Constrained Active Learning for Anchor Link Prediction Across Multiple Heterogeneous Social Networks.
SENSORS(2017)
Abstract
Nowadays, people are usually involved in multiple heterogeneous social networks simultaneously. Discovering the anchor links between the accounts owned by the same users across different social networks is crucial for many important inter-network applications, e.g., cross-network link transfer and cross-network recommendation. Many different supervised models have been proposed to predict anchor links so far, but they are effective only when the labeled anchor links are abundant. However, in real scenarios, such a requirement can hardly be met and most anchor links are unlabeled, since manually labeling the inter-network anchor links is quite costly and tedious. To overcome such a problem and utilize the numerous unlabeled anchor links in model building, in this paper, we introduce the active learning based anchor link prediction problem. Different from the traditional active learning problems, due to the one-to-one constraint on anchor links, if an unlabeled anchor link a - (u, v) is identified as positive (i.e., existing), all the other unlabeled anchor links incident to account u or account v will be negative (i.e., non-existing) automatically. Viewed in such a perspective, asking for the labels of potential positive anchor links in the unlabeled set will be rewarding in the active anchor link prediction problem. Various novel anchor link information gain measures are defined in this paper, based on which several constraint active anchor link prediction methods are introduced. Extensive experiments have been done on real-world social networkdatasets to compare the performance of these methods with state-of-art anchor link prediction methods. The experimental results show that the proposed Mean-entropy-based Constrained Active Learning (MC) method can outperform other methods with significant advantages.
MoreTranslated text
Key words
multiple heterogeneous social networks,anchor link prediction,Constrained Active Learning
AI Read Science
Must-Reading Tree
Example
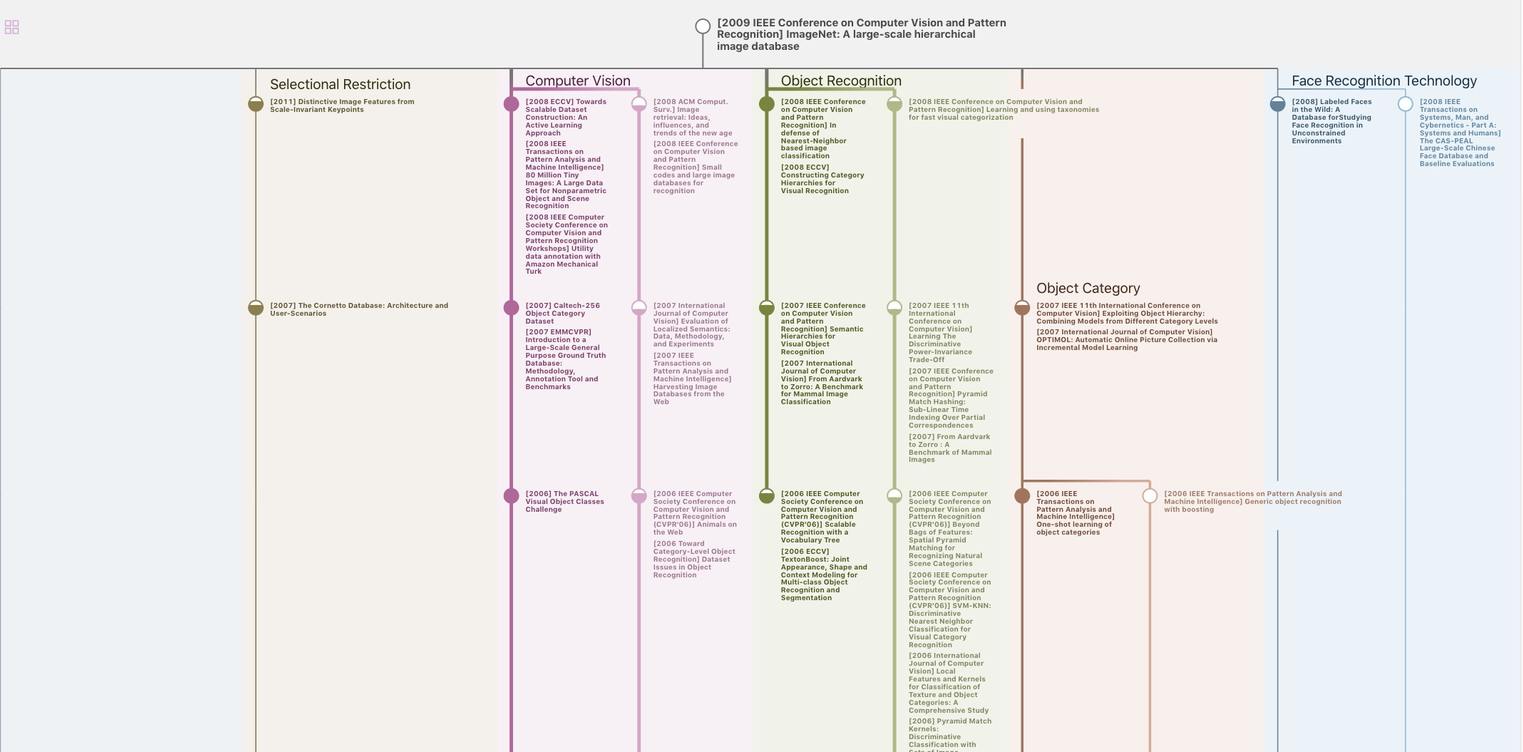
Generate MRT to find the research sequence of this paper
Chat Paper
Summary is being generated by the instructions you defined