Spike-time encoding as a data compression technique for pattern recognition of temporal data.
Inf. Sci.(2017)
摘要
The human brains ability to efficiently detect patterns from the continuous streaming sensory stimuli has been a source of constant intrigue for naturalists, and has set the course for the development of research into artificial intelligence. Efficient encoding of such input stimuli into discrete timing of events play a decisive role in the ability of the spiking neurons inside the human brain to compress, transmit and recognise information presented by the external environment. In this article, we introduce the spike-time or temporal encoding paradigm as an efficient general approach to data compression for the purpose of pattern recognition. The data compression through spike-time encoding not only dramatically reduces the volume of data required to capture discriminatory information leading to economical storage and transmission, but can also be used for pattern recognition in streaming data domain. We experimentally show that the spike-time data produced by the temporal encoding techniques achieve comparable (superior in some cases) performance of pattern recognition in comparison to the use of the whole raw data. This article also introduces a generalised background knowledge driven optimisation based temporal encoding framework for encoding time series data and as an illustration of this approach, further formulates a temporal encoding algorithm, namely GAGamma, designed to efficiently compress fMRI data using discrete spike-times. We have evaluated the temporal encoding algorithms on the benchmark Starplus fMRI dataset, and the results demonstrate the temporal encoding algorithms ability to achieve significant data compression without sacrificing the performance of the pattern recognition in the compressed space. We also show that the GAGamma algorithm provides enhanced flexibility and control (compared to the state of the art temporal encoding algorithms) within the design framework of the temporal encoding problem for fMRI, leading to better quality of spike-time data. The proposed approach, by the use of efficient brain-like encoding mechanism, opens up new possibilities in information compression, communication and pattern recognition and thus is applicable to a range of new applications.
更多查看译文
关键词
Spike-time encoding,Data compression,Pattern recognition
AI 理解论文
溯源树
样例
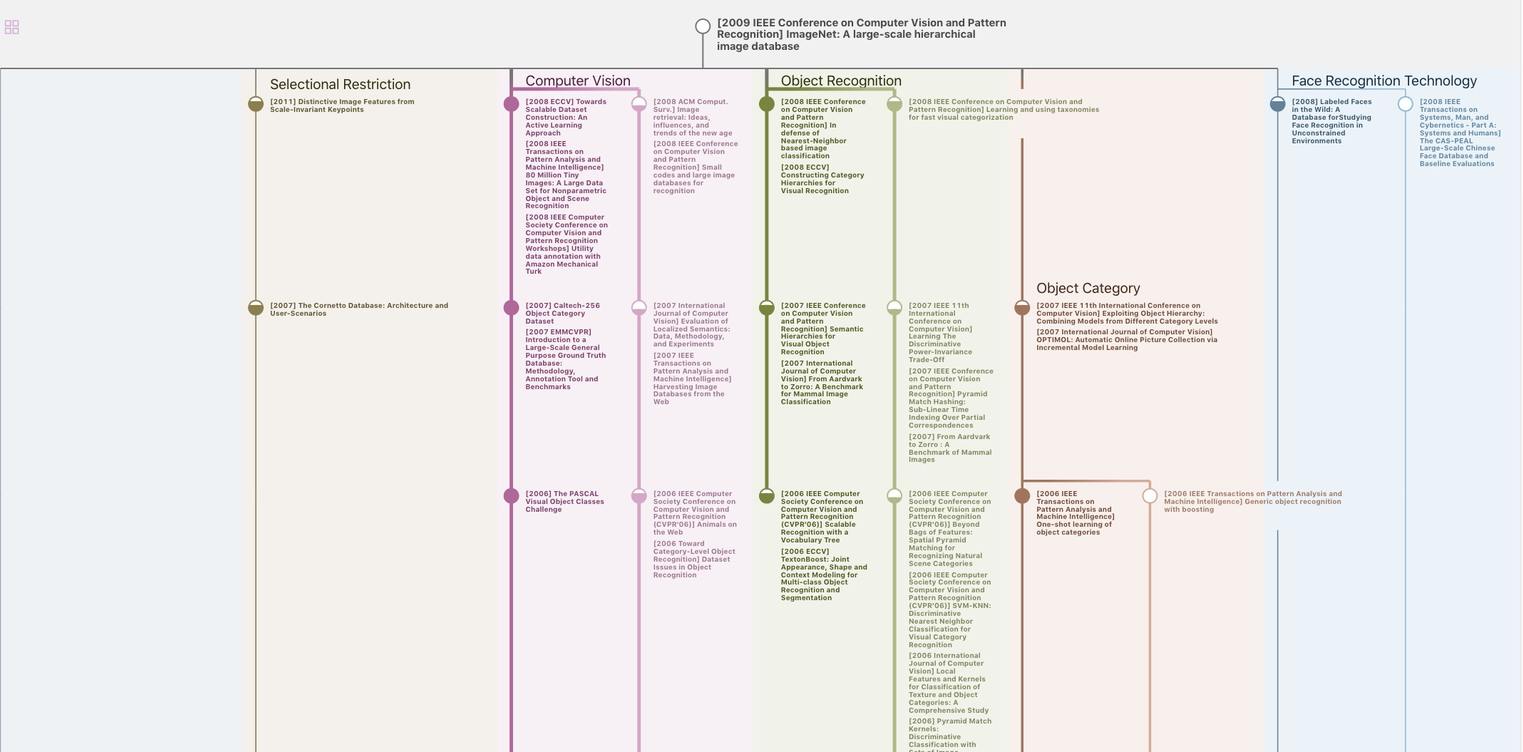
生成溯源树,研究论文发展脉络
Chat Paper
正在生成论文摘要