Sampled Differential Dynamic Programming
2016 IEEE/RSJ International Conference on Intelligent Robots and Systems (IROS)(2016)
摘要
We present SaDDP, a sampled version of the widely used differential dynamic programming (DDP) control algorithm. We contribute through establishing a novel connection between two major branches of robotics control research, that is, gradient-based methods such as DDP, and Monte Carlo methods such as path integral control (PI) that utilize random simulated trajectory rollouts. One of our key observations is that the Taylor-expansion, central to DDP, can be reformulated in terms of second-order statistics computed of the sampled trajectories. SaDDP makes few assumptions about the controlled system and works with black-box dynamics simulations with non-smooth contacts. Our simulation results show that the method outperforms PI and CMA-ES in both a simple linear-quadratic problem, and a multilink arm reaching task with obstacles.
更多查看译文
关键词
sampled differential dynamic programming,SaDDP,DDP control algorithm,robotics control research,gradient-based methods,Monte Carlo methods,random simulated trajectory rollouts,Taylor-expansion,black-box dynamics simulations,nonsmooth contacts,multilink arm,random location obstacles,robot arm
AI 理解论文
溯源树
样例
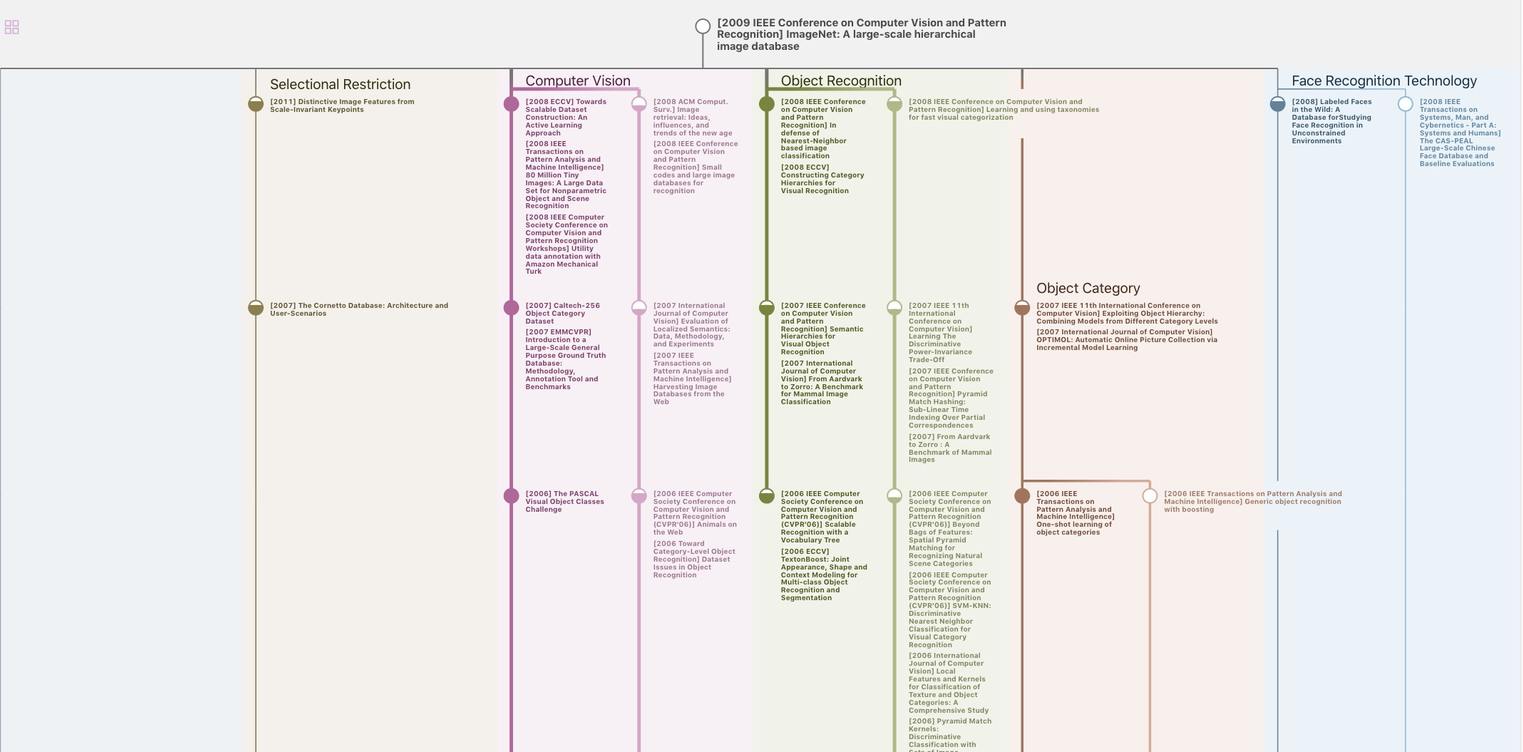
生成溯源树,研究论文发展脉络
Chat Paper
正在生成论文摘要