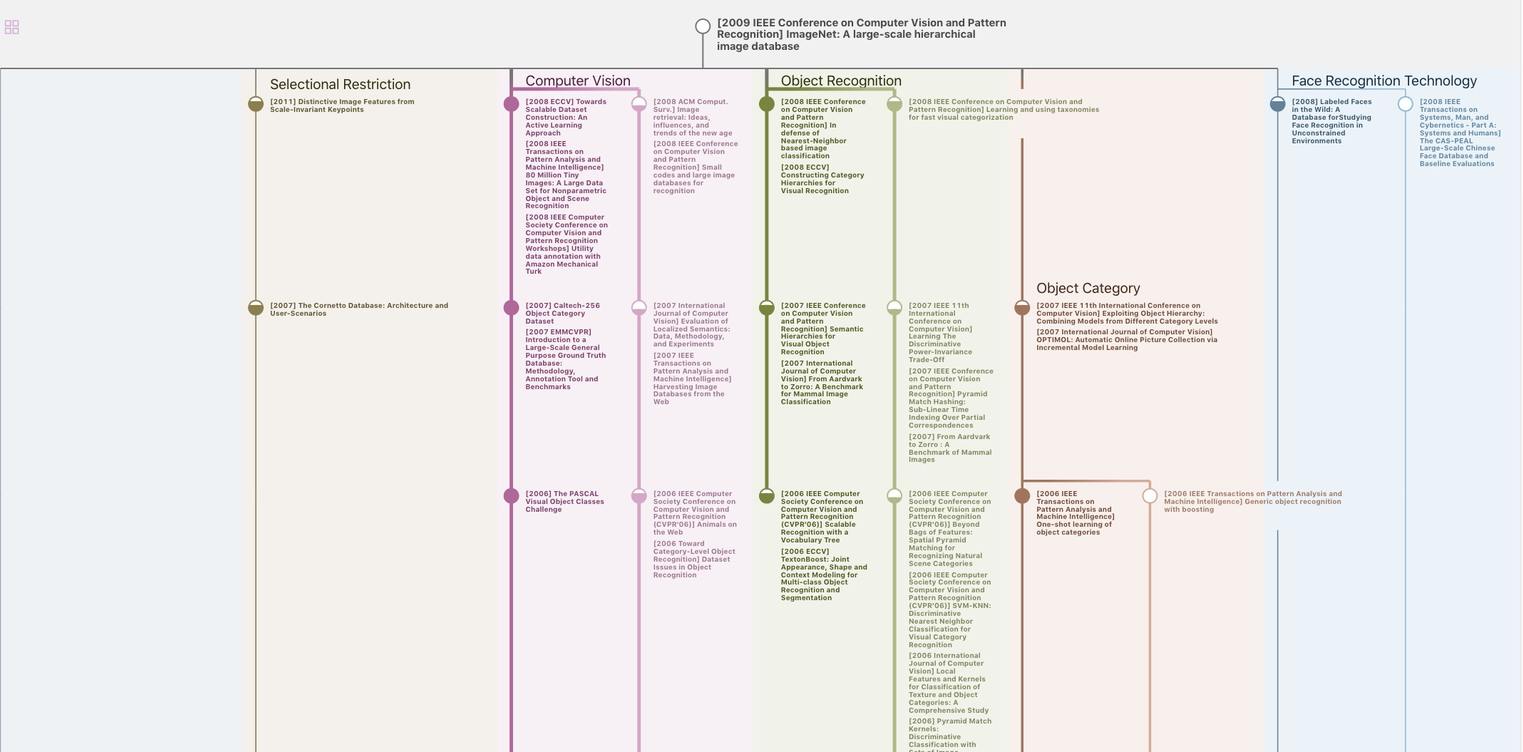
生成溯源树,研究论文发展脉络
Chat Paper
正在生成论文摘要