Approximate Message Passing for Structured Affine Rank Minimization Problem.
IEEE ACCESS(2017)
摘要
The topic of the rank minimization problem with affine constraints has been well studied in recent years. However, in many applications the data can exhibit other structures beyond simply being low rank. For example, images and videos present complex spatio-temporal structures, which are largely ignored by current affine rank minimization (ARM) methods. In this paper, we propose a novel approximate message passing (AMP)-based approach that is capable of capturing additional structures in the matrix entries, and can be implemented in a wide range of applications with little or no modification. Using probabilistic low-rank factorization, we derive our generalized AMP-based algorithm as an approximation of the loopy belief propagation algorithm. In addition, we apply a rank selection strategy and an expectation-maximization estimation strategy that adaptively obtain the optimal value of the algorithmic parameters. Then, we discuss the specializations of our proposed algorithm to the applications of structured ARM problems, such as compressive hyperspectral imaging and compressive video surveillance. Simulation results with both synthetic and real data demonstrate that the proposed algorithm yields the state-of-the-art reconstruction performance while maintaining competitive computational complexity.
更多查看译文
关键词
Affine rank minimization (ARM),structured low-rank matrix recovery,approximate message passing (AMP),belief propagation,graphical models,sum-product algorithm (SPA)
AI 理解论文
溯源树
样例
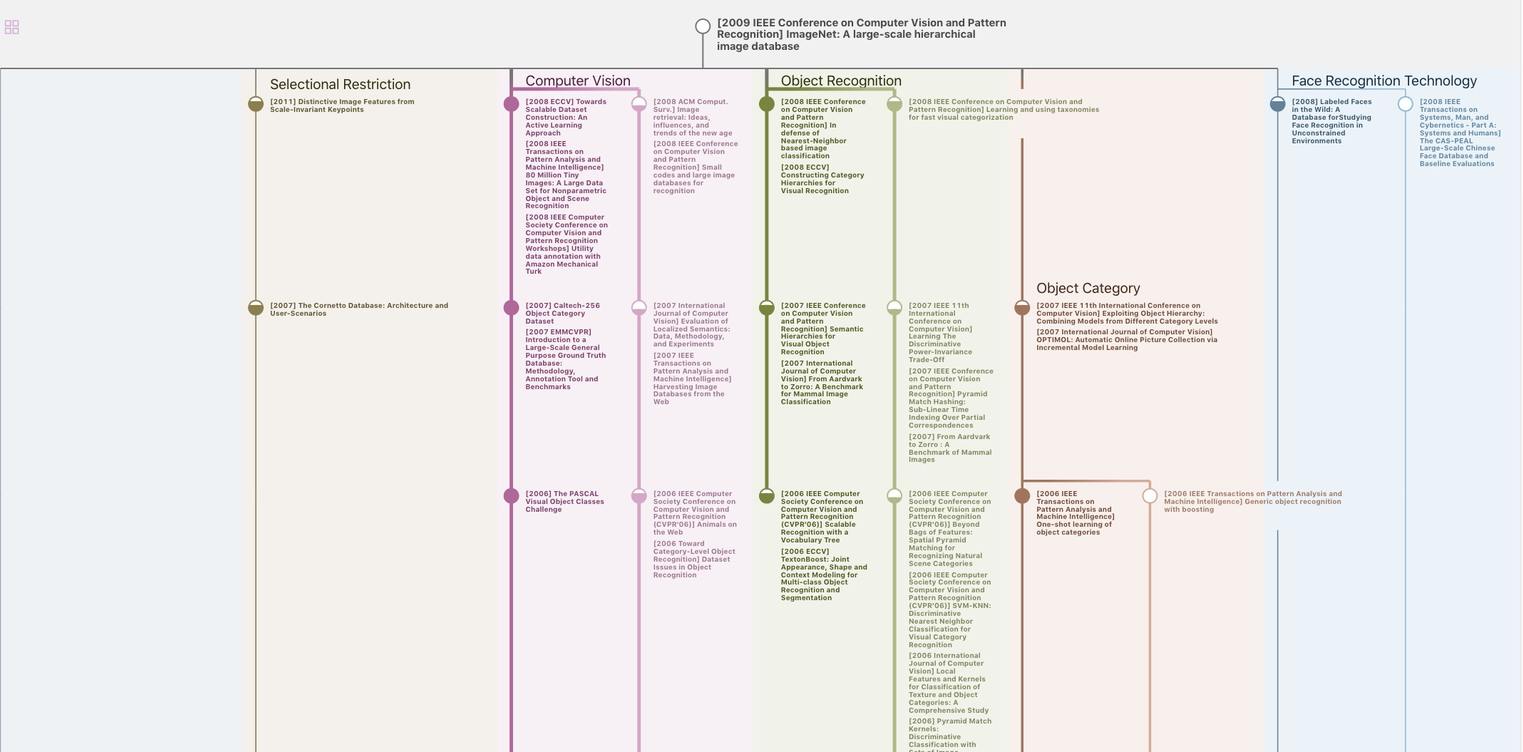
生成溯源树,研究论文发展脉络
Chat Paper
正在生成论文摘要