Accelerating Depthwise Separable Convolutions on Ultra-Low-Power Devices
arxiv(2024)
摘要
Depthwise separable convolutions are a fundamental component in efficient
Deep Neural Networks, as they reduce the number of parameters and operations
compared to traditional convolutions while maintaining comparable accuracy.
However, their low data reuse opportunities make deploying them notoriously
difficult. In this work, we perform an extensive exploration of alternatives to
fuse the depthwise and pointwise kernels that constitute the separable
convolutional block. Our approach aims to minimize time-consuming memory
transfers by combining different data layouts. When targeting a commercial
ultra-low-power device with a three-level memory hierarchy, the GreenWaves GAP8
SoC, we reduce the latency of end-to-end network execution by up to 11.40
Furthermore, our kernels reduce activation data movements between L2 and L1
memories by up to 52.97
更多查看译文
AI 理解论文
溯源树
样例
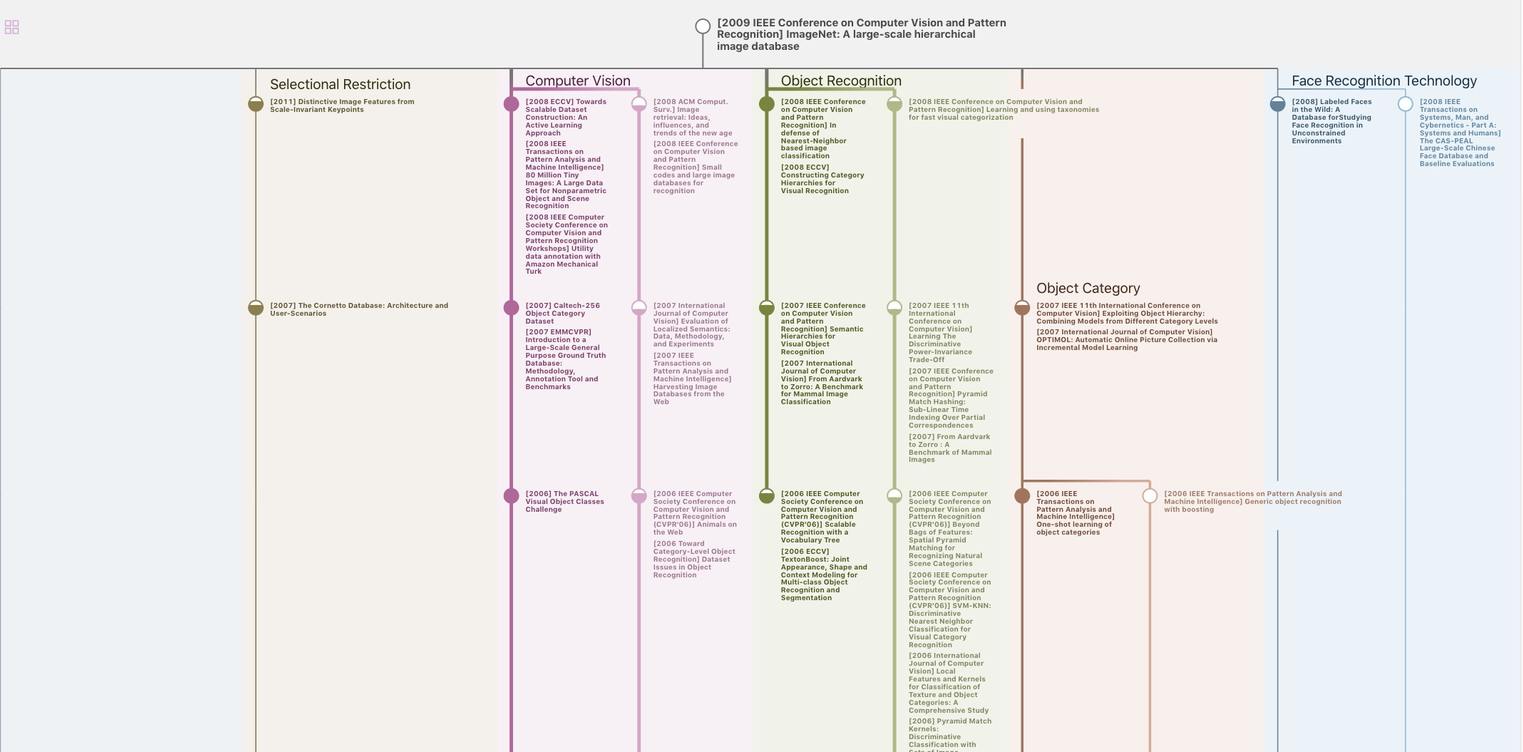
生成溯源树,研究论文发展脉络
Chat Paper
正在生成论文摘要