Handling Imbalanced Classification Problem: A Case Study On Social Media Datasets
JOURNAL OF INTELLIGENT & FUZZY SYSTEMS(2017)
摘要
The imbalanced data problem occurs when the number of representative instances for classes of interest is much lower than for other classes. The influence of imbalanced data on classification performance has been discussed in some previous research as a challenge to be studied. In this paper, we propose a method to solve the imbalanced data problem by focusing on preprocessing, including: i) sampling techniques (i.e., under-sampling, over-sampling, and hybrid-sampling) and ii) the instance weighting method to increase the number of features in minority classes and to reduce comprehensive coverage in majority classes. The experimental results show that the noisy data is reduced, making a smaller sized dataset, and training time decreases significantly. Moreover, distinct properties of each class are examined effectively. Refined data is used as input for Naive Bayes and support vector machine classifiers for the targets of the training process. The proposed methods are evaluated based on the number of non-geotagged resources that are labeled correctly with their geo-locations. In comparison with previous research, the proposed method achieves accuracy of 84%, whereas previous results were 75%.
更多查看译文
关键词
Imbalanced datasets,geotags resources,sampling method,instance weighting,location prediction
AI 理解论文
溯源树
样例
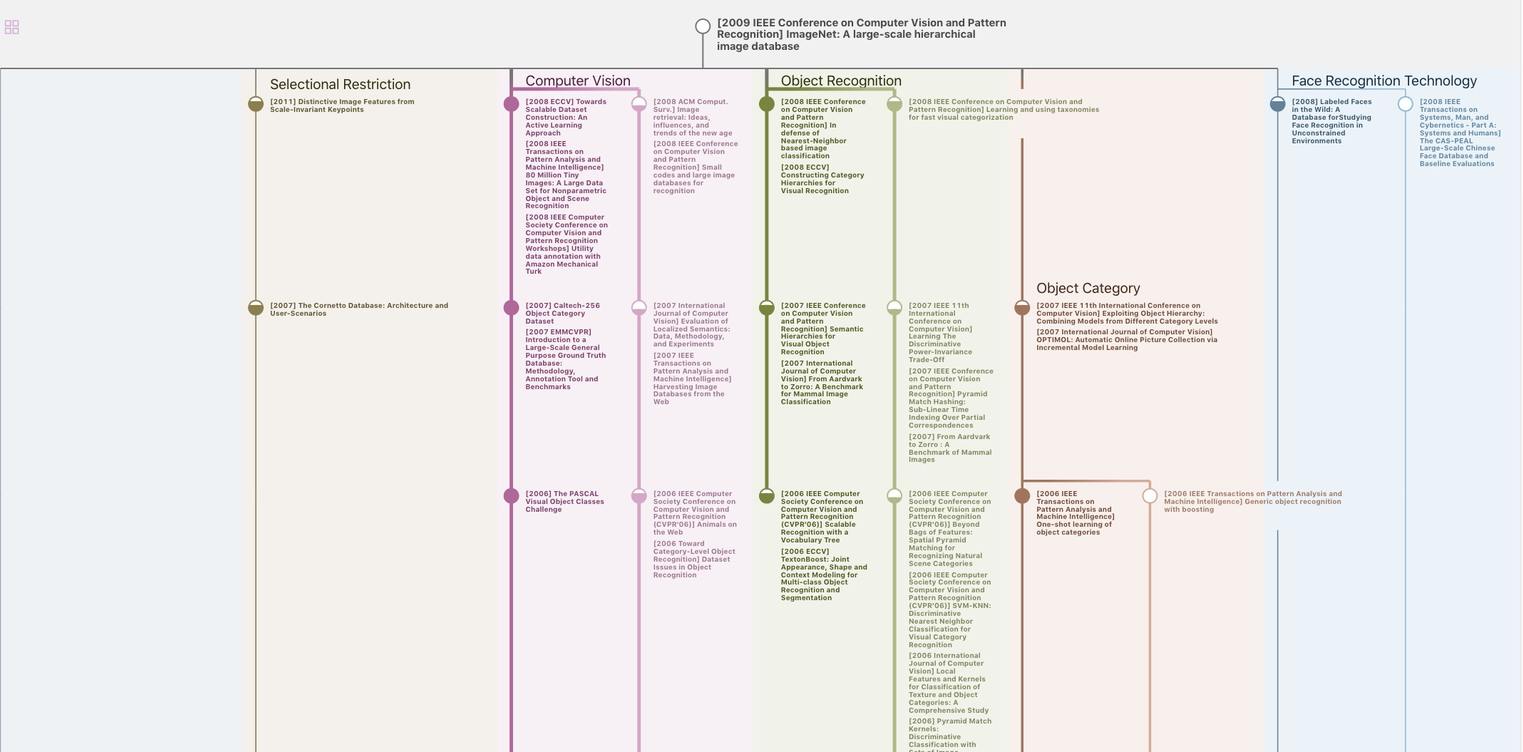
生成溯源树,研究论文发展脉络
Chat Paper
正在生成论文摘要