Faster Gated Recurrent Units Via Conditional Computation
2016 15th IEEE International Conference on Machine Learning and Applications (ICMLA)(2016)
摘要
In this work, we apply the idea of conditional computation to the gated recurrent unit (GRU), a type of recurrent activation function. With slight modifications to the GRU, the number of floating point operations required to calculate the feed-forward pass through the network may be significantly reduced. This allows for more rapid computation, enabling a trade-off between model accuracy and model speed. Such a tradeoff may be useful in a scenario where real-time performance is required, allowing for powerful recurrent models to be deployed on compute-limited devices.
更多查看译文
关键词
neural networks,conditional computation
AI 理解论文
溯源树
样例
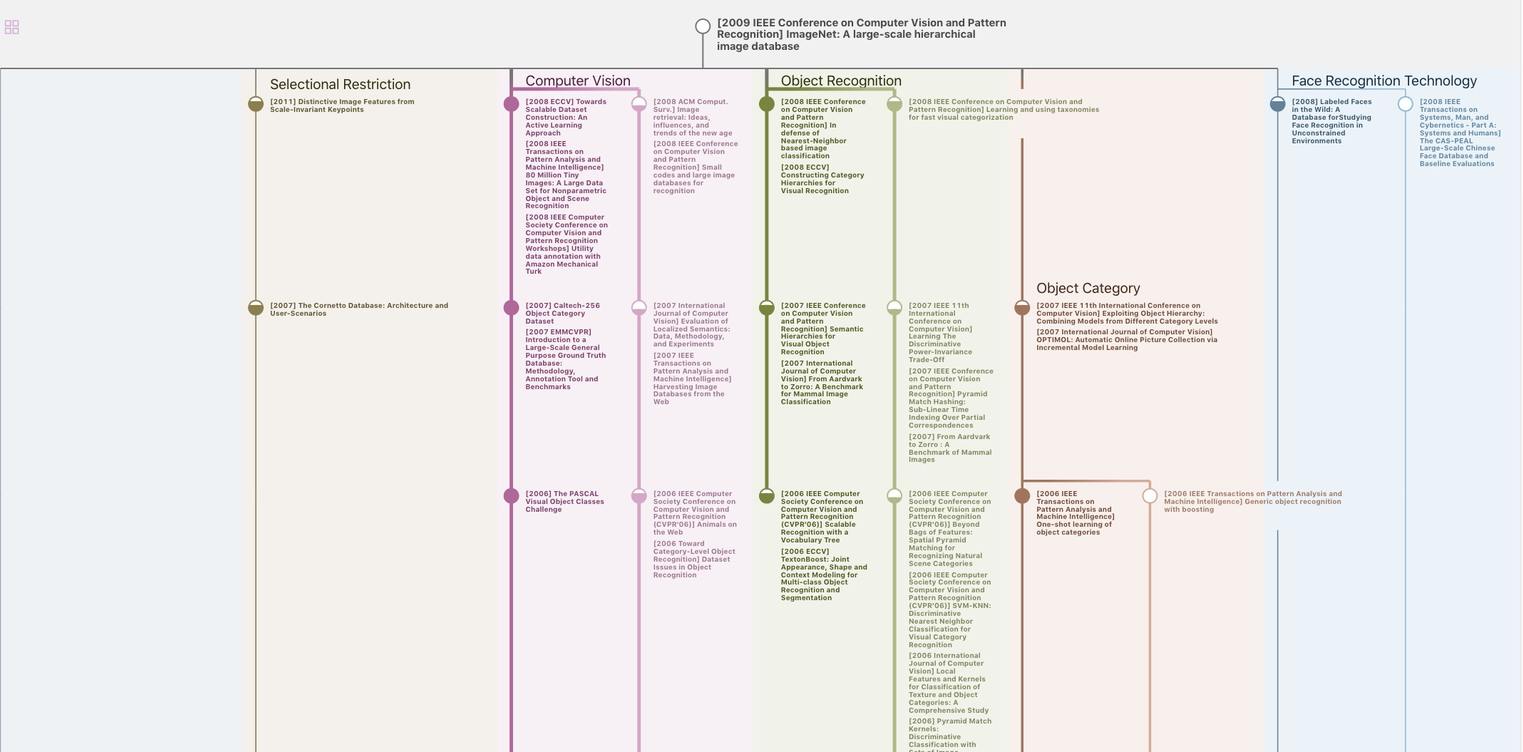
生成溯源树,研究论文发展脉络
Chat Paper
正在生成论文摘要