Model-Guided Segmentation Of Liver In Ct And Pet-Ct Images Of Child Patients Based On Statistical Region Merging
IEEE Conference Proceedings(2016)
Abstract
The paper introduces a novel model-guided method for liver segmentation in CT and PET-CT images. Using a model liver volume as a template and a liver shape annotated in one of the patient slices, it automatically segments the whole liver volume in the patient dataset. The method is based on non-deformable registration of the model volume to the patient data and combination of components pre-segmented by statistical region merging in each patient slice to maximise the overlap with the registered model shape. It does not require construction of probabilistic atlases, large training sets, or contrast enhancement of the portal venous phase. Its performance was tested on one CT and two PET-CT child patient scans, used alternately as patient data and, annotated by an expert, as a liver model. Additionally, subsampled and denoised data were used for testing, resulting in 21 experiments. The average accuracy measured as the Dice index between the computed volume and the expert-delineated one was 84.2 +/- 4.7 (as a percentage), which demonstrates robustness of the method to high variability in liver shape of child patients. The algorithm was developed primarily for the purpose of building voxel models of human anatomy for radiation dose calculation. The framework could be extended for segmentation of other organs and tissues necessary for construction of anatomy models.
MoreTranslated text
Key words
model-guided segmentation,PET-CT images,child patients,statistical region merging,liver segmentation,liver shape,patient slices,nondeformable registration,probabilistic atlases,contrast enhancement,organs,tissues
AI Read Science
Must-Reading Tree
Example
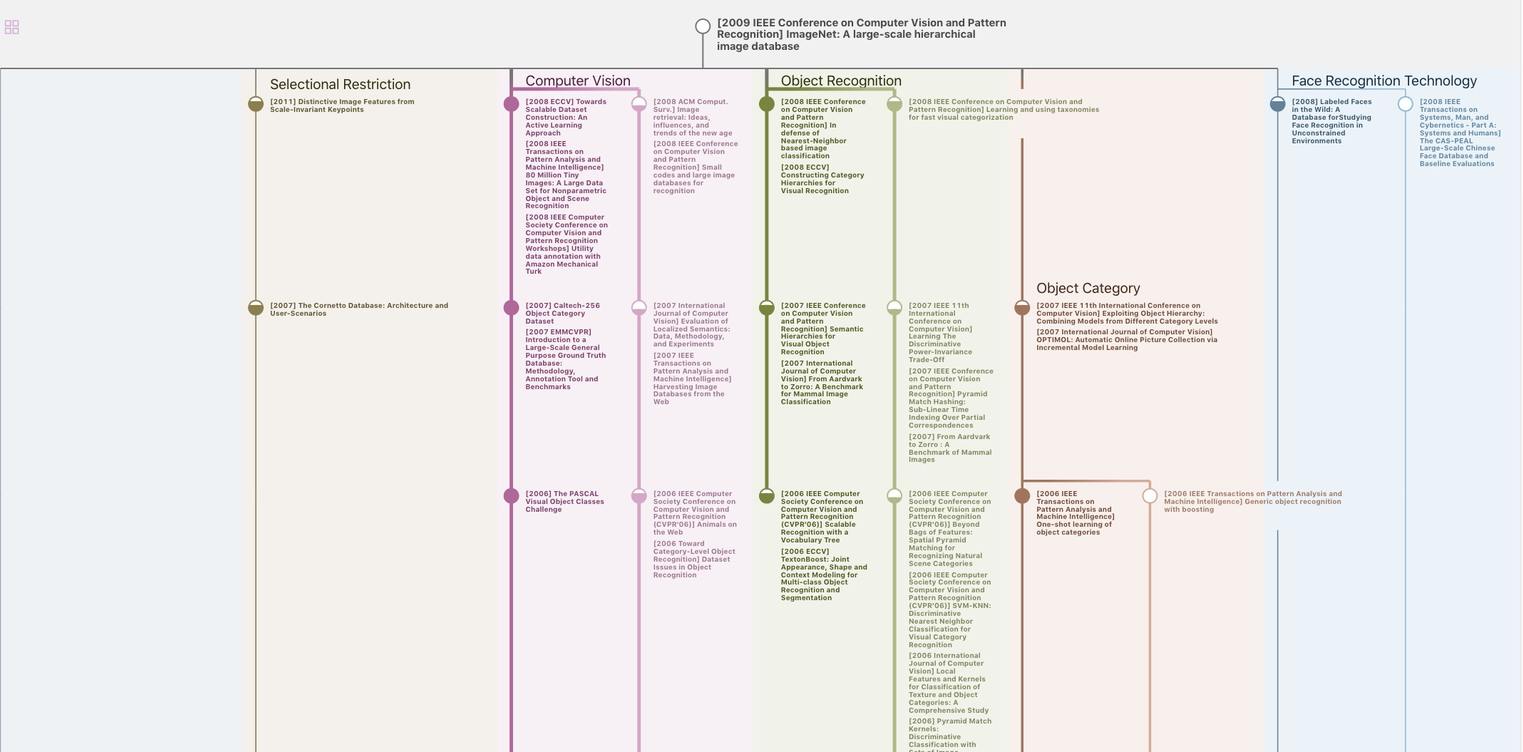
Generate MRT to find the research sequence of this paper
Chat Paper
Summary is being generated by the instructions you defined