Extension Of Canonical Adjacency Matrices For Frequent Approximate Subgraph Mining On Multi-Graph Collections
INTERNATIONAL JOURNAL OF PATTERN RECOGNITION AND ARTIFICIAL INTELLIGENCE(2017)
摘要
Into the data mining field, frequent approximate subgraph (FAS) mining has become an important technique with a broad spectrum of real-life applications. This fact is because several real-life phenomena can be modeled by graphs. In the literature, several algorithms have been reported for mining frequent approximate patterns on simple-graph collections; however, there are applications where more complex data structures, as multi-graphs, are needed for modeling the problem. But to the best of our knowledge, there is no FAS mining algorithm designed for dealing with multi-graphs. Therefore, in this paper, a canonical form (CF) for simple-graphs is extended to allow representing multi-graphs and a state-of-the-art algorithm for FAS mining is also extended for processing multi-graph collections by using the extended CF. Our experiments over different synthetic and real-world multi-graph collections show that the proposed algorithm has a good performance in terms of runtime and scalability. Additionally, we show the usefulness of the patterns computed by our algorithm in an image classiffication problem where images are represented as multi-graphs.
更多查看译文
关键词
Approximate multi-graph mining, frequent approximate subgraphs, canonical forms, canonical adjacency matrix
AI 理解论文
溯源树
样例
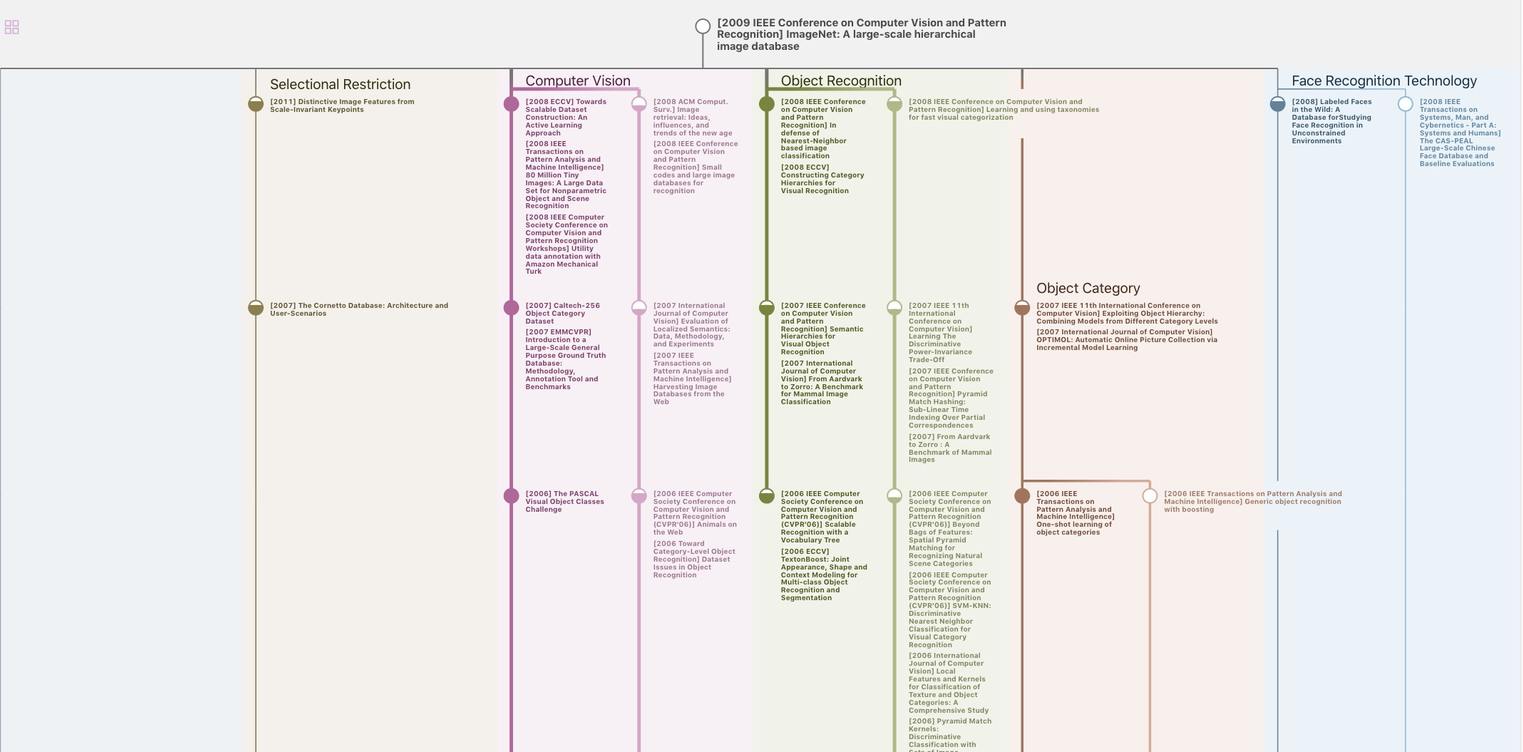
生成溯源树,研究论文发展脉络
Chat Paper
正在生成论文摘要