Multi-view clustering via multi-manifold regularized non-negative matrix factorization.
Neural Networks(2017)
摘要
Non-negative matrix factorization based multi-view clustering algorithms have shown their competitiveness among different multi-view clustering algorithms. However, non-negative matrix factorization fails to preserve the locally geometrical structure of the data space. In this paper, we propose a multi-manifold regularized non-negative matrix factorization framework (MMNMF) which can preserve the locally geometrical structure of the manifolds for multi-view clustering. MMNMF incorporates consensus manifold and consensus coefficient matrix with multi-manifold regularization to preserve the locally geometrical structure of the multi-view data space. We use two methods to construct the consensus manifold and two methods to find the consensus coefficient matrix, which leads to four instances of the framework. Experimental results show that the proposed algorithms outperform existing non-negative matrix factorization based algorithms for multi-view clustering.
更多查看译文
关键词
Non-negative matrix factorization,Multi-view clustering,Multi-manifold,Locally linear embedding (LLE)
AI 理解论文
溯源树
样例
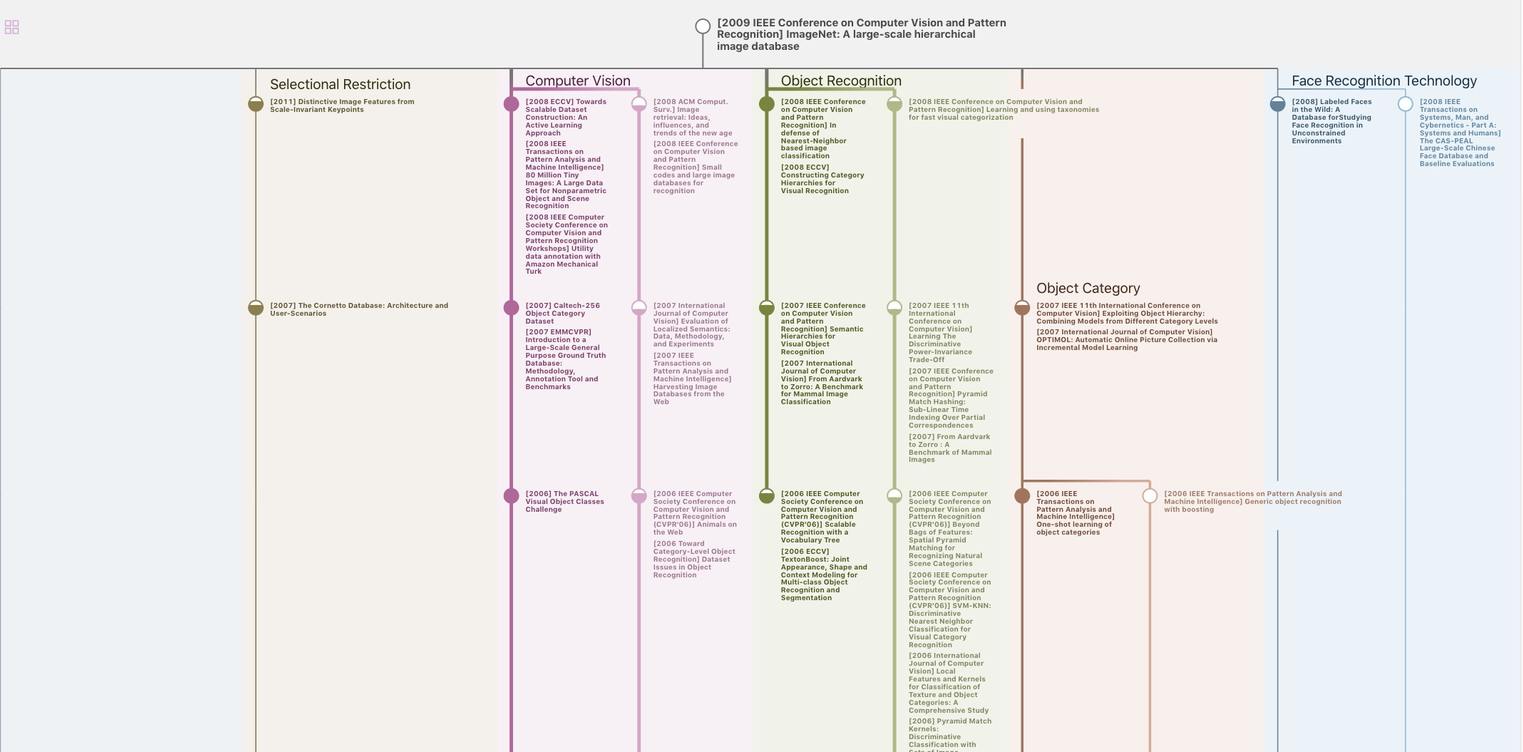
生成溯源树,研究论文发展脉络
Chat Paper
正在生成论文摘要