Learning User Attributes via Mobile Social Multimedia Analytics.
ACM TIST(2017)
摘要
Learning user attributes from mobile social media is a fundamental basis for many applications, such as personalized and targeting services. A large and growing body of literature has investigated the user attributes learning problem. However, far too little attention has been paid to jointly consider the dual heterogeneities of user attributes learning by harvesting multiple social media sources. In particular, user attributes are complementarily and comprehensively characterized by multiple social media sources, including footprints from Foursqare, daily updates from Twitter, professional careers from Linkedin, and photo posts from Instagram. On the other hand, attributes are inter-correlated in a complex way rather than independent to each other, and highly related attributes may share similar feature sets. Towards this end, we proposed a unified model to jointly regularize the source consistency and graph-constrained relatedness among tasks. As a byproduct, it is able to learn the attribute-specific and attribute-sharing features via graph-guided fused lasso penalty. Besides, we have theoretically demonstrated its optimization. Extensive evaluations on a real-world dataset thoroughly demonstrated the effectiveness of our proposed model.
更多查看译文
关键词
Algorithms,Experimentation,Performance,Learning user attributes,mobile social multimedia analytic,occupation inference
AI 理解论文
溯源树
样例
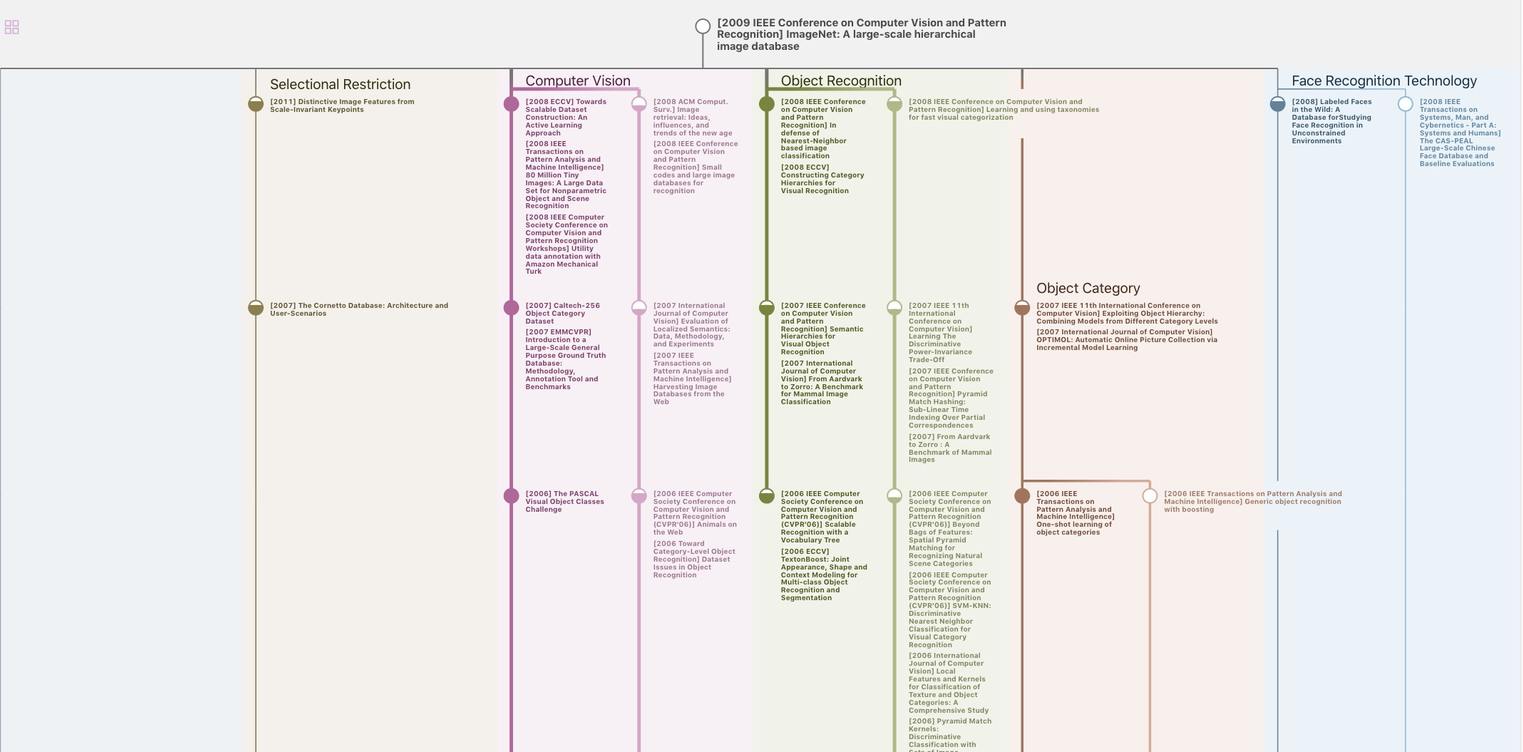
生成溯源树,研究论文发展脉络
Chat Paper
正在生成论文摘要