Multiple-View Active Learning For Environmental Sound Classification
INTERNATIONAL JOURNAL OF ONLINE ENGINEERING(2016)
摘要
Multi-view learning with multiple distinct feature sets is a rapid growing direction in machine learning with boosting the performance of supervised learning classification under the case of few labeled (1)data. The paper proposes Multi-view Simple Disagreement Sampling (MVSDS) and Multi-view Entropy Priority Sampling (MV-EPS) methods as the selecting samples strategies in active learning with multiple-view. For the given environmental sound data, the CELP features in 10 dimensions and the MFCC features in 13 dimensions are two views respectively. The experiments with a single view single classifier, SVML, MVSDS and MV-EPS on the environmental sound extracted two of views, CELP & MFCC are carried out to illustrate the results of the proposed methods and their performances are compared under different percent training examples. The experimental results show that multi-view active learning can effectively improve the performance of classification for environmental sound data, and MV-EPS method outperforms the MV-SDS.
更多查看译文
关键词
Active learning, Multiple-view learning, MV-SDS, MV-EPS
AI 理解论文
溯源树
样例
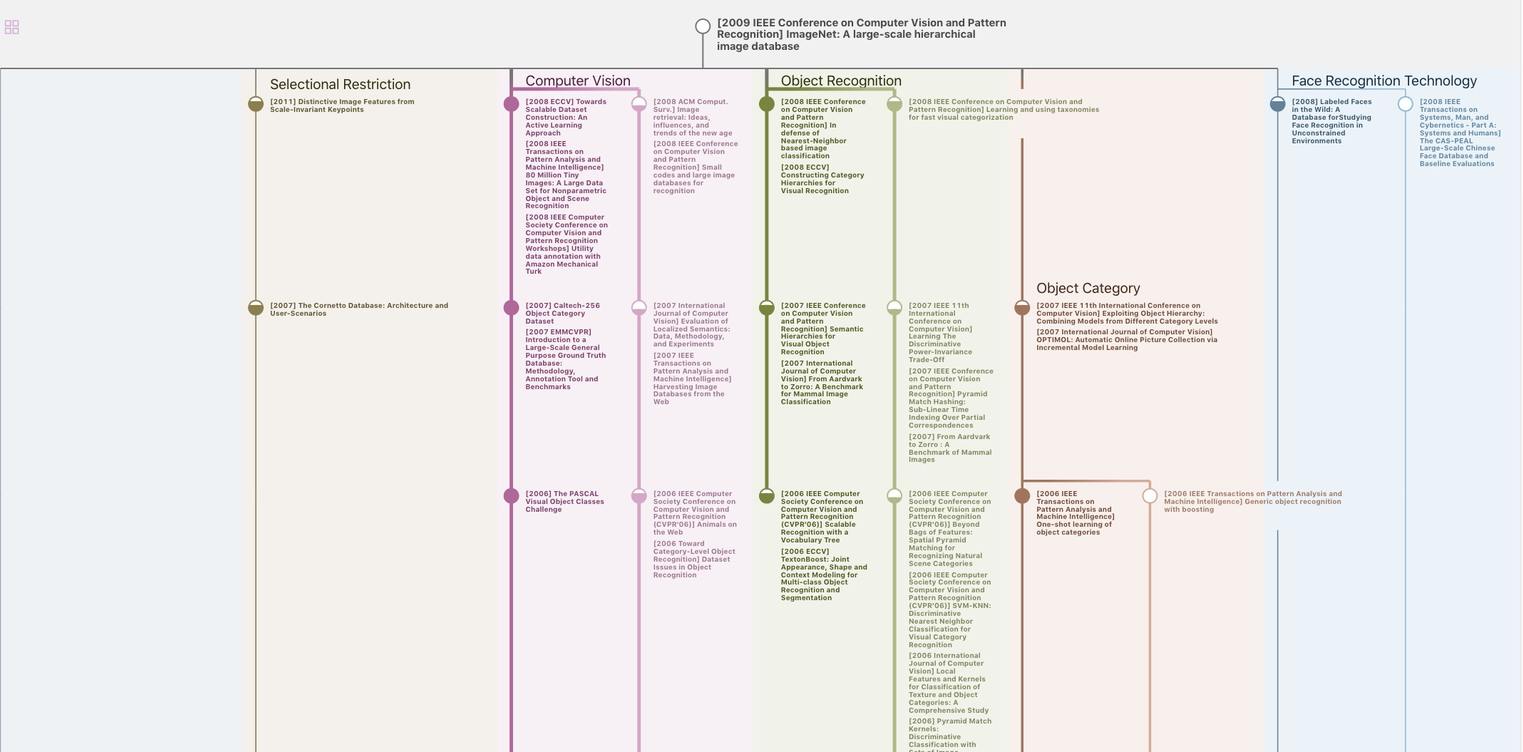
生成溯源树,研究论文发展脉络
Chat Paper
正在生成论文摘要