Lifelong Learning For Disturbance Rejection On Mobile Robots
2016 IEEE/RSJ International Conference on Intelligent Robots and Systems (IROS)(2016)
摘要
No two robots are exactly the same-even for a given model of robot, different units will require slightly different controllers. Furthermore, because robots change and degrade over time, a controller will need to change over time to remain optimal. This paper leverages lifelong learning in order to learn controllers for different robots. In particular, we show that by learning a set of control policies over robots with different (unknown) motion models, we can quickly adapt to changes in the robot, or learn a controller for a new robot with a unique set of disturbances. Furthermore, the approach is completely model-free, allowing us to apply this method to robots that have not, or cannot, be fully modeled.
更多查看译文
关键词
motion models,control policies,mobile robots,disturbance rejection,lifelong learning
AI 理解论文
溯源树
样例
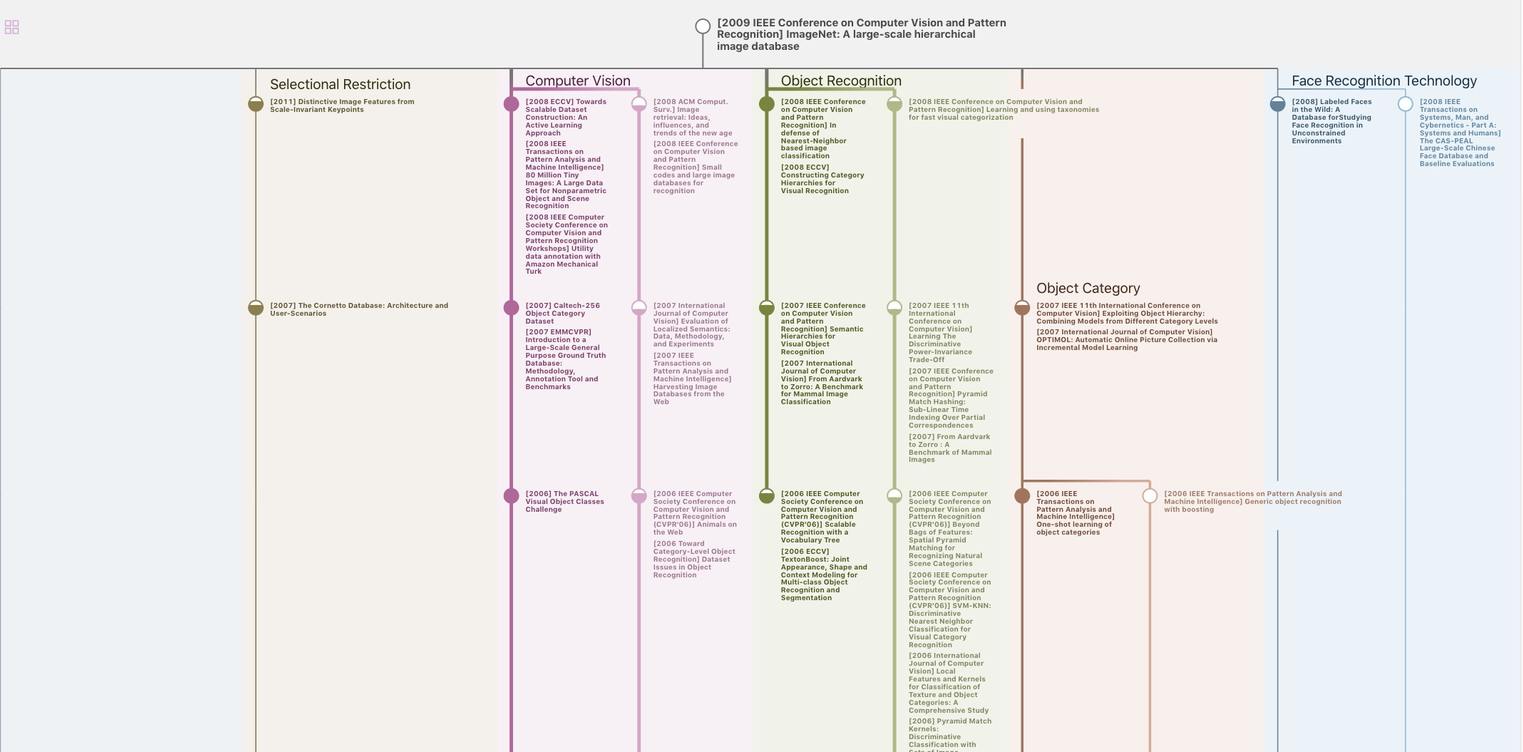
生成溯源树,研究论文发展脉络
Chat Paper
正在生成论文摘要