Reliable Attribute Selection Based on Random Forest (RASER).
INTELLIGENT SYSTEMS DESIGN AND APPLICATIONS (ISDA 2016)(2017)
摘要
Feature selection has become one of the most active research areas in the field of data mining. It allows removing redundant and irrelevant data sets of large size. Furthermore, there are several methods in the literature for selecting attributes. In this article, a new multi-objective method is proposed to select relevant and non-redundant features. Our proposed feature selection method is divided into three stages: The first step computes the feature relevance value based on random forests. The second step, computes the dissimilarity matrix representing the dependence between the features of our training datasets, and transform it into a complete graph whose nodes represent features and edges represent the values of dissimilarities between them. The last step is for the optimization in which a multi-objective optimization algorithm is applied. The proposed method is applied on many datasets to find the most relevant and non-redundant features and the performance of the proposed method is compared with that of the popular MBEGA, mRMR (MIQ) and mRMR (MID).
更多查看译文
关键词
Feature selection,Feature relevance,Feature redundance,Multi-objective optimization,Random forest
AI 理解论文
溯源树
样例
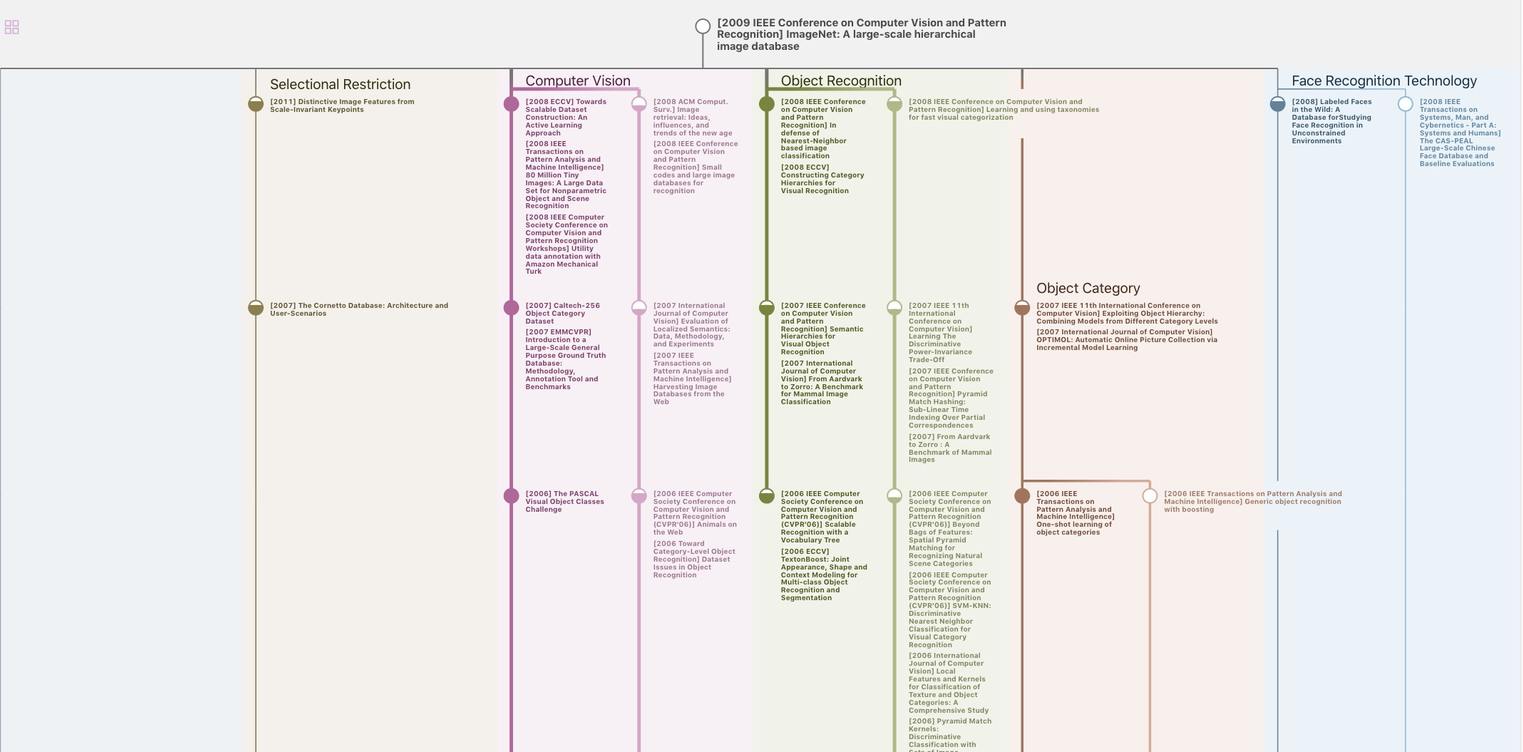
生成溯源树,研究论文发展脉络
Chat Paper
正在生成论文摘要